Unit – 4
Data Mining Primitives, Primitives, Languages and System Architectures
A data mining query is defined in terms of the following primitives
Task-relevant data: This is the database portion to be investigated. For example, suppose that you are a manager of All Electronics in charge of sales in the United States and Canada. In particular, you would like to study the buying trends of customers in Canada. Rather than mining on the entire database. These are referred to as relevant attributes
The kinds of knowledge to be mined: This specifies the data mining functions to be performed, such as characterization, discrimination, association, classification, clustering, or evolution analysis. For instance, if studying the buying habits of customers in Canada, you may choose to mine associations between customer profiles and the items that these customers like to buy
Background knowledge: Users can specify background knowledge, or knowledge about the domain to be mined. This knowledge is useful for guiding the knowledge discovery process, and for evaluating the patterns found. There are several kinds of background knowledge.
Interestingness measures: These functions are used to separate uninteresting patterns from knowledge. They may be used to guide the mining process, or after discovery, to evaluate the discovered patterns. Different kinds of knowledge may have different interestingness measures.
Presentation and visualization of discovered patterns: This refers to the form in which discovered patterns are to be displayed. Users can choose from different forms for knowledge presentation, such as rules, tables, charts, graphs, decision trees, and cubes.
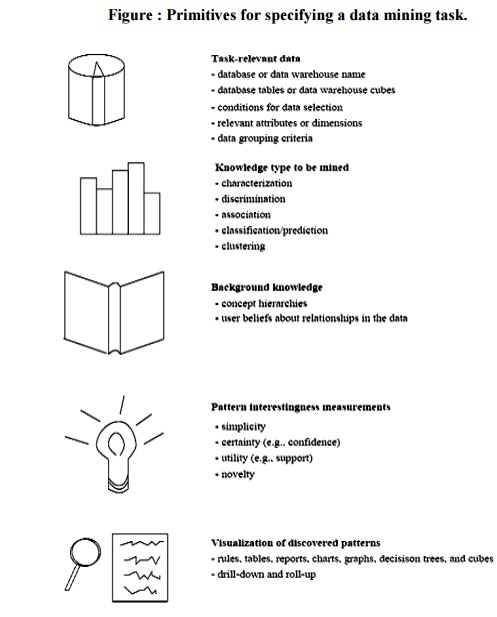
Text Mining is also known as Text Data Mining. The purpose is too unstructured information, extract meaningful numeric indices from the text. Thus, make the information contained in the text accessible to the various algorithms. Information can extracted to derive summaries contained in the documents. Hence, you can analyse words, clusters of words used in documents. In the most general terms, text mining will “turn text into numbers”. Such as predictive data mining projects, the application of unsupervised learning methods.
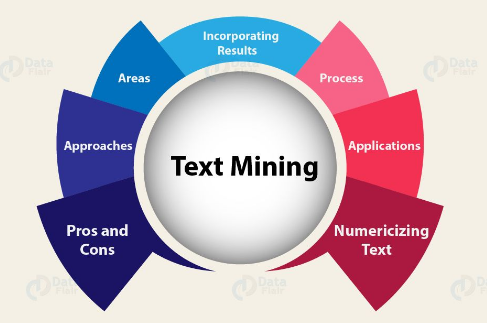
Areas of Text Mining in Data Mining
Following are the areas of text mining in Data Mining:
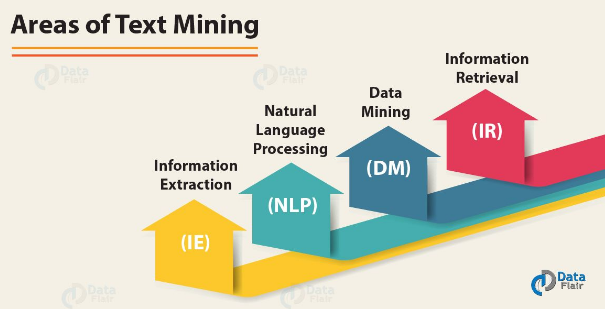
a. Information Retrieval (IR)
Information retrieval is regarded as an extension to document retrieval. That the documents that are returned are processed to condense. Thus document retrieval follow by a text summarization stage. That focuses on the query posed by the user. IR systems help in to narrow down the set of documents that are relevant to a particular problem. As text mining involves applying very complex algorithms to large document collections. Also, IR can speed up the analysis significantly by reducing the number of documents.
b. Data Mining (DM)
Data mining can loosely describe as looking for patterns in data. It can more characterize as the extraction of hidden from data. Data mining tools can predict behaviours and future trends. Also, it allows businesses to make positive, knowledge-based decisions. Data mining tools can answer business questions. Particularly that have traditionally been too time-consuming to resolve. They search databases for hidden and unknown patterns.
Follow this link to know about Data Mining Tools
c. Natural Language Processing (NLP)
NLP is one of the oldest and most challenging problems. It is the study of human language. So those computers can understand natural languages as humans do. NLP research pursues the vague question of how we understand the meaning of a sentence or a document. What are the indications we use to understand who did what to whom? The role of NLP in text mining is to deliver the system in the information extraction phase as an input.
d. Information Extraction (IE)
Information Extraction is the task of automatically extracting structured information from unstructured. In most of the cases, this activity includes processing human language texts by means of NLP.
Text Mining Process
A process of Text mining involves a series of activities to perform to mine the information. These activities are:
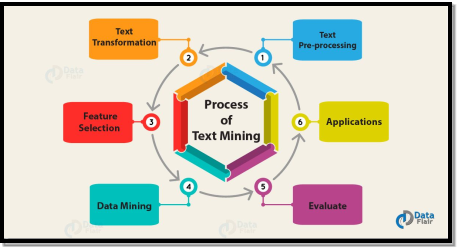
a. Text Pre-processing
It involves a series of steps as shown in below:
- Text Cleanup
Text Cleanup means removing any unnecessary or unwanted information. Such as remove ads from web pages, normalize text converted from binary formats.
- Tokenization
Tokenizing is simply achieved by splitting the text into white spaces.
- Part of Speech Tagging
Part-of-Speech (POS) tagging means word class assignment to each token. Its input is given by the tokenized text. Taggers have to cope with unknown words (OOV problem) and ambiguous word-tag mappings.
b. Text Transformation (Attribute Generation)
A text document is represented by the words it contains and their occurrences. Two main approaches to document representation are:
i. Bag of words
Ii. Vector Space
c. Feature Selection (Attribute Selection)
Feature selection also is known as variable selection. It is the process of selecting a subset of important features for use in model creation. Redundant features are the one which provides no extra information. Irrelevant features provide no useful or relevant information in any context.
d. Data Mining
At this point, the Text mining process merges with the traditional process. Classic Data Mining techniques are used in the structured database. Also, it resulted from the previous stages.
e. Evaluate
Evaluate the result, after evaluation, the result discard.
f. Applications
Text Mining applies in a variety of areas. Some of the most common areas are
- Web Mining
These days web contains a treasure of information about subjects. Such as persons, companies, organizations, products, etc. that may be of wide interest. Web Mining is an application of data mining techniques. That need to discover hidden and unknown patterns from the Web. Web mining is an activity of identifying term implied in a large document collection. It says C which denotes by a mapping i.e. C →p [10].
- Medical
Users exchange information with others about subjects of interest. Everyone wants to understand specific diseases, to inform about new therapies. Also, these expert forums also represent seismographs for medical. E-mails, e-consultations, and requests for medical advice. That is via the internet have been analyzed using quantitative or qualitative methods.
- Resume Filtering
Big enterprises and headhunters receive thousands of resumes from job applicants every day. Extracting information from resumes with high precision and recall is not easy. Automatically extracting this information can the first step in filtering resumes. Hence, automating the process of resume selection is an important task.
Approaches to Text Mining in Data Mining
Using well-tested methods and understanding the results of text mining. Once a data matrix has been computed from the input documents. And words found in those documents, various well-known analytic techniques. As it is used for further processing those data including methods for clustering.
“Black-box” approaches to text mining and extraction of concepts. There are text mining applications which offer “black-box” methods. That need to extract “deep meaning” from documents with little human effort. These text mining applications rely on proprietary algorithms.
Numericizing Text
Following are issues and considerations for Numericizing Text.
i. Large numbers of large documents
Examples of scenarios using large numbers of small were given earlier. But, if your intent is to extract “concepts” from only a few documents that are very large. Then analyses are less powerful because the “number of cases” in this case is very small. While the “number of variables” (extracted words) is very large.
ii. Excluding certain characters, short words, numbers, etc
Excluding numbers, certain characters can be done easily. But before the indexing of the input documents starts. You may also want to exclude “rare words,”. As defined as those that only occur in a small percentage of the processed documents.
iii. Include lists, exclude lists (stop-words)
This is useful when you want to search for particular words. Also, classifying the input documents based on the frequencies. Also, “stop-words,” i.e., terms that are to exclude from the indexing can define. Typically, a default list of English stop words includes “the”, “a”, “of”, “since,”. That is words that are used in the respective language very frequently. But communicate very little unique information about the contents of the document.
iv. Synonyms and phrases
Synonyms, such as “sick” or “ill”, or words that are used in particular phrases. Where they denote unique meaning and can combine for indexing.
For example-
“Microsoft Windows” might be such a phrase. That is a specific reference to the computer operating system. But has nothing to do with the common use of the term “Windows”. As it might, for example, use in descriptions of home improvement projects.
v. Stemming algorithms
An important pre-processing step before indexing of input documents. As it begins is the stemming of words. The term “stemming” refers to the reduction of words to their roots. So that, for example, different grammatical forms.
vi Support for different languages
Stemming, synonyms, the letters that are permitted in words. Also, are highly language dependent operations. Therefore, support for different languages is important.
Incorporating Text Mining Results
Incorporating Text Mining Results in Data Mining Projects, after significant words have been extracted from a set of input documents. And after singular value decomposition has been applied to extract salient semantic dimensions. Typically the next and most important step is to use the extracted information.
a. Graphics (visual data mining methods)
Depending on the purpose of the analyses, in some instances. We need extraction of semantic dimensions alone. As it can be a useful outcome if it clarifies the underlying structure.
b. Clustering and factoring
You can use cluster analysis methods to identify groups of documents. Also, to identify groups of similar input texts. This type of analysis also useful in the context of market research studies.
For example- of new car owners. You can also use Factor Analysis and Principal Components and Classification Analysis.
c. Predictive data mining
Another possibility is to use the raw as predictor variables in mining projects.
Text Mining Applications a. Analyzing open-ended survey responses
Unstructured text is very common. And may represent the majority of information available to a particular research.
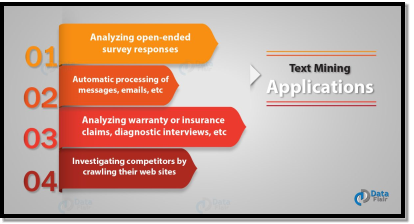
In survey research, it is not uncommon to include various open-ended questions. That is pertaining to the topic under investigation. The idea is to permit respondents to express their “views”. Also, opinions without constraining them to particular dimensions or a particular response format.
b. Automatic processing of messages, emails, etc
Another common application is to aid in the automatic classification of texts.
For example-
It is possible to “filter” out automatically most undesirable “junk email”. That is based on certain terms or words that are not likely to appear in legitimate messages. Although, instead identify undesirable electronic mail. In this manner, such messages can automatically discard. Such automatic systems for classifying electronic messages can also be useful in applications. That messages need to route to the most appropriate department. At the same time, the emails are screened for inappropriate or obscene messages. That are automatically returned to the sender with a request to remove the offending words or content.
c. Analyzing warranty or insurance claims, diagnostic interviews, etc
In some business domains, the majority of information is collected in open-ended.
For example-
Warranty claims or initial medical interviews can summarize in brief narratives. Increasingly, those notes are collected electronically. So those types of narratives are readily available for input. This information can then usefully exploit to, Likewise, in the medical field. Also, open-ended descriptions by patients of their own symptoms. That might yield useful clues for the actual medical diagnosis.
d. Investigating competitors by crawling their websites
Another type of application is to process the contents of Web pages in a particular domain.
For example-
You could go to a Web page, and begin “crawling” the links you find there to process all Web pages that are referenced. In this manner, you could derive a list of terms and documents available at that site. Hence determine the most important terms and features that are described.
Advantages & Disadvantages of Text Mining
Following are the pros and cons of Text Mining in Data Mining:
a. Advantages of Text Mining
Web mining essentially has many advantages. That make this technology attractive to corporations including the government agencies. This technology has enabled e-commerce to do personalized marketing. As it includes eventually results in higher trade volumes. The government agencies are using this technology to classify threats. The predicting capability can benefit the society by identifying criminal activities. The companies can establish a better customer relationship. Exactly by giving them exactly what they need. Companies can understand the needs of the customer better. Further, they can react to customer needs faster.
Disadvantages of Text Mining
Web mining the technology itself doesn’t create issues. Although, this technology when used on data of personal nature might cause concerns.
The most criticized ethical issue involving web mining is the invasion of privacy. Privacy is considered lost when information concerning an individual is obtained. The obtained data will analyze, and clustered to form profiles. Also, the data will make anonymous before clustering. So that no individual can link directly to a profile. But usually, the group profiles are used as if they are personal profiles. Thus these applications de-individualize the users by judging them by their mouse clicks.
De-individualization can define as a tendency of judging and treating people. Particularly, on the basis of group characteristics.
Another important concern is that the companies collecting the data. That is for a specific purpose might use the data for a totally different purpose. And this essentially violates the user’s interests. The growing trend of selling personal data as a commodity encourages website owners. That is to trade personal data obtained from their site. This trend has increased the amount of data being captured. Also, traded increasing the likeliness of one’s privacy being invaded.
The Data Mining Query Language (DMQL) was proposed by Han, Fu, Wang, et al. For the DBMiner data mining system. The Data Mining Query Language is actually based on the Structured Query Language (SQL). Data Mining Query Languages can be designed to support ad hoc and interactive data mining. This DMQL provides commands for specifying primitives. The DMQL can work with databases and data warehouses as well. DMQL can be used to define data mining tasks. Particularly we examine how to define data warehouses and data marts in DMQL.
Syntax for Task-Relevant Data Specification
Here is the syntax of DMQL for specifying task-relevant data −
Use database database_name
Or
Use data warehouse data_warehouse_name
In relevance to att_or_dim_list
From relation(s)/cube(s) [where condition]
Order by order_list
Group by grouping_list
Syntax for Specifying the Kind of Knowledge
Here we will discuss the syntax for Characterization, Discrimination, Association, Classification, and Prediction.
Characterization
The syntax for characterization is −
Mine characteristics [as pattern_name]
Analyze {measure(s) }
The analyze clause, specifies aggregate measures, such as count, sum, or count%.
For example −
Description describing customer purchasing habits.
Mine characteristics as customerPurchasing
Analyze count%
Discrimination
The syntax for Discrimination is −
Mine comparison [as {pattern_name]}
For {target_class } where {t arget_condition }
{versus {contrast_class_i }
Where {contrast_condition_i}}
Analyze {measure(s) }
For example, a user may define big spenders as customers who purchase items that cost $100 or more on an average; and budget spenders as customers who purchase items at less than $100 on an average. The mining of discriminant descriptions for customers from each of these categories can be specified in the DMQL as −
Mine comparison as purchaseGroups
For bigSpenders where avg(I.price) ≥$100
Versus budgetSpenders where avg(I.price)< $100
Analyze count
Association
The syntax for Association is−
Mine associations [ as {pattern_name} ]
{matching {metapattern} }
For Example −
Mine associations as buyingHabits
Matching P(X:customer,W) ^ Q(X,Y) ≥ buys(X,Z)
Where X is key of customer relation; P and Q are predicate variables; and W, Y, and Z are object variables.
Classification
The syntax for Classification is −
Mine classification [as pattern_name]
Analyze classifying_attribute_or_dimension
For example, to mine patterns, classifying customer credit rating where the classes are determined by the attribute credit_rating, and mine classification is determined as classifyCustomerCreditRating.
Analyze credit_rating
Prediction
The syntax for prediction is −
Mine prediction [as pattern_name]
Analyze prediction_attribute_or_dimension
{set {attribute_or_dimension_i= value_i}}
Syntax for Concept Hierarchy Specification
To specify concept hierarchies, use the following syntax −
Use hierarchy <hierarchy> for <attribute_or_dimension>
We use different syntaxes to define different types of hierarchies such as−
-schema hierarchies
Define hierarchy time_hierarchy on date as [date,month quarter,year]
-
Set-grouping hierarchies
Define hierarchy age_hierarchy for age on customer as
Level1: {young, middle_aged, senior} < level0: all
Level2: {20, ..., 39} < level1: young
Level3: {40, ..., 59} < level1: middle_aged
Level4: {60, ..., 89} < level1: senior
-operation-derived hierarchies
Define hierarchy age_hierarchy for age on customer as
{age_category(1), ..., age_category(5)}
:= cluster(default, age, 5) < all(age)
-rule-based hierarchies
Define hierarchy profit_margin_hierarchy on item as
Level_1: low_profit_margin < level_0: all
If (price - cost)< $50
Level_1: medium-profit_margin < level_0: all
If ((price - cost) > $50) and ((price - cost) ≤ $250))
Level_1: high_profit_margin < level_0: all
Syntax for Interestingness Measures Specification
Interestingness measures and thresholds can be specified by the user with the statement −
With <interest_measure_name> threshold = threshold_value
For Example −
With support threshold = 0.05
With confidence threshold = 0.7
Syntax for Pattern Presentation and Visualization Specification
We have a syntax, which allows users to specify the display of discovered patterns in one or more forms.
Display as <result_form>
For Example −
Display as table
Full Specification of DMQL
As a market manager of a company, you would like to characterize the buying habits of customers who can purchase items priced at no less than $100; with respect to the customer's age, type of item purchased, and the place where the item was purchased. You would like to know the percentage of customers having that characteristic. In particular, you are only interested in purchases made in Canada, and paid with an American Express credit card. You would like to view the resulting descriptions in the form of a table.
Use database AllElectronics_db
Use hierarchy location_hierarchy for B.address
Mine characteristics as customerPurchasing
Analyze count%
In relevance to C.age,I.type,I.place_made
From customer C, item I, purchase P, items_sold S, branch B
Where I.item_ID = S.item_ID and P.cust_ID = C.cust_ID and
P.method_paid = "AmEx" and B.address = "Canada" and I.price ≥ 100
With noise threshold = 5%
Display as table
The significant components of data mining systems are a data source, data mining engine, data warehouse server, the pattern evaluation module, graphical user interface, and knowledge base.
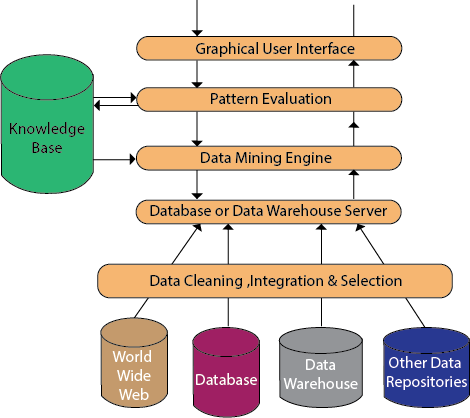
Data Source:
The actual source of data is the Database, data warehouse, World Wide Web (WWW), text files, and other documents. You need a huge amount of historical data for data mining to be successful. Organizations typically store data in databases or data warehouses. Data warehouses may comprise one or more databases, text files spreadsheets, or other repositories of data. Sometimes, even plain text files or spreadsheets may contain information. Another primary source of data is the World Wide Web or the internet.
Different processes:
Before passing the data to the database or data warehouse server, the data must be cleaned, integrated, and selected. As the information comes from various sources and in different formats, it can't be used directly for the data mining procedure because the data may not be complete and accurate. So, the first data requires to be cleaned and unified. More information than needed will be collected from various data sources, and only the data of interest will have to be selected and passed to the server. These procedures are not as easy as we think. Several methods may be performed on the data as part of selection, integration, and cleaning.
Database or Data Warehouse Server:
The database or data warehouse server consists of the original data that is ready to be processed. Hence, the server is cause for retrieving the relevant data that is based on data mining as per user request.
Data Mining Engine:
The data mining engine is a major component of any data mining system. It contains several modules for operating data mining tasks, including association, characterization, classification, clustering, prediction, time-series analysis, etc.
In other words, we can say data mining is the root of our data mining architecture. It comprises instruments and software used to obtain insights and knowledge from data collected from various data sources and stored within the data warehouse.
Pattern Evaluation Module:
The Pattern evaluation module is primarily responsible for the measure of investigation of the pattern by using a threshold value. It collaborates with the data mining engine to focus the search on exciting patterns.
This segment commonly employs stake measures that cooperate with the data mining modules to focus the search towards fascinating patterns. It might utilize a stake threshold to filter out discovered patterns. On the other hand, the pattern evaluation module might be coordinated with the mining module, depending on the implementation of the data mining techniques used. For efficient data mining, it is abnormally suggested to push the evaluation of pattern stake as much as possible into the mining procedure to confine the search to only fascinating patterns.
Graphical User Interface:
The graphical user interface (GUI) module communicates between the data mining system and the user. This module helps the user to easily and efficiently use the system without knowing the complexity of the process. This module cooperates with the data mining system when the user specifies a query or a task and displays the results.
Knowledge Base:
The knowledge base is helpful in the entire process of data mining. It might be helpful to guide the search or evaluate the stake of the result patterns. The knowledge base may even contain user views and data from user experiences that might be helpful in the data mining process. The data mining engine may receive inputs from the knowledge base to make the result more accurate and reliable. The pattern assessment module regularly interacts with the knowledge base to get inputs, and also update it.
Reference Books
1 Data Mining : Next Generation Challenges and Future Direction by Kargupta, et al, PHI.
2 Data Warehousing, Data Mining & OLAP by Alex Berson Stephen J.Smith.