Unit – 6
Small Samples
When you test a single mean, you’re comparing the mean value to some other hypothesized value. Which test you run depends on if you know the population standard deviation (σ) or not.
Known population standard deviation
If you know the value for σ, then the population mean has a normal distribution: use a one sample z-test. The z-test uses a formula to find a z-score, which you compare against a critical value found in a z-table. The formula is:
A one sample test of means compares the mean of a sample to a pre-specified value and tests for a deviation from that value. For example we might know that the average birth weight for white babies in the US is 3,410 grams and wish to compare the average birth weight of a sample of black babies to this value.
Assumptions
- Independent observations.
- The population from which the data is sampled is normally distributed.
Hypothesis:



Where μ0 is a pre-specified value (in our case this would be 3,410 grams).
Test Statistic
- First calculate
, the sample mean.
- We choose an α = 0.05 significance level
- If the standard deviation is known:

Using the significance level of 0.05, we reject the null hypothesis if z is greater than 1.96 or less than -1.96.
- If the standard deviation is unknown:

Using the significance level of 0.05, we reject the null hypothesis if |t| is greater than the critical value from a t-distribution with df = n-1.
Note: The shaded area is referred to as the critical region or rejection region.
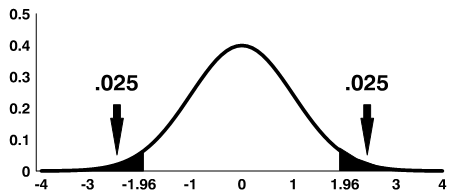
We can also calculate a 95% confidence interval around the mean. The general form for a confidence interval around the mean, if σ is unknown, is

For a two-sided 95% confidence interval, use the table of the t-distribution (found at the end of the section) to select the appropriate critical value of t for the two-sided α=0.05.
Example: one sample t-test
Recall the data used in module 3 in the data file "dixonmassey."
Data dixonmassey;
Input Obs chol52 chol62 age cor dchol agelt50 $;
Datalines;
1 | 240 | 209 | 35 | 0 | -31 | y |
2 | 243 | 209 | 64 | 1 | -34 | n |
3 | 250 | 173 | 61 | 0 | -77 | n |
4 | 254 | 165 | 44 | 0 | -89 | y |
5 | 264 | 239 | 30 | 0 | -25 | y |
6 | 279 | 270 | 41 | 0 | -9 | y |
7 | 284 | 274 | 31 | 0 | -10 | y |
8 | 285 | 254 | 48 | 1 | -31 | y |
9 | 290 | 223 | 35 | 0 | -67 | y |
10 | 298 | 209 | 44 | 0 | -89 | y |
11 | 302 | 219 | 51 | 1 | -83 | n |
12 | 310 | 281 | 52 | 0 | -29 | n |
13 | 312 | 251 | 37 | 1 | -61 | y |
14 | 315 | 208 | 61 | 1 | -107 | n |
15 | 322 | 227 | 44 | 1 | -95 | y |
16 | 337 | 269 | 52 | 0 | -68 | n |
17 | 348 | 299 | 31 | 0 | -49 | y |
18 | 384 | 238 | 58 | 0 | =146 | n |
19 | 386 | 285 | 33 | 0 | -101 | y |
20 | 520 | 325 | 40 | 1 | -195 | y |
;
Many doctors recommend having a total cholesterol level below 200 mg/dl. We will test to see if the 1952 population from which the Dixon and Massey sample was gathered is statistically different, on average, from this recommended level.
- H0: μ = 200 vs. H1: μ ≠ 200
- α=0.05
- Our sample of n=20 has
= 311.15 and s = 64.3929.
- Df = 19, so reject H0 if |t| > 2.093
Calculate:

|t| > 2.093 so we reject H0
The 95% confidence limits around the mean are
311.15 ± (2.093)(64.3929/√20)
311.15 ± 30.14
(281.01, 341.29)
One Sample t-test Using SAS:



Proc ttest data=name h0=μ0 alpha=α;
Var var;
Run;
SAS uses the stated α for the level of confidence (for example, α=0.05 will result in 95% confidence limits). For the hypothesis test, however, it does not compute critical values associated with the given α, and compare the t-statistic to the critical value. Rather, SAS will provide the p-value, the probability that T is more extreme than observed t. The decision rule, "reject if |t| > critical value associated with α" is equivalent to "reject if p < α."
SAS will provide the p value, the probability that T is more extreme than observed t. The decision rule, “reject if |t| critical value associated with
” is equivalent to “reject if p
a.”
Example:
Proc ttest data=dixonmassey h0=200 alpha=0.05;
Var chol52;
Title 'One Sample t-test with proc ttest';
Title2 'Testing if the sample of cholesterol levels in 1952 is statistically different from 200' ;
Run;
One sample t-test with proc ttest
‘Testing if the sample of cholesterol level in 1952 is statistically different from
The T TEST Procedure
Variable : chol52
N | Mean | Std Dev | Std Err | Minimum | Maximum |
20 | 311.2 | 64.3929 | 14.3987 | 240.0 | 520.0 |
Mean | 95% CL Mean | Std Dev | 95% CL | Std Dev |
311.2 | 281.0 | 64.3929 | 48.9702 | 94.0505 |
DF | T Value | Pr> |t| |
19 | 7.72 | <.0001 |
As in our hand calculations, t = 7.72, and we reject H0 (because p<0.0001 which is < 0.05, our selected α level).
The mean cholesterol in 1952 was 311.2, with 95% confidence limits (281.0, 341.3).
Testing the meaning of the correlation coefficient.
The relationship coefficient, r, tells us about the strength and direction of the linear relationship between X1 and X2.
Sample data is used to calculate r, the correlation coefficient for the sample. If we had data for the entire population, we could find the correlation coefficient for the population.
But since we only have sample data, we cannot calculate the population correlation coefficient. The sample correlation coefficient, r, is our estimate of the correlation coefficient for the unknown population.
• ρ = population correlation coefficient (unknown)
• r = sample relationship coefficient (known; calculated from sample data)
The hypothesis test allows us to decide if the value of the population correlation coefficient ρ is "close to zero" or "significantly different from zero". We decide on the basis of the correlation coefficient of sample r and the size of sample n.
If the test arranges that the relationship coefficient is meaningfully different from zero, we say that the relationship coefficient is "significant".
• Conclusion: there is sufficient evidence to conclude that there is a significant linear relationship between X1 and X2 because the correlation coefficient is significantly different from zero.
• Meaning of the conclusion: there is a significant linear relationship X1 and X2. If the test concludes that the correlation coefficient is not significantly different from zero (it is close to zero), we say that the correlation coefficient is not "significant".
Take the hypothesis test
• Null hypothesis: H0: ρ = 0
• Alternative hypothesis: Ha: ρ≠ 0
What do hypotheses mean in words?
• Hypothesis H0: the population correlation coefficient is NOT meaningfullydissimilar from zero. There is NO significant linear relationship (correlation) between X1 and X2 in the population.
• Alternative hypothesis Ha: the population correlation coefficient is significantly different from zero. Here is a significant linear relationship (correlation) amid X1 and X2 in the population.
Drawing a conclusion There are two methods of making a hypothetical decision. The test statistic to test this hypothesis is:
When the second formula is an equivalent form of the test statistic, n is the sample size and the degrees of freedom are n-2. This is a t-statistic and works in the same way as other t-tests.
Calculate the value t and compare it to the critical value in table t at the appropriate degrees of freedom and the level of confidence you want to maintain. If the calculated value is in the queue, then you cannot accept the null hypothesis that there is no linear relationship between these two independent random variables.
If the calculated t value is NOT in the queue, it is not possible to reject the null hypothesis that there is no linear relationship between the two variables.
A quick way to test correlations is the relationship between sample size and correlation. Me:
Then this implies that the correlation between the two variables demonstrates the existence of a linear relationship and is statistically significant at approximately the significance level of 0.05.
As the formula indicates, there is an inverse relationship between the sample size and the required correlation for the meaning of a linear relationship.
With only 10 observations, the required correlation for significance is 0.6325, for 30 observations the required correlation for significance drops to 0.3651 and for 100 observations the required level is only 0.2000.
Correlations can be useful for visualizing data, but are not used appropriately to "explain" a relationship between two variables. Perhaps a single statistic is not used more inadequately than the correlation coefficient.
Quoting correlations between health conditions and anything from place of residence to eye color has the effect of implying a cause and effect relationship. This simply cannot be accomplished with a correlation coefficient.
The correlation coefficient is obviously innocent of this misinterpretation. The analyst has a duty to use a statistic designed to test cause and effect relationships and report those results only if he intends to submit such a request.
The problem is that passing this stricter test is difficult, so lazy and / or unscrupulous "investigators" turn to correlations when they cannot legitimately support their case.
Define a t-test of a regression coefficient and provide a unique example of its use. Definition: A t-test is obtained by dividing a regression coefficient by its standard error and then comparing the result with critical values for students with df error.
Provides a test for the claim that when all other variables were included in the relevant regression model. Example: Suppose that 4 variables are suspected to influence some response. Suppose the assembly results include:
Variable | Regression coefficient | Standard error of regular coefficient |
.5 | 1 | -3 |
.4 | 2 | +2 |
.02 | 3 | +1 |
.6 | 4 | -.5 |
- t calculated for variables 1, 2 and 3 would be 5 or greater in absolute value while for variable 4 it would be less than 1. For most levels of significance, the hypothesis would be rejected. However, note that this is the case where, and have been included in the regression. For most levels of significance, the hypothesis would have continued (held) for the case where, and are in regression. Often this pattern of results will involve calculating another regression involving only and examining the proportions produced for that case.
- The correlation between the scores on a neuroticism test and the scores on an anxiety test is high and positive; Thus
- To. Anxiety causes neuroticism
- Yes. Those who score low on one test tend to score high on the other.
- C. Those who score small on one test tend to score low on the other.
- Re. You cannot make a meaningful prediction from one test to another.
- C. Those who score small on one test tend to score low on the additional.
- Testing the meaning of the correlation coefficient.
- LEARNING OUTCOMES
- • Calculate and interpret the correlation coefficient.
- The correlation coefficient, r, says us about the asset and direction of the linear relationship amid x and y. However, the reliability of the linear model also depends on the number of data points observed in the sample. We have to examine together the value of the correlation coefficient r and the sample size n.
- We done a hypothesis test on the "significance of the correlation coefficient" to choose whether the linear relationship in the sample data is strong sufficient to be used to model the connection in the population.
- Sample data is used to calculate r, the correlation coefficient for the sample. If we had data for the entire population, we could find the correlation coefficient for the population. But since we only have sample data, we cannot calculate the population correlation coefficient. The sample correlation coefficient, r, is our estimate of the correlation coefficient for the unknown population.
- • The representation for the population correlation coefficient is ρ, the Greek letter "rho".
- ρ = population correlation coefficient (unknown)
- r = sample correlation coefficient (known; calculated from sample data)
- The hypothesis test allows us to decide if the value of the population correlation coefficient
- ρ is "near to zero" or "significantly dissimilar from zero". We decide on the basis of the correlation coefficient of sample r and the size of sample n.
- If the test determines that the correlation coefficient is meaningfullydissimilar from zero, we say that the association coefficient is "significant".
- Assumption: there is sufficient indication to accomplish that there is aimportant linear relationship between x and y, since the association coefficient is significantly dissimilar from zero. What does the conclusion mean: There is a significant linear relationship between x and y. We can practice the reversion line to model the linear relationship amid x and y in the population.
- If the test achieves that the correlation coefficient is not meaningfullydiverse from zero (it is close to zero), we say that the correlation coefficient is not "significant".
- Assumption: “There is insufficient indication to accomplish that there is a significant linear relationship amid
- x and y because the correlation coefficient is not meaningfullydissimilar from zero. "What the conclusion means: There is no important linear association between x and y. So, we CANNOT use the reversion line to model a linear relationship amid x and y in the population.
- Note
- • If r is significant and the scatter diagram shows a linear trend, the line can be used to predict the value of y for the values of x that fall within the domain of the observed values of x.
- • If r is not significant OR if the scatter diagram does not show a linear trend, the line should not be used for forecasting.
- • If r is significant and if the scatter diagram shows a linear trend, the line may NOT be appropriate or reliable to predict OUT of the domain of the x values observed in the data.
- Take the hypothesis test
- Null hypothesis: H0: ρ = 0
- Alternative hypothesis: Ha: ρ≠ 0
- What do hypotheses mean in words?
- Hypothesis H0: the population relationship coefficient is NOT meaningfullydissimilar from zero. There is NO significant linear relationship (correlation) between x and y in the population.
- Alternative hypothesis Ha: the population correlation coefficient is significantly different from zero. There is aimportant linear relationship (correlation) between x and y in the population.
- Get a conclusion
- There are two methods of making the decision. The two methods are equivalent and give the same result.
- Method 1: using the p value
- Method 2: use of a table of critical values.
- In this chapter of this textbook, we will continuously use a significance level of 5%, α = 0.05
Note
- Using the p-value method, you can choose any appropriate level of significance desired; It is not limited to the use of α = 0.05. But the critical value table provided in this textbook assumes that we are using a significance level of 5%, α = 0.05. (If we wanted to use a significance level other than 5% with the critical value method, we would need several tables of critical values that are not provided in this manual).
- Method 1: use a p-value to make a decision
- To calculate the p-value using LinRegTTEST:
- • On the LinRegTTEST input screen, at the line prompt for β or ρ, highlight "≠ 0"
- The output screen shows the p value on the line that says "p =".
- (Most statistical software can calculate the p-value).
- If the p value is fewer than the meaning level (α = 0.05)
- Decision: reject the null hypothesis.
- Assumption: "There is sufficient indication to accomplish that there is aimportant linear relationship amid x and y since the correlation coefficient is meaningfullydissimilar from zero."
- If the p value is NOT less than the meaning level (α = 0.05)
- Decision: DO NOT reject the null hypothesis.
- Conclusion: "There is insufficient evidence to conclude that there is a significant linear relationship between x and y because the correlation coefficient is NOT significantly different from zero."
- Calculation Notes:
- Use technology to calculate the p-value. The calculations for calculating test statistics and p-value are described below:
- The p-value is considered using a t-distribution with n - 2 degrees of freedom.
- The formula for the test statistic is t = r√n - 2√1 - r2t = rn - 21 - r2. The test statistic value, t, is displayed on the computer or calculator output along with the p-value. The t-test statistic has the same sign as the correlation coefficient r.
- The p-value is the combined area in both tails.
Chi-square test for independence
This lesson explains how to take a chi-square test for independence. The test is useful when you have two definite variables from a single population.
It is used to determine if there is a significant association between the two variables.
For example, in an electoral poll, voters could be classified by gender (male or female) and voting preference (democratic, republican, or independent).
We could use a chi-square test for independence to determine if gender is linked to voting preference. The example problem at the end of the lesson considers this example.
When to use the Chi-square test for independence
The testing procedure described in this lesson is appropriate when the following conditions are true:
The sample method is a simple random sampling.
The variables under consideration are categorical.
If the sample data is showed in a likelihood table, the predictable frequency total for each cell in the table is at least 5.
This approach involves four phases: (1) declaring the hypotheses, (2) formulating an analysis plan, (3) analyzing the sample data, and (4) interpreting the results.
State hypotheses
Suppose that variable A has levels r and that variable B has levels c. The null hypothesis establishes that knowing the level of variable A does not help to predict the level of variable B.
That is, the variables are independent.
Ho: variable A and variable B are independent.
Ha: variables A and B are not independent.
The another hypothesis is that meaningful the level of flexible A can help you expect the level of variable B.
Note: support for the another hypothesis proposes that the variables are connected; but the connection is not essentiallyconnecting, in the sense that one variable "causes" the other.
Formulate an analysis plan.
The analysis plan describes how to use the sample data to accept or reject the null hypothesis. The plan must specify the following elements.
Level of significance. Researchers often choose significance levels of 0.01, 0.05, or 0.10; but you can use any value between 0 and 1.
Test method. Use the chi-square test to determine independence to determine if there is a significant relationship between two categorical variables.
Analyze the sample data.
Using the sample data, find the degrees of freedom, the predictable frequencies, the test statistic, and the P value associated with the test statistic. The methoddefined in this unit is showed in the sample problematic at the end of this lesson.
Degrees of freedom. The degrees of freedom (DF) are equal to:
DF = (r - 1) * (c - 1)
Where r is the number of levels for one catabolic variable and c is the number of levels for the other categorical variable.
You predictable frequencies. The predictable frequency counts are considereddistinctly for each level of one definite variable at each level of the other categorical variable.
Calculate the predictable frequencies r * c, according to the following formula.
Er, c = (nr * nc) / n
Where Er, c is the predictable frequency count for level r of variable A and level c of variable B, nr is the total number of sample explanations at level r of variable A, nc is the total number of sample explanations at level c of variable B, en is the total sample size.
Statistical test. The test statistic is a chi-square (Χ2) random variable defined by the following equation.
Χ2 = Σ[ (Or,c - Er,c)2 / Er,c ]
Where O, c is the observed frequency count at level r of variable A and level c of variable B, and Er, c is the predicted frequency count at level r of adjustable A and level c of adjustable B.
p-value The P value is the probability of detecting a sample figure as exciting as the test statistic.
Meanwhile the test measurement is a chi-square, use the Chi-square supply calculator to measure the probability related with the test statistic. Use the degrees of freedom considered above.
Interpretation of results
If the sample results are unlikely, given the null hypothesis, the researcher rejects the null hypothesis.
Typically, this involves comparing the P value with the significance level and rejecting the null hypothesis when the P value is less than the significance level.
Test your understanding:
A public opinion poll analyzed a simple random sample of 1,000 voters. Respondents were classified by gender (male or female) and by voting preference (Republican, Democratic, or Independent).
The effects are shown in the possibility table below.
| Voting Preferences | Row total | ||
Rep | Dem | Ind | ||
Male | 200 | 150 | 50 | 400 |
Female | 250 | 300 | 50 | 600 |
Column total | 450 | 450 | 100 | 1000 |
- Is there a gender gap? Do men's voting preferences differ significantly from women's preferences? Use a significance level of 0.05. Solution The solution to this problem involves four steps: (1) state the hypotheses, (2) formulate an analysis plan, (3) analyze the sample data, and (4) interpret the results.
- We work through the following steps:
State the hypotheses. The first step is to affirm the null hypothesis and an alternative hypothesis. Ho: Gender and voting preferences are independent. Ha: Gender and voting preferences are not independent.
Formulate an analysis plan. For this analysis, the significance level is 0.05. Using sample data, we will perform a chi-square test for independence.
Analyze the sample data. By applying the chi-square test for independence to the sampling data, we calculated degrees of freedom, predictable frequency counts, and chi-square test statistics.
Based on the chi-square statistic and the degrees of freedom, we determine the value P..
DF = (r - 1) * (c - 1) = (2 - 1) * (3 - 1) = 2
Er,c = (nr * nc) / n
E1,1 = (400 * 450) / 1000 = 180000/1000 = 180
E1,2 = (400 * 450) / 1000 = 180000/1000 = 180
E1,3 = (400 * 100) / 1000 = 40000/1000 = 40
E2,1 = (600 * 450) / 1000 = 270000/1000 = 270
E2,2 = (600 * 450) / 1000 = 270000/1000 = 270
E2,3 = (600 * 100) / 1000 = 60000/1000 = 60
Χ2 = Σ [ (Or,c - Er,c)2 / Er,c ]
Χ2 = (200 - 180)2/180 + (150 - 180)2/180 + (50 - 40)2/40
+ (250 - 270)2/270 + (300 - 270)2/270 + (50 - 60)2/60
Χ2 = 400/180 + 900/180 + 100/40 + 400/270 + 900/270 + 100/60
Χ2 = 2.22 + 5.00 + 2.50 + 1.48 + 3.33 + 1.67 = 16.2
Where DF is the degree of freedom, r is the number of levels of gender, c is the number of levels of the voting preference, nr is the number of observations of level r of gender, nc is the number of observations of level c of voting preference, n is the number of observations in the sample, Er, c is the predicted frequency count when gender is level r and voting preference is level c, and O, c is the observed frequency count when gender is level r, voting preference is level c.
The P value is the probability that a chi-square statistic with 2 degrees of freedom is more extreme than 16.2.
We use the Chi-Square distribution calculator to find P (Χ2> 16.2) = 0.0003.
Interpret the results. Since the P value (0.0003) is lower than the significance level (0.05), we cannot accept the null hypothesis.
Therefore, we conclude that there is a relationship between gender and voting preference.
References
- E. Kreyszig, “Advanced Engineering Mathematics”, John Wiley & Sons, 2006.
- P. G. Hoel, S. C. Port And C. J. Stone, “Introduction To Probability Theory”, Universal Book Stall, 2003.
- S. Ross, “A First Course in Probability”, Pearson Education India, 2002.
- W. Feller, “An Introduction To Probability Theory and Its Applications”, Vol. 1, Wiley, 1968.
- N.P. Bali and M. Goyal, “A Text Book of Engineering Mathematics”, Laxmi Publications, 2010.
- B.S. Grewal, “Higher Engineering Mathematics”, Khanna Publishers, 2000.
- T. Veerarajan, “Engineering Mathematics”, Tata Mcgraw-Hill, New Delhi, 2010