Unit - 1
Introduction
What is Artificial Intelligence?
In today's world, technology is growing very fast, and we are getting in touch with different new technologies day by day.
Here, one of the booming technologies of computer science is Artificial Intelligence which is ready to create a new revolution in the world by making intelligent machines. The Artificial Intelligence is now all around us. It is currently working with a variety of subfields, ranging from general to specific, such as self-driving cars, playing chess, proving theorems, playing music, Painting, etc.
AI is one of the fascinating and universal fields of Computer science which has a great scope in future. AI holds a tendency to cause a machine to work as a human.
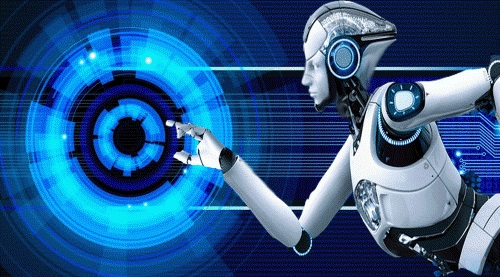
Artificial Intelligence is composed of two words Artificial and Intelligence, where Artificial defines "man-made," and intelligence defines "thinking power", hence AI means "a man-made thinking power."
So, we can define AI as:
"It is a branch of computer science by which we can create intelligent machines which can behave like a human, think like humans, and able to make decisions."
Artificial Intelligence exists when a machine can have human based skills such as learning, reasoning, and solving problems
With Artificial Intelligence you do not need to pre-program a machine to do some work, despite that you can create a machine with programmed algorithms which can work with own intelligence, and that is the awesomeness of AI.
It is believed that AI is not a new technology, and some people says that as per Greek myth, there were Mechanical men in early days which can work and behave like humans.
Why Artificial Intelligence?
Before Learning about Artificial Intelligence, we should know that what is the importance of AI and why should we learn it. Following are some main reasons to learn about AI:
- With the help of AI, you can create such software or devices which can solve real-world problems very easily and with accuracy such as health issues, marketing, traffic issues, etc.
- With the help of AI, you can create your personal virtual Assistant, such as Cortana, Google Assistant, Siri, etc.
- With the help of AI, you can build such Robots which can work in an environment where survival of humans can be at risk.
- AI opens a path for other new technologies, new devices, and new Opportunities.
Goals of Artificial Intelligence
Following are the main goals of Artificial Intelligence:
- Replicate human intelligence
- Solve Knowledge-intensive tasks
- An intelligent connection of perception and action
- Building a machine which can perform tasks that requires human intelligence such as:
- Proving a theorem
- Playing chess
- Plan some surgical operation
- Driving a car in traffic
- Creating some system which can exhibit intelligent behavior, learn new things by itself, demonstrate, explain, and can advise to its user.
What Comprises to Artificial Intelligence?
Artificial Intelligence is not just a part of computer science even it's so vast and requires lots of other factors which can contribute to it. To create the AI first we should know that how intelligence is composed, so the Intelligence is an intangible part of our brain which is a combination of Reasoning, learning, problem-solving perception, language understanding, etc.
To achieve the above factors for a machine or software Artificial Intelligence requires the following discipline:
- Mathematics
- Biology
- Psychology
- Sociology
- Computer Science
- Neurons Study
- Statistics
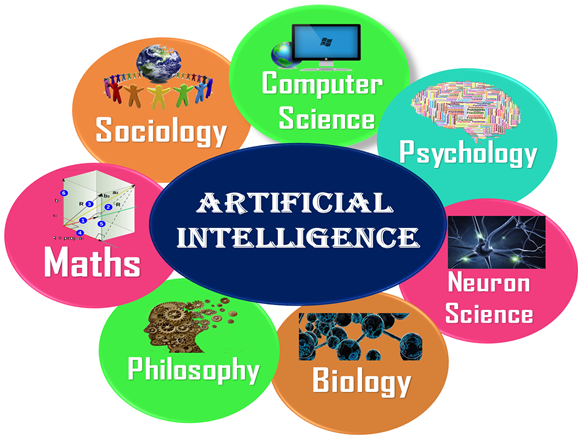
Advantages of Artificial Intelligence
Following are some main advantages of Artificial Intelligence:
- High Accuracy with less errors: AI machines or systems are prone to less errors and high accuracy as it takes decisions as per pre-experience or information.
- High-Speed: AI systems can be of very high-speed and fast-decision making, because of that AI systems can beat a chess champion in the Chess game.
- High reliability: AI machines are highly reliable and can perform the same action multiple times with high accuracy.
- Useful for risky areas: AI machines can be helpful in situations such as defusing a bomb, exploring the ocean floor, where to employ a human can be risky.
- Digital Assistant: AI can be very useful to provide digital assistant to the users such as AI technology is currently used by various E-commerce websites to show the products as per customer requirement.
- Useful as a public utility: AI can be very useful for public utilities such as a self-driving car which can make our journey safer and hassle-free, facial recognition for security purpose, Natural language processing to communicate with the human in human-language, etc.
Disadvantages of Artificial Intelligence
Every technology has some disadvantages, and the same goes for Artificial intelligence. Being so advantageous technology still, it has some disadvantages which we need to keep in our mind while creating an AI system. Following are the disadvantages of AI:
- High Cost: The hardware and software requirement of AI is very costly as it requires lots of maintenance to meet current world requirements.
- Can't think out of the box: Even we are making smarter machines with AI, but still they cannot work out of the box, as the robot will only do that work for which they are trained, or programmed.
- No feelings and emotions: AI machines can be an outstanding performer, but still it does not have the feeling so it cannot make any kind of emotional attachment with human, and may sometime be harmful for users if the proper care is not taken.
- Increase dependency on machines: With the increment of technology, people are getting more dependent on devices and hence they are losing their mental capabilities.
- No Original Creativity: As humans are so creative and can imagine some new ideas but still AI machines cannot beat this power of human intelligence and cannot be creative and imaginative.
Artificial Intelligence is not a new word and not a new technology for researchers. This technology is much older than you would imagine. Even there are the myths of Mechanical men in Ancient Greek and Egyptian Myths. Following are some milestones in the history of AI which defines the journey from the AI generation to till date development.
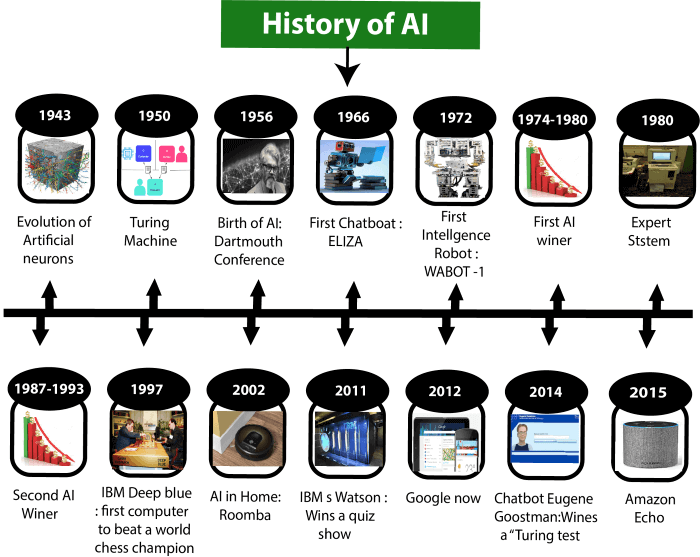
Maturation of Artificial Intelligence (1943-1952)
- Year 1943: The first work which is now recognized as AI was done by Warren McCulloch and Walter pits in 1943. They proposed a model of artificial neurons.
- Year 1949: Donald Hebb demonstrated an updating rule for modifying the connection strength between neurons. His rule is now called Hebbian learning.
- Year 1950: The Alan Turing who was an English mathematician and pioneered Machine learning in 1950. Alan Turing publishes "Computing Machinery and Intelligence" in which he proposed a test. The test can check the machine's ability to exhibit intelligent behavior equivalent to human intelligence, called a Turing test.
The birth of Artificial Intelligence (1952-1956)
- Year 1955: An Allen Newell and Herbert A. Simon created the "first artificial intelligence program "Which was named as "Logic Theorist". This program had proved 38 of 52 Mathematics theorems, and find new and more elegant proofs for some theorems.
- Year 1956: The word "Artificial Intelligence" first adopted by American Computer scientist John McCarthy at the Dartmouth Conference. For the first time, AI coined as an academic field.
At that time high-level computer languages such as FORTRAN, LISP, or COBOL were invented. And the enthusiasm for AI was very high at that time.
The golden years-Early enthusiasm (1956-1974)
- Year 1966: The researchers emphasized developing algorithms which can solve mathematical problems. Joseph Weizenbaum created the first chatbot in 1966, which was named as ELIZA.
- Year 1972: The first intelligent humanoid robot was built in Japan which was named as WABOT-1.
The first AI winter (1974-1980)
- The duration between years 1974 to 1980 was the first AI winter duration. AI winter refers to the time period where computer scientist dealt with a severe shortage of funding from government for AI researches.
- During AI winters, an interest of publicity on artificial intelligence was decreased.
A boom of AI (1980-1987)
- Year 1980: After AI winter duration, AI came back with "Expert System". Expert systems were programmed that emulate the decision-making ability of a human expert.
- In the Year 1980, the first national conference of the American Association of Artificial Intelligence was held at Stanford University.
The second AI winter (1987-1993)
- The duration between the years 1987 to 1993 was the second AI Winter duration.
- Again, Investors and government stopped in funding for AI research as due to high cost but not efficient result. The expert system such as XCON was very cost effective.
The emergence of intelligent agents (1993-2011)
- Year 1997: In the year 1997, IBM Deep Blue beats world chess champion, Gary Kasparov, and became the first computer to beat a world chess champion.
- Year 2002: for the first time, AI entered the home in the form of Roomba, a vacuum cleaner.
- Year 2006: AI came in the Business world till the year 2006. Companies like Facebook, Twitter, and Netflix also started using AI.
Deep learning, big data and artificial general intelligence (2011-present)
- Year 2011: In the year 2011, IBM's Watson won jeopardy, a quiz show, where it had to solve the complex questions as well as riddles. Watson had proved that it could understand natural language and can solve tricky questions quickly.
- Year 2012: Google has launched an Android app feature "Google now", which was able to provide information to the user as a prediction.
- Year 2014: In the year 2014, Chatbot "Eugene Goostman" won a competition in the infamous "Turing test."
- Year 2018: The "Project Debater" from IBM debated on complex topics with two master debaters and also performed extremely well.
- Google has demonstrated an AI program "Duplex" which was a virtual assistant and which had taken hairdresser appointment on call, and lady on other side didn't notice that she was talking with the machine.
Now AI has developed to a remarkable level. The concept of Deep learning, big data, and data science are now trending like a boom. Nowadays companies like Google, Facebook, IBM, and Amazon are working with AI and creating amazing devices. The future of Artificial Intelligence is inspiring and will come with high intelligence.
1. Al in E-commerce
The internet has opened the door for revolutionizing various sectors. E-commerce sector is that of the one of them. E-commerce sectors have unlocked that of the new opportunities and the scope for retailers. Retailers also have never seen such a growth in their sales. Artificial intelligence is taking that of the E-commerce to the next level. In this article, we are going to discuss 10 applications of artificial intelligence in E-commerce.
1. Chatbots
E-commerce websites are using chatbots to improve the customer support service. Chatbots are providing 24/7 customer support to buyers. Visit any recognized E-commerce website. You will be prompted with a chat box asking what do you want or how can I help. You can tell your requirements in the chatbox and you will be served with highly filtered results. Chatbots are built using artificial intelligence and are able to communicate with humans. They can also collect your past data and provide you with a very personalized user experience.
2. Image search
Ever come across a situation where you liked any product or item but don’t what it is called or what it is? Artificial intelligence service eases this type of task for you. The concept of that of the image search is implemented in the E-commerce websites with the application of the artificial intelligence. Artificial intelligence has made it possible to understand images. Buyers can make a search on the basis of images. Mobile apps of E-commerce websites can find the product by just pointing the camera towards the product. This eliminates the need for keyword searches.
3. Handling Customer Data
E-commerce platforms have two things in abundance. On is an endless list of products and other is data. E-commerce has to deal with a lot of data every day. This data can be anything like daily sales, the total number of items sold, the number of orders received in an area or as a whole and what not. It has to take care of customer data also. Handling that amount of data is not possible for a human. Artificial intelligence can not only collect this data in a more structured form but also generate proper insights out of this data.
This helps in understanding the customer behaviour of the whole populations as well as of the individual buyer. Understanding the customer buying pattern can make E-commerce to make changes wherever needed and predicting the next buy of the user also.
4. Recommendation Systems
Have you ever experienced that how E-commerce websites like that of the Amazon is constantly showing that of the products similar you just checked? Well, this is the application of that of the artificial intelligence in E-commerce. AI and machine learning algorithms can predict the behaviour of the buyer from its past searches, likings, frequently bought products. By predicting the behaviour of the user, E-commerce websites are able to recommend the products that user is highly interested in. This improves the user experience as the user no longer has to spend hours searching the product. It also helps the E-commerce websites to improve that of their sales.
5. Inventory management
The inventory management is one of the most important areas in any business. You have to keep yourself updated on how much inventory you are holding and how much more is needed. There are thousands of product categories over the E-commerce websites. Keeping an eye on the inventory of all the products daily is not possible for a human. This is where artificial intelligence comes into that of the picture. Artificial intelligence applications have helped E-commerce in managing the inventory. Moreover, the inventory management system will get better over the time. AI systems build a correlation between the current demand and the future demand.
6. Cybersecurity
Artificial intelligence has also improved that of the cybersecurity of the E-commerce websites. It can prevent or detect any kind of fraudulent activities. E-Commerce has to deal with a lot of transactions on that of the daily basis. Cybercriminals and hackers can hack the user account to gain unauthenticated access. This can lead to that of the exposure of the private data and the online fraud. The reputation of that of the business also gets a very big blow. To prevent this, Artificial intelligence and the machine learning algorithms are developed that can mitigate that of the chances of fraud activities over that of the website.
7. Better Decision Making
E-commerce can make better decisions with that of the application of Artificial intelligence. Data analysts have to handle a lot of data every day. This data is very big for them to handle. Moreover, analysing the data also becomes a difficult task. Artificial intelligence has fastened that of the decision-making process of the E-Commerce. AI algorithms can easily identify the complex patterns in the data by predicting user behaviour and their purchasing pattern.
8. After Sales Service
Selling the product is not enough. Businesses have to aid that of the customer in the complete buying cycle. After sales service is an integral part of after-sales service. Artificial intelligence applications can automate the feedback form, replacements and handling any other ambiguity in the product. By solving the buyer’s issues, the brand value of the website gets improved.
9. CRM
In the past, Customer Relationship Management (CRM) relied on the people to collect a huge amount of data in order to collect the data and serve the clients. But today, artificial intelligence can predict which customers are most likely to make a purchase and how can we better engage with them. Artificial intelligence applications can help in identifying the trends and plan the actions according to the latest trends. With the help of machine learning algorithms, advanced CRM can learn and improve over time.
10. Sales Improvement
Artificial intelligence applications can generate and predict the accurate forecast of the E-commerce business. The study of historical data, data analytics, and latest trends can help in optimizing the resource allocation, build a healthy pipeline and analyse the team performance. The managers can get a better insight into the latest trends in sales. They can analyse the trends and can improve the sales by making strategies well before time.
Conclusion
Digital Platform has made life easier for that of the retailers as well as that of the buyers. E-commerce websites are witnessing that of an exponential hike in that of their sales. Artificial intelligence has helped E-Commerce websites in providing the better user experience. Artificial intelligence research in that of the field of E-commerce is leveraging that of the sales of E-commerce too.
2. Al in Industry
To define industrial AI, we must first define that of the AI itself. Although the field of artificial intelligence has existed for over half a century, it has no clear and all-encompassing definition. Further, the lines between AI and adjacent fields like machine learning, big data, predictive analytics, and IoT are often blurred, as are the lines between AI and subfields like deep neural networks and cognitive computing.
For our purposes, artificial intelligence refers to those computer science techniques and technologies that allow software to exhibit ‘smarts’—in other words, to do things that seem human-like. This can include things like making decisions, recognizing objects, or understanding speech. It really is a verybroad term.
Strictly speaking, machine learning (ML) is a subset of AI. ML refers to a set of techniques that allow us to create AI software by training that software with data) to display some desired intelligent behaviour. This is as opposed to, for example, explicitly programming our software with a bunch of rules to generate our desired behaviour—and it’s a very powerful concept.
It is for this reason that, while machine learning is only one way to build an artificially intelligent system, for all practical purposes ML and AI are used interchangeably today. All the interesting activity in AI is in machine learning.
What about cognitive computing? It’s a bit more esoteric a term, usually used to highlight capabilities akin to humans’ higher level thinking and reasoning skills. An example would be the ability to determine the sentiment expressed in text or images, or what objects are present in pictures. But again, for all practical purposes, the term is most often used interchangeably with AI—in fact, it’s the preferred term in some regions of the world—and the work in this field is based upon machine learning.
How does this relate to big data? Well, data is used to train the machines, and the more you have of it the better (assuming it’s high quality data).9 And how about predictive analytics? Well, machine learning can be a more powerful way to make predictions, and one that can learn from patterns in the data. But simple averages and other formulas can be used for predictions as well... These need not be based on ML/AI.
Finally, for enterprises whose operations involve the physical world, the industrial internet of things (IoT/IIoT) is an increasingly important source of insight into the status, location and performance of enterprise assets (see Figure 1). Because IoT devices and sensors can number into the millions, and can report status with millisecond resolution, the resulting data volumes can quickly become voluminous, lending themselves to the application of machine learning techniques
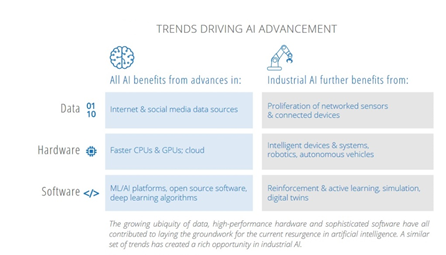
To what then does the term “industrial AI” refer? Well, certainly the word industrial has certain immediate connotations, primarily of manufacturing and heavy industry. But to limit our scope to just those industries would be to miss the less obvious connections between a broad set of related use cases, the environments they exist within, and the common challenges and requirements that they give rise to.
Rather than referring to a set of vertical industries, by industrial AI we’re referring to a class of applications that can exist within any vertical:
We define industrial AI as any application of AI relating to the physical operations or systems of an enterprise. Industrial AI is focused on that of the helping and the enterprise monitor, optimize or control the behaviour of these operations and systems to improve their efficiency and performance (see Figure 2).
According to this definition, industrial AI includes, for example, applications relating to the manufacture of physical products, to supply chains and ware-houses where physical items are stored and then moved, to that of the operation of building HVAC systems, and much more (see Figure 3). Any company in any industry can have opportunities to apply industrial
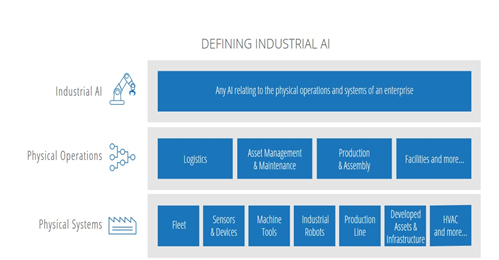
Due to the physical nature of the systems and processes to which they relate, industrial AI systems share similar characteristics and constraints. For example, the fact that industrial AI ultimately relates to the physical systems of an enter-prise tends to mean that access to training and test data is more difficult; the reliance on subject matter expertise is larger; the AI models themselves are harder to develop, train, and test; and the costs associated with their failure are greater. In other words, that the stakes are higher. We elaborate on the significance of this in the next section.
3. Al in Medicine
Many industries have been disrupted by that of the influx of the new technologies in that of the Information Age. Healthcare is no different. Particularly in that of the case of automation, machine learning, and the artificial intelligence (AI), the doctors, the hospitals, the insurance companies, and that of the industries with ties to that of the healthcare have all been impacted – in many of the cases in more positive, the substantial ways than that of the other industries.
According to a 2016 report from that of the CB Insights, about 86% of that of the healthcare provider organizations, the life science companies, and that of the technology vendors to that of the healthcare are using the artificial intelligence technology. By 2020, these of the organizations will spend an average of the $54 million on that of the artificial intelligence projects.
So what are the solutions are they most commonly implementing? Here are 10 common ways AI is changing that of the healthcare now and will in the future. They are as follows
1. Managing Medical Records and Other Data
Since the first step in that of the health care is compiling and then analysing information (like medical records and other past history), the data management is the most widely used application of that of the artificial intelligence and the digital automation. The Robots collect, the store, the re-format, and the trace data to provide faster, more consistent access.
2. Doing Repetitive Jobs
Analysing tests, the X-Rays, the CT scans, the data entry, and that of the other mundane tasks can all be done faster and more accurately by that of the robots. Cardiology and that of the radiology are two disciplines where the amount of data to analyse can that be overwhelming and very time consuming. Cardiologists and radiologists in that of the future should only look at the most complicated cases where human supervision is very useful.
3. Treatment Design
Artificial intelligence systems have been created to that of the analyse data – notes and reports from that of a patient’s file, external research, and clinical expertise – to help select the correct, individually customized treatment path.
4. Digital Consultation
Apps like Babylon in that of the UK use AI to give medical consultation based on the personal medical history and that of the common medical knowledge. Users report their symptoms into the app, which uses that of the speech recognition to compare against a database of the illnesses. Babylon then offers a recommended action, taking into that of the account the user’s medical history.
5. Virtual Nurses
The start-up Sense has developed Molly which is a digital nurse to help that of the people monitor patient’s condition and then follow up with the treatments, between doctor visits. The program uses machine learning to support that of the patients, specializing in chronic illnesses.
In 2016, Boston Children’s Hospital developed an app for Amazon Alexa that gives basic health information and advice for parents of ill children. The app answers asked questions about medications and whether symptoms require a doctor visit.
6. Medication Management
The National Institutes of Health have created that of the AiCure app to monitor the use of medication by a patient. A smartphone’s webcam is partnered with AI to autonomously confirm that patients are taking their prescriptions and helps them manage their condition. Most common users could be people with serious medical conditions, patients who tend to go against doctor advice, and participants in clinical trials.
7. Drug Creation
Developing pharmaceuticals through that of the clinical trials can take more than a decade and cost billions of dollars. Making this process faster and that of the cheaper could change the world. Amidst the recent Ebola virus scare, a program powered by that of the AI was used to scan existing medicines that could be redesigned to fight the disease.
The program found two of the medications that may reduce Ebola infectivity in one day, when analysis of this type generally takes months or years – a difference that could mean saving thousands of lives.
8. Precision Medicine
Genetics and genomics look for that of the mutations and the links to disease from that of the information in DNA. With the help of the AI, body scans can spot cancer and the vascular diseases early and then predict the health issues people might face based on their genetics.
9. Health Monitoring
Wearable health trackers – like those from that of the FitBit, Apple, Garmin and others – monitors heart rate and activity levels. They can send the alerts to the user to get more exercise and can share this type of information to the doctors (and AI systems) for additional data points on the needs and habits of that of the patients.
10. Healthcare System Analysis
In the Netherlands, 97% of that of the healthcare invoices are digital. A Dutch company uses AI to sift through that of the data to highlight the mistakes in treatments, workflow inefficiencies, and then helps area healthcare systems avoid unnecessary patient hospitalizations.
These are just a sample of that of the solutions AI is offering the healthcare industry. As innovation pushes the capabilities of that of the automation and the digital workforces, from providers like Novation, more solutions to save time, lower the costs, and increase the accuracy will be possible.
Agents in Artificial Intelligence
An AI system can be defined as the study of the rational agent and its environment. The agents sense the environment through sensors and act on their environment through actuators. An AI agent can have mental properties such as knowledge, belief, intention, etc.
What is an Agent?
An agent can be anything that perceive its environment through sensors and act upon that environment through actuators. An Agent runs in the cycle of perceiving, thinking, and acting. An agent can be:
- Human-Agent: A human agent has eyes, ears, and other organs which work for sensors and hand, legs, vocal tract work for actuators.
- Robotic Agent: A robotic agent can have cameras, infrared range finder, NLP for sensors and various motors for actuators.
- Software Agent: Software agent can have keystrokes, file contents as sensory input and act on those inputs and display output on the screen.
Hence the world around us is full of agents such as thermostat, cell phone, camera, and even we are also agents.
Before moving forward, we should first know about sensors, effectors, and actuators.
Sensor: Sensor is a device which detects the change in the environment and sends the information to other electronic devices. An agent observes its environment through sensors.
Actuators: Actuators are the component of machines that converts energy into motion. The actuators are only responsible for moving and controlling a system. An actuator can be an electric motor, gears, rails, etc.
Effectors: Effectors are the devices which affect the environment. Effectors can be legs, wheels, arms, fingers, wings, fins, and display screen.
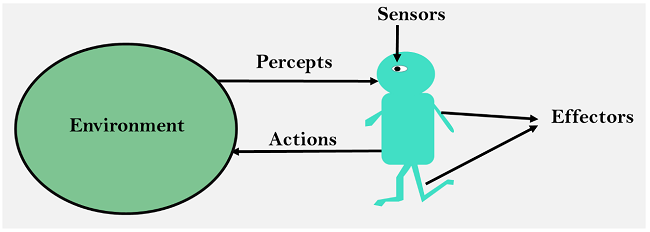
Intelligent Agents:
An intelligent agent is an autonomous entity which act upon an environment using sensors and actuators for achieving goals. An intelligent agent may learn from the environment to achieve their goals. A thermostat is an example of an intelligent agent.
Following are the main four rules for an AI agent:
- Rule 1: An AI agent must have the ability to perceive the environment.
- Rule 2: The observation must be used to make decisions.
- Rule 3: Decision should result in an action.
- Rule 4: The action taken by an AI agent must be a rational action.
Rational Agent:
A rational agent is an agent which has clear preference, models uncertainty, and acts in a way to maximize its performance measure with all possible actions.
A rational agent is said to perform the right things. AI is about creating rational agents to use for game theory and decision theory for various real-world scenarios.
For an AI agent, the rational action is most important because in AI reinforcement learning algorithm, for each best possible action, agent gets the positive reward and for each wrong action, an agent gets a negative reward.
Note: Rational agents in AI are very similar to intelligent agents.
Rationality:
The rationality of an agent is measured by its performance measure. Rationality can be judged on the basis of following points:
- Performance measure which defines the success criterion.
- Agent prior knowledge of its environment.
- Best possible actions that an agent can perform.
- The sequence of percepts.
Note: Rationality differs from Omniscience because an Omniscient agent knows the actual outcome of its action and act accordingly, which is not possible in reality.
Structure of an AI Agent
The task of AI is to design an agent program which implements the agent function. The structure of an intelligent agent is a combination of architecture and agent program. It can be viewed as:
- Agent = Architecture + Agent program
Following are the main three terms involved in the structure of an AI agent:
Architecture: Architecture is machinery that an AI agent executes on.
Agent Function: Agent function is used to map a percept to an action.
- f:P* → A
Agent program: Agent program is an implementation of agent function. An agent program executes on the physical architecture to produce function f.
PEAS Representation
PEAS is a type of model on which an AI agent works upon. When we define an AI agent or rational agent, then we can group its properties under PEAS representation model. It is made up of four words:
- P: Performance measure
- E: Environment
- A: Actuators
- S: Sensors
Here performance measure is the objective for the success of an agent's behavior.
PEAS for self-driving cars:
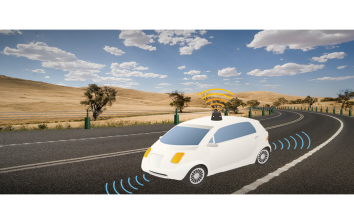
Let's suppose a self-driving car then PEAS representation will be:
Performance: Safety, time, legal drive, comfort
Environment: Roads, other vehicles, road signs, pedestrian
Actuators: Steering, accelerator, brake, signal, horn
Sensors: Camera, GPS, speedometer, odometer, accelerometer, sonar.
Example of Agents with their PEAS representation
Agent | Performance measure | Environment | Actuators | Sensors |
1. Medical Diagnose |
|
|
| Keyboard |
2. Vacuum Cleaner |
|
|
|
|
3. Part -picking Robot |
|
|
|
|
An intelligent agent (IA) is an entity that makes a decision,that enables artificial intelligence to be put into action. It can also be described as a software entity that conducts operations in the place of users or programs after sensing the environment. It uses actuators to initiate action in that environment.
Characteristics of intelligent agents
Intelligent agents have the following distinguishing characteristics:
- They have some level of autonomy that allows them to perform certain tasks on their own.
- They have a learning ability that enables them to learn even as tasks are carried out.
- They can interact with other entities such as agents, humans, and systems.
- New rules can be accommodated by intelligent agents incrementally.
- They exhibit goal-oriented habits.
- They are knowledge-based. They use knowledge regarding communications, processes, and entities.
The structure of intelligent agents
The IA structure consists of three main parts: architecture, agent function, and agent program.
- Architecture: This refers to machinery or devices that consists of actuators and sensors. The intelligent agent executes on this machinery. Examples include a personal computer, a car, or a camera.
- Agent function: This is a function in which actions are mapped from a certain percept sequence. Percept sequence refers to a history of what the intelligent agent has perceived.
- Agent program: This is an implementation or execution of the agent function. The agent function is produced through the agent program’s execution on the physical architecture.
Categories of intelligent agents
There are 5 main categories of intelligent agents. The grouping of these agents is based on their capabilities and level of perceived intelligence.
Simple reflex agents
These agents perform actions using the current percept, rather than the percept history. The condition-action rule is used as the basis for the agent function. In this category, a fully observable environment is ideal for the success of the agent function.
Model-based reflex agents
Unlike simple reflex agents, model-based reflex agents consider the percept history in their actions. The agent function can still work well even in an environment that is not fully observable. These agents use an internal model that determines the percept history and effect of actions. They reflect on certain aspects of the present state that have been unobserved.
Goal-based agents
These agents have higher capabilities than model-based reflex agents. Goal-based agents use goal information to describe desirable capabilities. This allows them to choose among various possibilities. These agents select the best action that enhances the attainment of the goal.
Utility-based agents
These agents make choices based on utility. They are more advanced than goal-based agents because of an extra component of utility measurement. Using a utility function, a state is mapped against a certain measure of utility. A rational agent selects the action that optimizes the expected utility of the outcome.
Learning agents
These are agents that have the capability of learning from their previous experience.
Learning agents have the following elements.
- The learning element: This element enables learning agents to learn from previous experiences.
- The critic: It provides feedback on how the agent is doing.
- The performance element: This element decides on the external action that needs to be taken.
- The problem generator: This acts as a feedback agent that performs certain tasks such as making suggestions (new) and keeping history.
How intelligent agents work
Intelligent agents work through three main components: sensors, actuators, and effectors. Getting an overview of these components can improve our understanding of how intelligent agents work.
- Sensors: These are devices that detect any changes in the environment. This information is sent to other devices. In artificial intelligence, the environment of the system is observed by intelligent agents through sensors.
- Actuators: These are components through which energy is converted into motion. They perform the role of controlling and moving a system. Examples include rails, motors, and gears.
- Effectors: The environment is affected by effectors. Examples include legs, fingers, wheels, display screen, and arms.
Computer vision is a field of study focused on the problem of helping computers to see.
At an abstract level, the goal of computer vision problems is to use the observed image data to infer something about the world.
It is a multidisciplinary field that could broadly be called a subfield of artificial intelligence and machine learning, which may involve the use of specialized methods and make use of general learning algorithms.
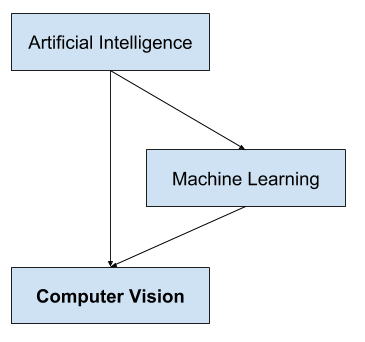
Overview of the Relationship of Artificial Intelligence and Computer Vision
As a multidisciplinary area of study, it can look messy, with techniques borrowed and reused from a range of disparate engineering and computer science fields.
One particular problem in vision may be easily addressed with a hand-crafted statistical method, whereas another may require a large and complex ensemble of generalized machine learning algorithms.
Computer vision as a field is an intellectual frontier. Like any frontier, it is exciting and disorganized, and there is often no reliable authority to appeal to. Many useful ideas have no theoretical grounding, and some theories are useless in practice; developed areas are widely scattered, and often one looks completely inaccessible from the other.
The goal of computer vision is to understand the content of digital images. Typically, this involves developing methods that attempt to reproduce the capability of human vision.
Understanding the content of digital images may involve extracting a description from the image, which may be an object, a text description, a three-dimensional model, and so on.
Computer vision is the automated extraction of information from images. Information can mean anything from 3D models, camera position, object detection and recognition to grouping and searching image content.
Computer Vision and Image Processing
Computer vision is distinct from image processing.
Image processing is the process of creating a new image from an existing image, typically simplifying or enhancing the content in some way. It is a type of digital signal processing and is not concerned with understanding the content of an image.
A given computer vision system may require image processing to be applied to raw input, e.g. Pre-processing images.
Examples of image processing include:
- Normalizing photometric properties of the image, such as brightness or color.
- Cropping the bounds of the image, such as centering an object in a photograph.
- Removing digital noise from an image, such as digital artifacts from low light levels.
Challenge of Computer Vision
Helping computers to see turns out to be very hard.
The goal of computer vision is to extract useful information from images. This has proved a surprisingly challenging task; it has occupied thousands of intelligent and creative minds over the last four decades, and despite this we are still far from being able to build a general-purpose “seeing machine.”
Computer vision seems easy, perhaps because it is so effortless for humans.
Initially, it was believed to be a trivially simple problem that could be solved by a student connecting a camera to a computer. After decades of research, “computer vision” remains unsolved, at least in terms of meeting the capabilities of human vision.
Making a computer see was something that leading experts in the field of Artificial Intelligence thought to be at the level of difficulty of a summer student’s project back in the sixties. Forty years later the task is still unsolved and seems formidable.
One reason is that we don’t have a strong grasp of how human vision works.
Studying biological vision requires an understanding of the perception organs like the eyes, as well as the interpretation of the perception within the brain. Much progress has been made, both in charting the process and in terms of discovering the tricks and shortcuts used by the system, although like any study that involves the brain, there is a long way to go.
Perceptual psychologists have spent decades trying to understand how the visual system works and, even though they can devise optical illusions to tease apart some of its principles, a complete solution to this puzzle remains elusive
Another reason why it is such a challenging problem is because of the complexity inherent in the visual world.
A given object may be seen from any orientation, in any lighting conditions, with any type of occlusion from other objects, and so on. A true vision system must be able to “see” in any of an infinite number of scenes and still extract something meaningful.
Computers work well for tightly constrained problems, not open unbounded problems like visual perception.
Tasks in Computer Vision
Nevertheless, there has been progress in the field, especially in recent years with commodity systems for optical character recognition and face detection in cameras and smartphones.
Computer vision is at an extraordinary point in its development. The subject itself has been around since the 1960s, but only recently has it been possible to build useful computer systems using ideas from computer vision.
- Optical character recognition (OCR)
- Machine inspection
- Retail (e.g. Automated checkouts)
- 3D model building (photogrammetry)
- Medical imaging
- Automotive safety
- Match move (e.g. Merging CGI with live actors in movies)
- Motion capture (mocap)
- Surveillance
- Fingerprint recognition and biometrics
It is a broad area of study with many specialized tasks and techniques, as well as specializations to target application domains.
Computer vision has a wide variety of applications, both old (e.g., mobile robot navigation, industrial inspection, and military intelligence) and new (e.g., human computer interaction, image retrieval in digital libraries, medical image analysis, and the realistic rendering of synthetic scenes in computer graphics).
It may be helpful to zoom in on some of the more simpler computer vision tasks that you are likely to encounter or be interested in solving given the vast number of publicly available digital photographs and videos available.
Many popular computer vision applications involve trying to recognize things in photographs; for example:
- Object Classification: What broad category of object is in this photograph?
- Object Identification: Which type of a given object is in this photograph?
- Object Verification: Is the object in the photograph?
- Object Detection: Where are the objects in the photograph?
- Object Landmark Detection: What are the key points for the object in the photograph?
- Object Segmentation: What pixels belong to the object in the image?
- Object Recognition: What objects are in this photograph and where are they?
Other common examples are related to information retrieval; for example: finding images like an image or images that contain an object.
Summary
In this post, you discovered a gentle introduction to the field of computer vision.
Specifically, you learned:
- The goal of the field of computer vision and its distinctness from image processing.
- What makes the problem of computer vision challenging.
- Typical problems or tasks pursued in computer vision.
Natural Language Processing (NLP) refers to AI method of communicating with an intelligent system using a natural language such as English.
Processing of Natural Language is required when you want an intelligent system like robot to perform as per your instructions, when you want to hear decision from a dialogue based clinical expert system, etc.
The field of NLP involves making computers to perform useful tasks with the natural language’s humans use. The input and output of an NLP system can be −
- Speech
- Written Text
Components of NLP
There are two components of NLP as given −
Natural Language Understanding (NLU)
Understanding involves the following tasks −
- Mapping the given input in natural language into useful representations.
- Analyzing different aspects of the language.
Natural Language Generation (NLG)
It is the process of producing meaningful phrases and sentences in the form of natural language from some internal representation.
It involves −
- Text planning− It includes retrieving the relevant content from knowledge base.
- Sentence planning− It includes choosing required words, forming meaningful phrases, setting tone of the sentence.
- Text Realization− It is mapping sentence plan into sentence structure.
The NLU is harder than NLG.
Difficulties in NLU
NL has an extremely rich form and structure.
It is very ambiguous. There can be different levels of ambiguity −
- Lexical ambiguity− It is at very primitive level such as word-level.
- For example, treating the word “board” as noun or verb?
- Syntax Level ambiguity− A sentence can be parsed in different ways.
- For example, “He lifted the beetle with red cap.” − Did he use cap to lift the beetle or he lifted a beetle that had red cap?
- Referential ambiguity− Referring to something using pronouns. For example, Rima went to Gauri. She said, “I am tired.” − Exactly who is tired?
- One input can mean different meanings.
- Many inputs can mean the same thing.
NLP Terminology
- Phonology− It is study of organizing sound systematically.
- Morphology− It is a study of construction of words from primitive meaningful units.
- Morpheme− It is primitive unit of meaning in a language.
- Syntax− It refers to arranging words to make a sentence. It also involves determining the structural role of words in the sentence and in phrases.
- Semantics− It is concerned with the meaning of words and how to combine words into meaningful phrases and sentences.
- Pragmatics− It deals with using and understanding sentences in different situations and how the interpretation of the sentence is affected.
- Discourse− It deals with how the immediately preceding sentence can affect the interpretation of the next sentence.
- World Knowledge− It includes the general knowledge about the world.
Steps in NLP
There are general five steps −
- Lexical Analysis− It involves identifying and analyzing the structure of words. Lexicon of a language means the collection of words and phrases in a language. Lexical analysis is dividing the whole chunk of txt into paragraphs, sentences, and words.
- Syntactic Analysis (Parsing)− It involves analysis of words in the sentence for grammar and arranging words in a manner that shows the relationship among the words. The sentence such as “The school goes to boy” is rejected by English syntactic analyzer.
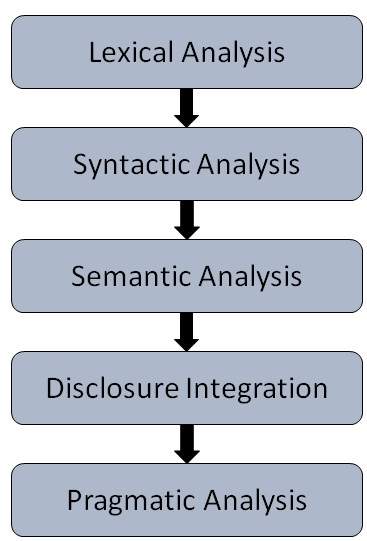
- Semantic Analysis− It draws the exact meaning or the dictionary meaning from the text. The text is checked for meaningfulness. It is done by mapping syntactic structures and objects in the task domain. The semantic analyzer disregards sentence such as “hot ice-cream”.
- Discourse Integration− The meaning of any sentence depends upon the meaning of the sentence just before it. In addition, it also brings about the meaning of immediately succeeding sentence.
- Pragmatic Analysis− During this, what was said is re-interpreted on what it actually meant. It involves deriving those aspects of language which require real world knowledge.
Implementation Aspects of Syntactic Analysis
There are a number of algorithms researchers have developed for syntactic analysis, but we consider only the following simple methods −
- Context-Free Grammar
- Top-Down Parser
Let us see them in detail −
Context-Free Grammar
It is the grammar that consists rules with a single symbol on the left-hand side of the rewrite rules. Let us create grammar to parse a sentence −
“The bird pecks the grains”
Articles (DET)− a | an | the
Nouns− bird | birds | grain | grains
Noun Phrase (NP)− Article + Noun | Article + Adjective + Noun
= DET N | DET ADJ N
Verbs− pecks | pecking | pecked
Verb Phrase (VP)− NP V | V NP
Adjectives (ADJ)− beautiful | small | chirping
The parse tree breaks down the sentence into structured parts so that the computer can easily understand and process it. In order for the parsing algorithm to construct this parse tree, a set of rewrite rules, which describe what tree structures are legal, need to be constructed.
These rules say that a certain symbol may be expanded in the tree by a sequence of other symbols. According to first order logic rule, if there are two strings Noun Phrase (NP) and Verb Phrase (VP), then the string combined by NP followed by VP is a sentence. The rewrite rules for the sentence are as follows −
S → NP VP
NP → DET N | DET ADJ N
VP → V NP
Lexocon−
DET → a | the
ADJ → beautiful | perching
N → bird | birds | grain | grains
V → peck | pecks | pecking
The parse tree can be created as shown −
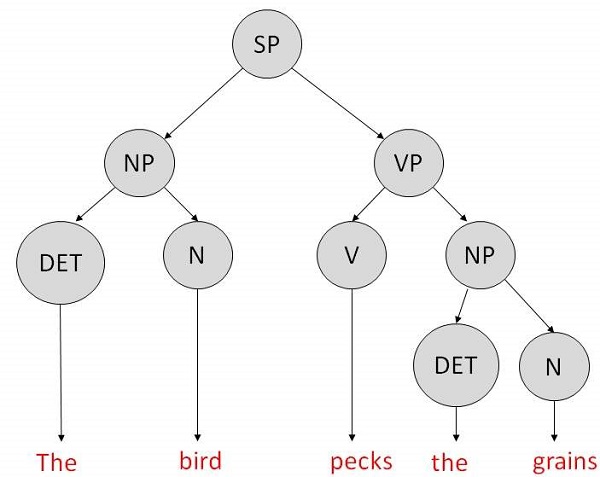
Now consider the above rewrite rules. Since V can be replaced by both, "peck" or "pecks", sentences such as "The bird peck the grains" can be wrongly permitted. i. e. The subject-verb agreement error is approved as correct.
Merit− The simplest style of grammar, therefore widely used one.
Demerits −
- They are not highly precise. For example, “The grains peck the bird”, is a syntactically correct according to parser, but even if it makes no sense, parser takes it as a correct sentence.
- To bring out high precision, multiple sets of grammar need to be prepared. It may require a completely different sets of rules for parsing singular and plural variations, passive sentences, etc., which can lead to creation of huge set of rules that are unmanageable.
Top-Down Parser
Here, the parser starts with the S symbol and attempts to rewrite it into a sequence of terminal symbols that matches the classes of the words in the input sentence until it consists entirely of terminal symbols.
These are then checked with the input sentence to see if it matched. If not, the process is started over again with a different set of rules. This is repeated until a specific rule is found which describes the structure of the sentence.
Merit− It is simple to implement.
Demerits −
- It is inefficient, as the search process has to be repeated if an error occurs.
- Slow speed of working.
References:
1. Stuart Russell, Peter Norvig, “Artificial Intelligence – A Modern Approach”, Pearson Education
2. Elaine Rich and Kevin Knight, “Artificial Intelligence”, McGraw-Hill
3. E Charniak and D McDermott, “Introduction to Artificial Intelligence”, Pearson Education
4. Dan W. Patterson, “Artificial Intelligence and Expert Systems”, Prentice Hall of India