Unit - 5
Learning
Changes in the system that allow it to perform the same task more efficiently the next time around. Learning is the process of creating or changing representations of what one is experiencing. Learning causes beneficial mental changes. Learning enhances comprehension and productivity.
Find out about new objects or structures that you didn't know about before (data mining, scientific discovery). Fill in any observations or requirements regarding a domain that are skeletal or incomplete (this expands the domain of expertise and lessens the brittleness of the system). Create software agents that can learn from their users and other software agents. Replicate a key feature of intelligent behavior.
Arthur Samuel, an American pioneer in the fields of computer games and artificial intelligence, invented the phrase Machine Learning in 1959, stating that "it offers computers the ability to learn without being expressly taught."
"A computer programme is said to learn from experience E with respect to some task T and some performance measure P, if its performance on T, as measured by P, increases with experience E," Tom Mitchell wrote in 1997.
At a high-level, machine learning is simply the study of teaching a computer programme or algorithm how to gradually improve upon a set task that it is given. On the research-side of things, machine learning can be seen through the prism of theoretical and mathematical simulation of how this method works. However, more technically it is the study of how to construct applications that exhibit this iterative progress.
Machine Learning is an Application of Artificial Intelligence (AI) that gives machines the opportunity to learn from their experiences and develop themselves without doing any coding.
Machine Learning is a branch of Artificial Intelligence. Machine Learning is the study of making machines more human-like in their actions and decisions by allowing them the ability to learn and create their own programmes. This is achieved with minimal human interference, i.e., no explicit programming. The learning process is automated and enhanced based on the experiences of the machines in the process.
In the real world, we are surrounded by individuals who can learn anything from their experiences thanks to their ability to learn, and we have computers or machines that follow our commands. But, like a human, can a machine learn from past experiences or data? So here's where Machine Learning comes in.
Machine learning is a subfield of artificial intelligence that focuses on the development of algorithms that allow a computer to learn on its own from data and previous experiences. Arthur Samuel was the first to coin the term "machine learning" in 1959. In a nutshell, we can characterize it as follows:
“Machine learning enables a machine to automatically learn from data, improve performance from experiences, and predict things without being explicitly programmed.”
Machine learning algorithms create a mathematical model with the help of sample historical data, referred to as training data, that aids in making predictions or judgments without being explicitly programmed. In order to create predictive models, machine learning combines computer science and statistics. Machine learning is the process of creating or employing algorithms that learn from past data. The more information we supply, the better our performance will be.
If a system can enhance its performance by gaining new data, it has the potential to learn.
Steps
There are Seven Steps of Machine Learning
- Gathering Data
- Preparing that data
- Choosing a model
- Training
- Evaluation
- Hyperparameter Tuning
- Prediction
Features of ML
● Data is used by machine learning to find distinct patterns in a dataset.
● It can learn from previous data and improve on its own.
● It is a technology that is based on data.
● Data mining and machine learning are very similar in that they both deal with large amounts of data.
Key takeaway:
Machine Learning is an Application of Artificial Intelligence (AI) that gives machines the opportunity to learn from their experiences and develop themselves without doing any coding.
On the research-side of things, machine learning can be seen through the prism of theoretical and mathematical simulation of how this method works.
Learning is a process, whereas knowledge is the result, consequence, output, or effect of learning through experience, study, education, or training data.
"The process of obtaining new understanding, knowledge, behaviors, abilities, beliefs, attitudes, and preferences is known as learning."
Learning is a system/method/algorithm through which you acquire information from your parents, a peer, a street, a school or college or an institution, a book, or the internet. So learning is the process of acquiring skill or knowledge by trial and error in life or through study, observation, instruction, and other means.
Facts, information, data, truths, principles, beliefs, and harmful behaviors are all learned.
What we learn and learn to know is what we know.
Scientific activities such as theoretical, experimental, computational, or data analytic activities drive human and machine knowledge and learning. Science is the sum total of all known facts about reality, people, and technology. And only at the transdisciplinary level, where all barriers between and within knowledge areas and technical domains are transgressed by holistic, systematic, and transdisciplinary learning and knowledge, as shown above, could real-world learning and knowledge be achieved.
Once again, human knowledge is highly specialized and fragmented into small disciplines and fields, impeding our ability to address global change issues like as climate change, pandemics, and other planetary hazards and threats.
There is only one type of causal learning. However, in behavioristic psychology, learning is classified as either passive or active, non-associative, associative, or active (the process by which a person or animal or machine learns an association between two stimuli or events). Informal and formal education, e-learning, meaningful or rote learning, interactive or accidental or episodic learning, enculturation, and socialization are all factors to consider.
Learning, according to the ML/DL philosophy, is a change in behavior brought about by interacting with the environment in order to establish new patterns of reactions to stimuli/inputs, whether internal or external, conscious or subconscious, voluntary or involuntary.
All that a humanity, society, mind, or machine understands has been learned through experience or education. Intuition and experience, information and comprehension, values, attitudes and views, awareness and familiarity are all part of knowledge. Human minds, unlike robots, are capable of one-short learning, transfer learning, and relearning.
Learned behaviors could be demonstrated by humans, animals, plants, and machines.
When the program does not learn from advice, it can learn by generalizing from its own experiences.
● Learning by parameter adjustment
● Learning with macro-operators
● Learning by chunking
● The unity problem
Learning by parameter adjustment
● The learning system in this example uses an evaluation technique that combines data from various sources into a single static summary.
● For example, demand and production capacity might be combined into a single score to indicate the likelihood of increased output.
● However, defining how much weight each component should be given a priori is difficult.
● By guessing the correct settings and then letting the computer modify them based on its experience, the correct weight can be discovered.
● Features that appear to be strong indicators of overall performance will have their weights increased, while those that do not will have their weights reduced.
● This type of learning strategy is useful when there is a shortage of knowledge.
● In game programming, for example, factors such as piece advantage and mobility are combined into a single score to assess whether a particular board position is desired. This single score is nothing more than data that the algorithm has gathered through calculation.
● This is accomplished via programs' static evaluation functions, which combine several elements into a single score. This function's polynomial form is as follows:
● The t words represent the values of the traits that contribute to the evaluation. The c terms are the coefficients or weights that are allocated to each of these values.
● When it comes to creating programs, deciding on the right value to give each weight might be tricky. As a result, the most important rule in parameter adjustment is to:
● Begin by making an educated guess at the appropriate weight values.
● Adjust the program's weight based on your previous experience.
● Traits that appear to be good forecasters will have their weights increased, while features that look to be bad predictions will have their weights reduced.
● The following are significant factors that affect performance:
● When should the value of a coefficient be increased and when should it be decreased?
● The coefficients of good predictors should be increased, while the coefficients of undesirable predictors should be reduced.
● The difficulty of properly assigning blame to each of the phases that led to a single outcome is referred to as the credit assignment system.
● How much should the price change?
● Hill climbing is a type of learning technique.
● This strategy is particularly beneficial in situations when new knowledge is scarce or in programs where it is used in conjunction with more knowledge-intensive strategies.
Learning with Macro-Operators
● Macro-operators are collections of actions that can be handled together.
● The learning component saves the computed plan as a macro-operator once a problem is solved.
● The preconditions are the initial conditions of the just-solved problem, while the postconditions are the problem's outcomes.
● The problem solver makes good use of prior experience-based knowledge.
● By generalizing macro-operations, the issue solver can even tackle other challenges. To achieve generality, all constants in the macro-operators are substituted with variables.
● Despite the fact that the macro operator's multiple operators cause countless undesirable local changes, it can have a minimal global influence.
● Macro operators can help us develop domain-specific knowledge.
Learning by Chunking
● It's similar to chunking to learn with macro-operators. Problem-solving systems that use production systems are most likely to use it.
● A series of rules in the form of if-then statements makes up a production system. That is, what actions should be made in a specific situation. Bring an umbrella if it's raining, for example.
● Problem solvers employ rules to solve difficulties. Some of these rules may be more valuable than others, and the results are grouped together.
● Chunking can be used to gain a general understanding of search control.
● Chunks learned at the start of the problem-solving process can be employed at a later stage. The chunk is saved by the system to be used in future challenges.
The utility problem
● When knowledge is acquired in the hopes of improving a system's performance, it actually causes harm. In learning systems, this is referred to as the utility problem.
● The problem can be encountered in a wide range of AI systems, but it's particularly common in speedup learning. Speedup learning approaches are aimed to teach people control principles in order to assist them enhance their problem-solving skills. When these systems are given complete freedom to learn, they usually display the unwelcome attribute of actually slowing down.
● Although each control rule has a positive utility (improve performance), when combined, they have a negative utility (reduce performance) (degrade performance).
● The serial nature of modern hardware is one of the sources of the utility problem. The more control rules a system acquires, the longer it takes for the system to test them on each cycle.
● This type of utility analysis can be used to a wide range of learning challenges.
● Each control rule in the PRODIGY program has a utility measure. This metric considers the rule's average savings, the frequency with which it is used, and the cost of matching it.
● A proposed regulation is discarded or forgotten if it provides a negative utility.
Induction learning
Learning through Induction We have a collection of xi, f (xi) for 1in in supervised learning, and our goal is to determine 'f' using an adaptive approach. It's a machine learning method for inferring rules from facts or data. In logic, conditional or antecedent reasoning is the process of reasoning from the specific to the general. Theoretical studies in machine learning mostly concern supervised learning, a sort of inductive learning. An algorithm is provided with samples that are labeled in a useful way in supervised learning.
Inductive learning techniques, such as artificial neural networks, allow the real robot to learn simply from data that has already been collected. Another way is to let the bot learn everything in his environment by inducing facts from it. Inductive learning is the term for this type of learning. Finally, you may train the bot to evolve across numerous generations and improve its performance.
f(x) is the target function
An example is a pair [x, f(x)]
Some practical examples of induction are:
● Credit risk assessment.
● The x is the property of the customer.
● The f(x) is credit approved or not.
● Disease diagnosis.
● The x are the properties of the patient.
● The f(x) is the disease they suffer from.
● Face recognition.
● The x are bitmaps of peoples faces.
● The f(x) is to assign a name to the face.
● Automatic steering.
● The x are bitmap images from a camera in front of the car.
● The f(x) is the degree the steering wheel should be turned.
Two perspectives on inductive learning:
● Learning is the removal of uncertainty. Data removes some of the uncertainty. We are removing more uncertainty by selecting a class of hypotheses.
● Learning is guessing a good and small hypothesis class. It necessitates speculation. Because we don't know the answer, we'll have to rely on trial and error. You don't need to learn if you're confident in your domain knowledge. But we're not making educated guesses.
In the fields of statistics and machine learning, probabilistic models—in which unobserved variables are seen as stochastic and dependencies between variables are recorded in joint probability distributions—are commonly utilized.
Many desirable properties of probabilistic models include the ability to reason about the uncertainties inherent in most data, the ability to build complex models from simple parts, a natural safeguard against overfitting, and the ability to infer fully coherent inferences over complex structures from data.
Probabilistic Models in Machine Learning is the application of statistical coding to data analysis. It was one of the first machine learning methods. To this day, it's still widely used. The Naive Bayes algorithm is one of the most well-known algorithms in this group.
Probabilistic modeling provides a framework for adopting the concept of learning. The probabilistic framework specifies how to express and deploy model reservations. In scientific data analysis, predictions play a significant role. Machine learning, automation, cognitive computing, and artificial intelligence all rely heavily on them.
The use of probabilistic models to define the world is portrayed as a common idiom. These were explained using random variables, such as building pieces that were thought to be connected by probabilistic linkages.
In machine learning, there are probabilistic and non-probabilistic models. Basic knowledge of probability concepts, such as random variables and probability distributions, would be beneficial in gaining a thorough understanding of probabilistic models.
Intelligent systems must be able to derive representations from noisy or ambiguous data. Bayes' theorem, in particular, is useful in probability theory as a systematic framework for mixing prior knowledge and empirical evidence.
Key takeaway
Probabilistic Models in Machine Learning is the application of statistical coding to data analysis. It was one of the first machine learning methods. To this day, it's still widely used.
Expert systems are computer programs that are designed to tackle complicated issues in a certain domain at a level of human intellect and expertise.
An expert system is a piece of software that can deal with difficult problems and make choices in the same way as a human expert can. It achieves it by using reasoning and inference techniques to retrieve knowledge from its knowledge base based on the user's requests.
The performance of an expert system is based on the knowledge stored in its knowledge base by the expert. The better the system functions, the more knowledge is saved in the KB. When typing in the Google search box, one of the most common examples of an ES is a suggestion of spelling problems.
An expert system is a computer simulation of a human expert. It can alternatively be described as a computer software that imitates the judgment and conduct of a human or an organization with substantial knowledge and experience in a certain field.
In such a system, a knowledge base containing accumulated experience and a set of rules for applying the knowledge base to each specific case presented to the program are usually provided. Expert systems also leverage human knowledge to solve problems that would normally necessitate human intelligence. These expert systems use computers to transmit expertise knowledge as data or rules.
The expert system is a type of AI, and the first ES was created in 1970, making it the first successful artificial intelligence approach. As an expert, it solves the most difficult problems by extracting knowledge from its knowledge base. Like a human expert, the system assists in decision making for complex problems by employing both facts and heuristics. It is so named because it comprises expert knowledge of a certain subject and is capable of solving any complex problem in that domain. These systems are tailored to a particular field, such as medicine or science.
An expert system's performance is determined on the knowledge stored in its knowledge base by the expert. The more knowledge that is stored in the KB, the better the system performs. When typing in the Google search box, one of the most common examples of an ES is a suggestion of spelling problems.
The following is a block diagram that depicts how an expert system works:
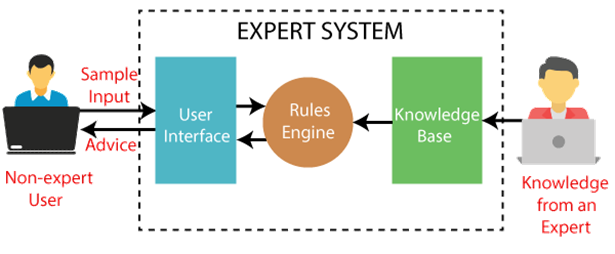
Fig 1: Expert system
Characteristics of Expert System
● High Efficiency and Accuracy - The expert system provides high efficiency and accuracy when addressing any type of complex problem in a specified domain.
● Understandable - It replies in a way that the user can easily comprehend. It can accept human-language input and produce human-language output.
● Reliable - It is really dependable in terms of producing an efficient and accurate result.
● Highly responsive - ES returns the answer to any sophisticated query in a matter of seconds.
Key takeaway
Expert systems are computer programs that are designed to tackle complicated issues in a certain domain at a level of human intellect and expertise.
An expert system is a piece of software that can deal with difficult problems and make choices in the same way as a human expert can.
Inference is used in First-Order Logic to generate new facts or sentences from current ones. It's crucial to understand some basic FOL terms before diving into the FOL inference rule.
Substitution:
Substitution is a fundamental approach for modifying phrases and formulations. All first-order logic inference systems contain it. The substitution becomes more difficult when there are quantifiers in FOL. We refer to the replacement of a constant "a" for the variable "x" when we write F[a/x].
Equality:
Atomic sentences are generated in First-Order Logic not only through the employment of predicate and words, but also through the application of equality. We can do this by using equality symbols, which indicate that the two terms relate to the same thing.
FOL inference rules for quantifier:
Because inference rules in first-order logic are comparable to those in propositional logic, below are some basic inference rules in FOL:
● Universal Generalization
● Universal Instantiation
● Existential Instantiation
● Existential introduction
Universal Generalization:
- Universal generalization is a valid inference rule that states that if premise P(c) is true for any arbitrary element c in the universe of discourse, we can arrive at the conclusion x P. (x).
- It can be represented as:

- If we want to prove that every element has a similar property, we can apply this rule.
- x must not be used as a free variable in this rule.
Example: Let's represent, P(c): "A byte contains 8 bits", so for ∀ x P(x) "All bytes contain 8 bits.", it will also be true.
Universal Instantiation:
- Universal instantiation, often known as universal elimination or UI, is a valid inference rule. It can be used numerous times to add more sentences.
- The new knowledge base is logically equivalent to the previous one.
- We can infer any phrase by replacing a ground word for the variable, according to the UI.
- According to the UI rule, any phrase P(c) can be inferred by substituting a ground term c (a constant inside domain x) for any object in the universe of discourse in x P(x).
- It can be represented as:

Example: IF "Every person like ice-cream"=> ∀x P(x) so we can infer that
"John likes ice-cream" => P(c)
Existential Instantiation:
- Existential Elimination, also known as Existential Instantiation, is a valid first-order logic inference rule.
- It can only be used once to substitute for the existential sentence.
- Despite the fact that the new KB is not conceptually identical to the previous KB, it will suffice if the old KB was.
- This rule states that one can infer P(c) from the formula given in the form of ∃x P(x) for a new constant symbol c.
- The only constraint with this rule is that c must be a new word for which P(c) is true.
- It can be represented as:

Existential introduction
- An existential generalization, also known as an existential introduction, is a valid inference rule in first-order logic.
- If some element c in the world of discourse has the characteristic P, we can infer that something else in the universe has the attribute P, according to this rule.
- It can be represented as:

Example: Let's say that,
"Pritisha got good marks in English."
"Therefore, someone got good marks in English."
Propositional vs. First-Order Inference
Previously, inference in first order logic was checked via propositionalization, which is the act of turning the Knowledge Base included in first order logic into propositional logic and then utilizing any of the propositional logic inference mechanisms to check inference.
Inference rules for quantifiers:
To get sentences without quantifiers, several inference techniques can be applied to sentences containing quantifiers. We'll be able to make the conversion if we follow these requirements.
Universal Instantiation (UI):
The rule states that by substituting a ground word (a term without variables) for the variable, we can deduce any sentence. Let SUBST () stand for the outcome of the substitution on the sentence a. The rule is then written.
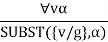
For any v and g ground term combinations. For example, in the knowledge base, there is a statement that states that all greedy rulers are Evil.
x King(x)A Greedy(x) Evil(x)
For the variable x, with the substitutions like {x/John}, {x/Richard} the following sentences can be inferred
King(John) A Greedy(John) Evil(John)
King(Richard) A Greedy(Richard) Evil(Richard)
As a result, the set of all potential instantiations can be used to substitute a globally quantified phrase.
Existential Instantiation (EI):
The existential statement states that there is some object that fulfills a requirement, and that the instantiation process is simply giving that object a name that does not already belong to another object. A Skolem constant is the moniker given to this new name. Existential Instantiation is a subset of a broader process known as "skolemization."
If the statement a, variable v, and constant symbol k do not occur anywhere else in the knowledge base,
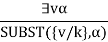
For example, from the sentence
3x Crown(x)A OnHead(x, John)
So we can infer the sentence
Crown(C1)A OnHead(C1, John)
As long as C1 does not appear elsewhere in the knowledge base. Thus, an existentially quantified sentence can be replaced by one instantiation
Elimination of Universal and Existential quantifiers should give new knowledge base which can be shown to be inferentially equivalent to old in the sense that it is satisfiable exactly when the original knowledge base is satisfiable.
Key takeaway
In First-Order Logic, inference is used to derive new facts or sentences from existing ones.
In First-Order Logic, atomic sentences are formed not only via the use of predicate and words, but also via the application of equality.
There are some Inference rules that can be applied to sentences with quantifiers to obtain sentences without quantifiers.
Knowledge acquisition is the process of gathering or collecting information from a variety of sources. It refers to the process of adding new information to a knowledge base while simultaneously refining or upgrading current information. Acquisition is the process of increasing a system's capabilities or improving its performance at a certain activity.
As a result, it is the creation and refinement of knowledge toward a certain goal. Acquired knowledge contains facts, rules, concepts, procedures, heuristics, formulas, correlations, statistics, and any other important information. Experts in the field, text books, technical papers, database reports, periodicals, and the environment are all possible sources of this knowledge.
Knowledge acquisition is a continuous process that takes place throughout a person's life. Knowledge acquisition is exemplified via machine learning. It could be a method of self-study or refining aided by computer programs. The newly acquired knowledge should be meaningfully linked to previously acquired knowledge.
The data should be accurate, non-redundant, consistent, and thorough. Knowledge acquisition helps with tasks like knowledge entry and knowledge base upkeep. The knowledge acquisition process builds dynamic data structures in order to refine current information.
The knowledge engineer's role is equally important in the development of knowledge refinements. Knowledge engineers are professionals who elicit knowledge from specialists. They write and edit code, operate several interactive tools, and create a knowledge base, among other things, to combine knowledge from multiple sources.
Fig 2: Knowledge Engineer’s Roles in Interactive Knowledge Acquisition
Key takeaway
- The gathering or collection of knowledge from multiple sources is known as knowledge acquisition.
- Knowledge acquisition is an ongoing process that spans a person's entire life.
- Machine learning is an example of knowledge acquisition.
A knowledge-based system (KBS) is a computer programme that collects and applies information from various sources. Artificial intelligence aids in the solution of problems, particularly difficult challenges, with a KBS. These systems are largely used to assist humans in making decisions, learning, and doing other tasks.
A knowledge-based system is a popular artificial intelligence application. These systems are capable of making decisions based on the data and information in their databases. They can also understand the context of the data being processed.
An interface engine plus a knowledge base make up a knowledge-based system. The interface engine serves as a search engine, while the knowledge base serves as a knowledge repository. Learning is an important part of any knowledge-based system, and learning simulation helps to enhance it over time. Expert systems, intelligent tutoring systems, hypertext manipulation systems, CASE-based systems, and databases with an intelligent user interface are all examples of knowledge-based systems.
The following are examples of knowledge-based systems:
● Medical diagnosis systems - There are systems that can assist with disease diagnosis, such as Mycin, one of the earliest KBS. Such algorithms can identify likely diagnoses (and include a confidence level around the diagnosis) as well as make therapy suggestions by inputting data or answering a series of questions.
● Eligibility analysis systems - It is possible to determine whether a person is eligible for a particular service by answering guided questions. When determining eligibility, a system would ask questions until it received an answer that was processed as disqualifying, and then transmit that information to the individual, all without the requirement for a third-party person's involvement.
● Blackboard systems - A blackboard system, in contrast to other KBS, is highly reliant on updates from human specialists. A varied collection of people will collaborate to discover the best answer to an issue. Each individual will update the blackboard with a partial solution as they work on the problem until it is eventually solved.
● Classification systems - KBS can also be used to examine various pieces of information in order to determine their classification status. For example, by studying mass spectra and chemical components, information might be inputted to determine distinct chemical compounds.
Key takeaway
A knowledge-based system (KBS) is a computer programme that collects and applies information from various sources. Artificial intelligence aids in the solution of problems, particularly difficult challenges, with a KBS.
Natural Language Processing (NLP) is an artificial intelligence (AI) way of communicating with intelligent computers using natural language such as English.
Natural language processing is essential when you want an intelligent system, such as a robot, to follow your commands, when you want to hear a decision from a dialogue-based clinical expert system, and so on.
Computational linguistics—rule-based human language modeling—is combined with statistical, machine learning, and deep learning models in NLP. These technologies, when used together, allow computers to process human language in the form of text or speech data and 'understand' its full meaning, including the speaker's or writer's intent and sentiment.
NLP is used to power computer programmes that translate text from one language to another, respond to spoken commands, and quickly summarise vast amounts of material—even in real time. Voice-activated GPS systems, digital assistants, speech-to-text dictation software, customer care chatbots, and other consumer conveniences are all examples of NLP in action. However, NLP is increasingly being used in corporate solutions to help businesses streamline operations, boost employee productivity, and simplify mission-critical business processes.
An NLP system's input and output can be anything -
● Speech
● Written Text
Components of NLP
As stated previously, NLP has two components. −
Natural Language Understanding (NLU)
The following tasks are required for comprehension:
● Translating natural language input into meaningful representations.
● Examining many features of the language.
Natural Language Generation (NLG)
It's the process of turning some internal information into meaningful words and sentences in natural language.
It involves −
● Text planning − It comprises obtaining appropriate content from the knowledge base as part of the text planning process.
● Sentence planning − Sentence planning entails selecting necessary words, generating meaningful phrases, and determining the tone of the sentence.
● Text Realization − It is the mapping of a sentence plan into a sentence structure that is known as text realization.
The NLU is more difficult than the NLG.
NLP Terminology
● Phonology - It is the systematic organization of sounds.
● Morphology - It is the study of how words are constructed from simple meaningful units.
● Morpheme − In a language, a morphe is a basic unit of meaning.
● Syntax - It is the process of putting words together to form a sentence. It also entails figuring out what structural role words have in sentences and phrases.
● Semantics - It is the study of the meaning of words and how to put them together to form meaningful phrases and sentences.
● Pragmatics - It is the study of how to use and understand sentences in various settings, as well as how this affects the sentence's meaning.
● Discourse - It is concerned with how the prior sentence influences the interpretation of the following sentence.
● World Knowledge − The term "world knowledge" refers to general knowledge about the world.
NLP Tasks
Because human language is riddled with ambiguities, writing software that accurately interprets the intended meaning of text or voice input is extremely challenging. Homonyms, homophones, sarcasm, idioms, metaphors, grammar and usage exceptions, sentence structure variations—these are just a few of the irregularities in human language that take humans years to learn, but that programmers must teach natural language-driven applications to recognise and understand accurately from the start if those applications are to be useful.
Several NLP activities help the machine understand what it's absorbing by breaking down human text and speech input in ways that the computer can understand. The following are some of these responsibilities:
● Speech recognition, The task of consistently turning voice data into text data is known as speech recognition, or speech-to-text. Any programme that follows voice commands or responds to spoken questions requires speech recognition. The way individuals speak—quickly, slurring words together, with varied emphasis and intonation, in diverse dialects, and frequently using improper grammar—makes speech recognition particularly difficult.
● Part of speech tagging, The method of determining the part of speech of a particular word or piece of text based on its use and context is known as part of speech tagging, or grammatical tagging. 'Make' is a verb in 'I can make a paper aircraft,' and a noun in 'What make of car do you own?' according to part of speech.
● Natural language generation is the job of converting structured data into human language; it is frequently referred to as the polar opposite of voice recognition or speech-to-text.
● Word sense disambiguation is the process of determining the meaning of a word with several meanings using a semantic analysis procedure to discover which word makes the most sense in the current context. The meaning of the verb 'make' in 'make the grade' (achieve) vs.'make a bet', for example, can be distinguished via word sense disambiguation (place).
● NEM (named entity recognition) is a technique for recognising words or sentences as useful entities. 'Kentucky' is a region, and 'Fred' is a man's name, according to NEM.
References:
- E.Rich and K. Knight, Artificial Intelligence, Tata McGraw Hill, 2008.
- Artificial intelligence and soft computing for beginners by Anandita Das Bhattachargee, Shroff Publishers
- Artificial Intelligence – A Practical Approach: Patterson , Tata McGraw Hill, 3rd Edition
Unit - 5
Learning
Changes in the system that allow it to perform the same task more efficiently the next time around. Learning is the process of creating or changing representations of what one is experiencing. Learning causes beneficial mental changes. Learning enhances comprehension and productivity.
Find out about new objects or structures that you didn't know about before (data mining, scientific discovery). Fill in any observations or requirements regarding a domain that are skeletal or incomplete (this expands the domain of expertise and lessens the brittleness of the system). Create software agents that can learn from their users and other software agents. Replicate a key feature of intelligent behavior.
Arthur Samuel, an American pioneer in the fields of computer games and artificial intelligence, invented the phrase Machine Learning in 1959, stating that "it offers computers the ability to learn without being expressly taught."
"A computer programme is said to learn from experience E with respect to some task T and some performance measure P, if its performance on T, as measured by P, increases with experience E," Tom Mitchell wrote in 1997.
At a high-level, machine learning is simply the study of teaching a computer programme or algorithm how to gradually improve upon a set task that it is given. On the research-side of things, machine learning can be seen through the prism of theoretical and mathematical simulation of how this method works. However, more technically it is the study of how to construct applications that exhibit this iterative progress.
Machine Learning is an Application of Artificial Intelligence (AI) that gives machines the opportunity to learn from their experiences and develop themselves without doing any coding.
Machine Learning is a branch of Artificial Intelligence. Machine Learning is the study of making machines more human-like in their actions and decisions by allowing them the ability to learn and create their own programmes. This is achieved with minimal human interference, i.e., no explicit programming. The learning process is automated and enhanced based on the experiences of the machines in the process.
In the real world, we are surrounded by individuals who can learn anything from their experiences thanks to their ability to learn, and we have computers or machines that follow our commands. But, like a human, can a machine learn from past experiences or data? So here's where Machine Learning comes in.
Machine learning is a subfield of artificial intelligence that focuses on the development of algorithms that allow a computer to learn on its own from data and previous experiences. Arthur Samuel was the first to coin the term "machine learning" in 1959. In a nutshell, we can characterize it as follows:
“Machine learning enables a machine to automatically learn from data, improve performance from experiences, and predict things without being explicitly programmed.”
Machine learning algorithms create a mathematical model with the help of sample historical data, referred to as training data, that aids in making predictions or judgments without being explicitly programmed. In order to create predictive models, machine learning combines computer science and statistics. Machine learning is the process of creating or employing algorithms that learn from past data. The more information we supply, the better our performance will be.
If a system can enhance its performance by gaining new data, it has the potential to learn.
Steps
There are Seven Steps of Machine Learning
- Gathering Data
- Preparing that data
- Choosing a model
- Training
- Evaluation
- Hyperparameter Tuning
- Prediction
Features of ML
● Data is used by machine learning to find distinct patterns in a dataset.
● It can learn from previous data and improve on its own.
● It is a technology that is based on data.
● Data mining and machine learning are very similar in that they both deal with large amounts of data.
Key takeaway:
Machine Learning is an Application of Artificial Intelligence (AI) that gives machines the opportunity to learn from their experiences and develop themselves without doing any coding.
On the research-side of things, machine learning can be seen through the prism of theoretical and mathematical simulation of how this method works.
Learning is a process, whereas knowledge is the result, consequence, output, or effect of learning through experience, study, education, or training data.
"The process of obtaining new understanding, knowledge, behaviors, abilities, beliefs, attitudes, and preferences is known as learning."
Learning is a system/method/algorithm through which you acquire information from your parents, a peer, a street, a school or college or an institution, a book, or the internet. So learning is the process of acquiring skill or knowledge by trial and error in life or through study, observation, instruction, and other means.
Facts, information, data, truths, principles, beliefs, and harmful behaviors are all learned.
What we learn and learn to know is what we know.
Scientific activities such as theoretical, experimental, computational, or data analytic activities drive human and machine knowledge and learning. Science is the sum total of all known facts about reality, people, and technology. And only at the transdisciplinary level, where all barriers between and within knowledge areas and technical domains are transgressed by holistic, systematic, and transdisciplinary learning and knowledge, as shown above, could real-world learning and knowledge be achieved.
Once again, human knowledge is highly specialized and fragmented into small disciplines and fields, impeding our ability to address global change issues like as climate change, pandemics, and other planetary hazards and threats.
There is only one type of causal learning. However, in behavioristic psychology, learning is classified as either passive or active, non-associative, associative, or active (the process by which a person or animal or machine learns an association between two stimuli or events). Informal and formal education, e-learning, meaningful or rote learning, interactive or accidental or episodic learning, enculturation, and socialization are all factors to consider.
Learning, according to the ML/DL philosophy, is a change in behavior brought about by interacting with the environment in order to establish new patterns of reactions to stimuli/inputs, whether internal or external, conscious or subconscious, voluntary or involuntary.
All that a humanity, society, mind, or machine understands has been learned through experience or education. Intuition and experience, information and comprehension, values, attitudes and views, awareness and familiarity are all part of knowledge. Human minds, unlike robots, are capable of one-short learning, transfer learning, and relearning.
Learned behaviors could be demonstrated by humans, animals, plants, and machines.
When the program does not learn from advice, it can learn by generalizing from its own experiences.
● Learning by parameter adjustment
● Learning with macro-operators
● Learning by chunking
● The unity problem
Learning by parameter adjustment
● The learning system in this example uses an evaluation technique that combines data from various sources into a single static summary.
● For example, demand and production capacity might be combined into a single score to indicate the likelihood of increased output.
● However, defining how much weight each component should be given a priori is difficult.
● By guessing the correct settings and then letting the computer modify them based on its experience, the correct weight can be discovered.
● Features that appear to be strong indicators of overall performance will have their weights increased, while those that do not will have their weights reduced.
● This type of learning strategy is useful when there is a shortage of knowledge.
● In game programming, for example, factors such as piece advantage and mobility are combined into a single score to assess whether a particular board position is desired. This single score is nothing more than data that the algorithm has gathered through calculation.
● This is accomplished via programs' static evaluation functions, which combine several elements into a single score. This function's polynomial form is as follows:
● The t words represent the values of the traits that contribute to the evaluation. The c terms are the coefficients or weights that are allocated to each of these values.
● When it comes to creating programs, deciding on the right value to give each weight might be tricky. As a result, the most important rule in parameter adjustment is to:
● Begin by making an educated guess at the appropriate weight values.
● Adjust the program's weight based on your previous experience.
● Traits that appear to be good forecasters will have their weights increased, while features that look to be bad predictions will have their weights reduced.
● The following are significant factors that affect performance:
● When should the value of a coefficient be increased and when should it be decreased?
● The coefficients of good predictors should be increased, while the coefficients of undesirable predictors should be reduced.
● The difficulty of properly assigning blame to each of the phases that led to a single outcome is referred to as the credit assignment system.
● How much should the price change?
● Hill climbing is a type of learning technique.
● This strategy is particularly beneficial in situations when new knowledge is scarce or in programs where it is used in conjunction with more knowledge-intensive strategies.
Learning with Macro-Operators
● Macro-operators are collections of actions that can be handled together.
● The learning component saves the computed plan as a macro-operator once a problem is solved.
● The preconditions are the initial conditions of the just-solved problem, while the postconditions are the problem's outcomes.
● The problem solver makes good use of prior experience-based knowledge.
● By generalizing macro-operations, the issue solver can even tackle other challenges. To achieve generality, all constants in the macro-operators are substituted with variables.
● Despite the fact that the macro operator's multiple operators cause countless undesirable local changes, it can have a minimal global influence.
● Macro operators can help us develop domain-specific knowledge.
Learning by Chunking
● It's similar to chunking to learn with macro-operators. Problem-solving systems that use production systems are most likely to use it.
● A series of rules in the form of if-then statements makes up a production system. That is, what actions should be made in a specific situation. Bring an umbrella if it's raining, for example.
● Problem solvers employ rules to solve difficulties. Some of these rules may be more valuable than others, and the results are grouped together.
● Chunking can be used to gain a general understanding of search control.
● Chunks learned at the start of the problem-solving process can be employed at a later stage. The chunk is saved by the system to be used in future challenges.
The utility problem
● When knowledge is acquired in the hopes of improving a system's performance, it actually causes harm. In learning systems, this is referred to as the utility problem.
● The problem can be encountered in a wide range of AI systems, but it's particularly common in speedup learning. Speedup learning approaches are aimed to teach people control principles in order to assist them enhance their problem-solving skills. When these systems are given complete freedom to learn, they usually display the unwelcome attribute of actually slowing down.
● Although each control rule has a positive utility (improve performance), when combined, they have a negative utility (reduce performance) (degrade performance).
● The serial nature of modern hardware is one of the sources of the utility problem. The more control rules a system acquires, the longer it takes for the system to test them on each cycle.
● This type of utility analysis can be used to a wide range of learning challenges.
● Each control rule in the PRODIGY program has a utility measure. This metric considers the rule's average savings, the frequency with which it is used, and the cost of matching it.
● A proposed regulation is discarded or forgotten if it provides a negative utility.
Induction learning
Learning through Induction We have a collection of xi, f (xi) for 1in in supervised learning, and our goal is to determine 'f' using an adaptive approach. It's a machine learning method for inferring rules from facts or data. In logic, conditional or antecedent reasoning is the process of reasoning from the specific to the general. Theoretical studies in machine learning mostly concern supervised learning, a sort of inductive learning. An algorithm is provided with samples that are labeled in a useful way in supervised learning.
Inductive learning techniques, such as artificial neural networks, allow the real robot to learn simply from data that has already been collected. Another way is to let the bot learn everything in his environment by inducing facts from it. Inductive learning is the term for this type of learning. Finally, you may train the bot to evolve across numerous generations and improve its performance.
f(x) is the target function
An example is a pair [x, f(x)]
Some practical examples of induction are:
● Credit risk assessment.
● The x is the property of the customer.
● The f(x) is credit approved or not.
● Disease diagnosis.
● The x are the properties of the patient.
● The f(x) is the disease they suffer from.
● Face recognition.
● The x are bitmaps of peoples faces.
● The f(x) is to assign a name to the face.
● Automatic steering.
● The x are bitmap images from a camera in front of the car.
● The f(x) is the degree the steering wheel should be turned.
Two perspectives on inductive learning:
● Learning is the removal of uncertainty. Data removes some of the uncertainty. We are removing more uncertainty by selecting a class of hypotheses.
● Learning is guessing a good and small hypothesis class. It necessitates speculation. Because we don't know the answer, we'll have to rely on trial and error. You don't need to learn if you're confident in your domain knowledge. But we're not making educated guesses.
In the fields of statistics and machine learning, probabilistic models—in which unobserved variables are seen as stochastic and dependencies between variables are recorded in joint probability distributions—are commonly utilized.
Many desirable properties of probabilistic models include the ability to reason about the uncertainties inherent in most data, the ability to build complex models from simple parts, a natural safeguard against overfitting, and the ability to infer fully coherent inferences over complex structures from data.
Probabilistic Models in Machine Learning is the application of statistical coding to data analysis. It was one of the first machine learning methods. To this day, it's still widely used. The Naive Bayes algorithm is one of the most well-known algorithms in this group.
Probabilistic modeling provides a framework for adopting the concept of learning. The probabilistic framework specifies how to express and deploy model reservations. In scientific data analysis, predictions play a significant role. Machine learning, automation, cognitive computing, and artificial intelligence all rely heavily on them.
The use of probabilistic models to define the world is portrayed as a common idiom. These were explained using random variables, such as building pieces that were thought to be connected by probabilistic linkages.
In machine learning, there are probabilistic and non-probabilistic models. Basic knowledge of probability concepts, such as random variables and probability distributions, would be beneficial in gaining a thorough understanding of probabilistic models.
Intelligent systems must be able to derive representations from noisy or ambiguous data. Bayes' theorem, in particular, is useful in probability theory as a systematic framework for mixing prior knowledge and empirical evidence.
Key takeaway
Probabilistic Models in Machine Learning is the application of statistical coding to data analysis. It was one of the first machine learning methods. To this day, it's still widely used.
Expert systems are computer programs that are designed to tackle complicated issues in a certain domain at a level of human intellect and expertise.
An expert system is a piece of software that can deal with difficult problems and make choices in the same way as a human expert can. It achieves it by using reasoning and inference techniques to retrieve knowledge from its knowledge base based on the user's requests.
The performance of an expert system is based on the knowledge stored in its knowledge base by the expert. The better the system functions, the more knowledge is saved in the KB. When typing in the Google search box, one of the most common examples of an ES is a suggestion of spelling problems.
An expert system is a computer simulation of a human expert. It can alternatively be described as a computer software that imitates the judgment and conduct of a human or an organization with substantial knowledge and experience in a certain field.
In such a system, a knowledge base containing accumulated experience and a set of rules for applying the knowledge base to each specific case presented to the program are usually provided. Expert systems also leverage human knowledge to solve problems that would normally necessitate human intelligence. These expert systems use computers to transmit expertise knowledge as data or rules.
The expert system is a type of AI, and the first ES was created in 1970, making it the first successful artificial intelligence approach. As an expert, it solves the most difficult problems by extracting knowledge from its knowledge base. Like a human expert, the system assists in decision making for complex problems by employing both facts and heuristics. It is so named because it comprises expert knowledge of a certain subject and is capable of solving any complex problem in that domain. These systems are tailored to a particular field, such as medicine or science.
An expert system's performance is determined on the knowledge stored in its knowledge base by the expert. The more knowledge that is stored in the KB, the better the system performs. When typing in the Google search box, one of the most common examples of an ES is a suggestion of spelling problems.
The following is a block diagram that depicts how an expert system works:
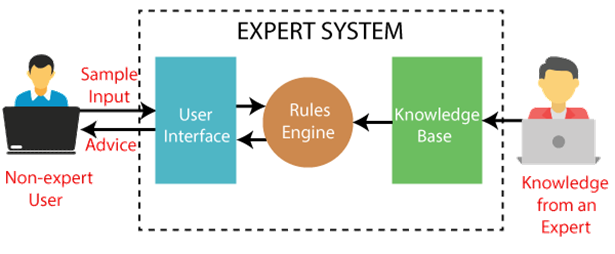
Fig 1: Expert system
Characteristics of Expert System
● High Efficiency and Accuracy - The expert system provides high efficiency and accuracy when addressing any type of complex problem in a specified domain.
● Understandable - It replies in a way that the user can easily comprehend. It can accept human-language input and produce human-language output.
● Reliable - It is really dependable in terms of producing an efficient and accurate result.
● Highly responsive - ES returns the answer to any sophisticated query in a matter of seconds.
Key takeaway
Expert systems are computer programs that are designed to tackle complicated issues in a certain domain at a level of human intellect and expertise.
An expert system is a piece of software that can deal with difficult problems and make choices in the same way as a human expert can.
Inference is used in First-Order Logic to generate new facts or sentences from current ones. It's crucial to understand some basic FOL terms before diving into the FOL inference rule.
Substitution:
Substitution is a fundamental approach for modifying phrases and formulations. All first-order logic inference systems contain it. The substitution becomes more difficult when there are quantifiers in FOL. We refer to the replacement of a constant "a" for the variable "x" when we write F[a/x].
Equality:
Atomic sentences are generated in First-Order Logic not only through the employment of predicate and words, but also through the application of equality. We can do this by using equality symbols, which indicate that the two terms relate to the same thing.
FOL inference rules for quantifier:
Because inference rules in first-order logic are comparable to those in propositional logic, below are some basic inference rules in FOL:
● Universal Generalization
● Universal Instantiation
● Existential Instantiation
● Existential introduction
Universal Generalization:
- Universal generalization is a valid inference rule that states that if premise P(c) is true for any arbitrary element c in the universe of discourse, we can arrive at the conclusion x P. (x).
- It can be represented as:

- If we want to prove that every element has a similar property, we can apply this rule.
- x must not be used as a free variable in this rule.
Example: Let's represent, P(c): "A byte contains 8 bits", so for ∀ x P(x) "All bytes contain 8 bits.", it will also be true.
Universal Instantiation:
- Universal instantiation, often known as universal elimination or UI, is a valid inference rule. It can be used numerous times to add more sentences.
- The new knowledge base is logically equivalent to the previous one.
- We can infer any phrase by replacing a ground word for the variable, according to the UI.
- According to the UI rule, any phrase P(c) can be inferred by substituting a ground term c (a constant inside domain x) for any object in the universe of discourse in x P(x).
- It can be represented as:

Example: IF "Every person like ice-cream"=> ∀x P(x) so we can infer that
"John likes ice-cream" => P(c)
Existential Instantiation:
- Existential Elimination, also known as Existential Instantiation, is a valid first-order logic inference rule.
- It can only be used once to substitute for the existential sentence.
- Despite the fact that the new KB is not conceptually identical to the previous KB, it will suffice if the old KB was.
- This rule states that one can infer P(c) from the formula given in the form of ∃x P(x) for a new constant symbol c.
- The only constraint with this rule is that c must be a new word for which P(c) is true.
- It can be represented as:

Existential introduction
- An existential generalization, also known as an existential introduction, is a valid inference rule in first-order logic.
- If some element c in the world of discourse has the characteristic P, we can infer that something else in the universe has the attribute P, according to this rule.
- It can be represented as:

Example: Let's say that,
"Pritisha got good marks in English."
"Therefore, someone got good marks in English."
Propositional vs. First-Order Inference
Previously, inference in first order logic was checked via propositionalization, which is the act of turning the Knowledge Base included in first order logic into propositional logic and then utilizing any of the propositional logic inference mechanisms to check inference.
Inference rules for quantifiers:
To get sentences without quantifiers, several inference techniques can be applied to sentences containing quantifiers. We'll be able to make the conversion if we follow these requirements.
Universal Instantiation (UI):
The rule states that by substituting a ground word (a term without variables) for the variable, we can deduce any sentence. Let SUBST () stand for the outcome of the substitution on the sentence a. The rule is then written.
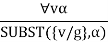
For any v and g ground term combinations. For example, in the knowledge base, there is a statement that states that all greedy rulers are Evil.
x King(x)A Greedy(x) Evil(x)
For the variable x, with the substitutions like {x/John}, {x/Richard} the following sentences can be inferred
King(John) A Greedy(John) Evil(John)
King(Richard) A Greedy(Richard) Evil(Richard)
As a result, the set of all potential instantiations can be used to substitute a globally quantified phrase.
Existential Instantiation (EI):
The existential statement states that there is some object that fulfills a requirement, and that the instantiation process is simply giving that object a name that does not already belong to another object. A Skolem constant is the moniker given to this new name. Existential Instantiation is a subset of a broader process known as "skolemization."
If the statement a, variable v, and constant symbol k do not occur anywhere else in the knowledge base,
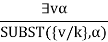
For example, from the sentence
3x Crown(x)A OnHead(x, John)
So we can infer the sentence
Crown(C1)A OnHead(C1, John)
As long as C1 does not appear elsewhere in the knowledge base. Thus, an existentially quantified sentence can be replaced by one instantiation
Elimination of Universal and Existential quantifiers should give new knowledge base which can be shown to be inferentially equivalent to old in the sense that it is satisfiable exactly when the original knowledge base is satisfiable.
Key takeaway
In First-Order Logic, inference is used to derive new facts or sentences from existing ones.
In First-Order Logic, atomic sentences are formed not only via the use of predicate and words, but also via the application of equality.
There are some Inference rules that can be applied to sentences with quantifiers to obtain sentences without quantifiers.
Knowledge acquisition is the process of gathering or collecting information from a variety of sources. It refers to the process of adding new information to a knowledge base while simultaneously refining or upgrading current information. Acquisition is the process of increasing a system's capabilities or improving its performance at a certain activity.
As a result, it is the creation and refinement of knowledge toward a certain goal. Acquired knowledge contains facts, rules, concepts, procedures, heuristics, formulas, correlations, statistics, and any other important information. Experts in the field, text books, technical papers, database reports, periodicals, and the environment are all possible sources of this knowledge.
Knowledge acquisition is a continuous process that takes place throughout a person's life. Knowledge acquisition is exemplified via machine learning. It could be a method of self-study or refining aided by computer programs. The newly acquired knowledge should be meaningfully linked to previously acquired knowledge.
The data should be accurate, non-redundant, consistent, and thorough. Knowledge acquisition helps with tasks like knowledge entry and knowledge base upkeep. The knowledge acquisition process builds dynamic data structures in order to refine current information.
The knowledge engineer's role is equally important in the development of knowledge refinements. Knowledge engineers are professionals who elicit knowledge from specialists. They write and edit code, operate several interactive tools, and create a knowledge base, among other things, to combine knowledge from multiple sources.
Fig 2: Knowledge Engineer’s Roles in Interactive Knowledge Acquisition
Key takeaway
- The gathering or collection of knowledge from multiple sources is known as knowledge acquisition.
- Knowledge acquisition is an ongoing process that spans a person's entire life.
- Machine learning is an example of knowledge acquisition.
A knowledge-based system (KBS) is a computer programme that collects and applies information from various sources. Artificial intelligence aids in the solution of problems, particularly difficult challenges, with a KBS. These systems are largely used to assist humans in making decisions, learning, and doing other tasks.
A knowledge-based system is a popular artificial intelligence application. These systems are capable of making decisions based on the data and information in their databases. They can also understand the context of the data being processed.
An interface engine plus a knowledge base make up a knowledge-based system. The interface engine serves as a search engine, while the knowledge base serves as a knowledge repository. Learning is an important part of any knowledge-based system, and learning simulation helps to enhance it over time. Expert systems, intelligent tutoring systems, hypertext manipulation systems, CASE-based systems, and databases with an intelligent user interface are all examples of knowledge-based systems.
The following are examples of knowledge-based systems:
● Medical diagnosis systems - There are systems that can assist with disease diagnosis, such as Mycin, one of the earliest KBS. Such algorithms can identify likely diagnoses (and include a confidence level around the diagnosis) as well as make therapy suggestions by inputting data or answering a series of questions.
● Eligibility analysis systems - It is possible to determine whether a person is eligible for a particular service by answering guided questions. When determining eligibility, a system would ask questions until it received an answer that was processed as disqualifying, and then transmit that information to the individual, all without the requirement for a third-party person's involvement.
● Blackboard systems - A blackboard system, in contrast to other KBS, is highly reliant on updates from human specialists. A varied collection of people will collaborate to discover the best answer to an issue. Each individual will update the blackboard with a partial solution as they work on the problem until it is eventually solved.
● Classification systems - KBS can also be used to examine various pieces of information in order to determine their classification status. For example, by studying mass spectra and chemical components, information might be inputted to determine distinct chemical compounds.
Key takeaway
A knowledge-based system (KBS) is a computer programme that collects and applies information from various sources. Artificial intelligence aids in the solution of problems, particularly difficult challenges, with a KBS.
Natural Language Processing (NLP) is an artificial intelligence (AI) way of communicating with intelligent computers using natural language such as English.
Natural language processing is essential when you want an intelligent system, such as a robot, to follow your commands, when you want to hear a decision from a dialogue-based clinical expert system, and so on.
Computational linguistics—rule-based human language modeling—is combined with statistical, machine learning, and deep learning models in NLP. These technologies, when used together, allow computers to process human language in the form of text or speech data and 'understand' its full meaning, including the speaker's or writer's intent and sentiment.
NLP is used to power computer programmes that translate text from one language to another, respond to spoken commands, and quickly summarise vast amounts of material—even in real time. Voice-activated GPS systems, digital assistants, speech-to-text dictation software, customer care chatbots, and other consumer conveniences are all examples of NLP in action. However, NLP is increasingly being used in corporate solutions to help businesses streamline operations, boost employee productivity, and simplify mission-critical business processes.
An NLP system's input and output can be anything -
● Speech
● Written Text
Components of NLP
As stated previously, NLP has two components. −
Natural Language Understanding (NLU)
The following tasks are required for comprehension:
● Translating natural language input into meaningful representations.
● Examining many features of the language.
Natural Language Generation (NLG)
It's the process of turning some internal information into meaningful words and sentences in natural language.
It involves −
● Text planning − It comprises obtaining appropriate content from the knowledge base as part of the text planning process.
● Sentence planning − Sentence planning entails selecting necessary words, generating meaningful phrases, and determining the tone of the sentence.
● Text Realization − It is the mapping of a sentence plan into a sentence structure that is known as text realization.
The NLU is more difficult than the NLG.
NLP Terminology
● Phonology - It is the systematic organization of sounds.
● Morphology - It is the study of how words are constructed from simple meaningful units.
● Morpheme − In a language, a morphe is a basic unit of meaning.
● Syntax - It is the process of putting words together to form a sentence. It also entails figuring out what structural role words have in sentences and phrases.
● Semantics - It is the study of the meaning of words and how to put them together to form meaningful phrases and sentences.
● Pragmatics - It is the study of how to use and understand sentences in various settings, as well as how this affects the sentence's meaning.
● Discourse - It is concerned with how the prior sentence influences the interpretation of the following sentence.
● World Knowledge − The term "world knowledge" refers to general knowledge about the world.
NLP Tasks
Because human language is riddled with ambiguities, writing software that accurately interprets the intended meaning of text or voice input is extremely challenging. Homonyms, homophones, sarcasm, idioms, metaphors, grammar and usage exceptions, sentence structure variations—these are just a few of the irregularities in human language that take humans years to learn, but that programmers must teach natural language-driven applications to recognise and understand accurately from the start if those applications are to be useful.
Several NLP activities help the machine understand what it's absorbing by breaking down human text and speech input in ways that the computer can understand. The following are some of these responsibilities:
● Speech recognition, The task of consistently turning voice data into text data is known as speech recognition, or speech-to-text. Any programme that follows voice commands or responds to spoken questions requires speech recognition. The way individuals speak—quickly, slurring words together, with varied emphasis and intonation, in diverse dialects, and frequently using improper grammar—makes speech recognition particularly difficult.
● Part of speech tagging, The method of determining the part of speech of a particular word or piece of text based on its use and context is known as part of speech tagging, or grammatical tagging. 'Make' is a verb in 'I can make a paper aircraft,' and a noun in 'What make of car do you own?' according to part of speech.
● Natural language generation is the job of converting structured data into human language; it is frequently referred to as the polar opposite of voice recognition or speech-to-text.
● Word sense disambiguation is the process of determining the meaning of a word with several meanings using a semantic analysis procedure to discover which word makes the most sense in the current context. The meaning of the verb 'make' in 'make the grade' (achieve) vs.'make a bet', for example, can be distinguished via word sense disambiguation (place).
● NEM (named entity recognition) is a technique for recognising words or sentences as useful entities. 'Kentucky' is a region, and 'Fred' is a man's name, according to NEM.
References:
- E.Rich and K. Knight, Artificial Intelligence, Tata McGraw Hill, 2008.
- Artificial intelligence and soft computing for beginners by Anandita Das Bhattachargee, Shroff Publishers
- Artificial Intelligence – A Practical Approach: Patterson , Tata McGraw Hill, 3rd Edition
Unit - 5
Learning
Changes in the system that allow it to perform the same task more efficiently the next time around. Learning is the process of creating or changing representations of what one is experiencing. Learning causes beneficial mental changes. Learning enhances comprehension and productivity.
Find out about new objects or structures that you didn't know about before (data mining, scientific discovery). Fill in any observations or requirements regarding a domain that are skeletal or incomplete (this expands the domain of expertise and lessens the brittleness of the system). Create software agents that can learn from their users and other software agents. Replicate a key feature of intelligent behavior.
Arthur Samuel, an American pioneer in the fields of computer games and artificial intelligence, invented the phrase Machine Learning in 1959, stating that "it offers computers the ability to learn without being expressly taught."
"A computer programme is said to learn from experience E with respect to some task T and some performance measure P, if its performance on T, as measured by P, increases with experience E," Tom Mitchell wrote in 1997.
At a high-level, machine learning is simply the study of teaching a computer programme or algorithm how to gradually improve upon a set task that it is given. On the research-side of things, machine learning can be seen through the prism of theoretical and mathematical simulation of how this method works. However, more technically it is the study of how to construct applications that exhibit this iterative progress.
Machine Learning is an Application of Artificial Intelligence (AI) that gives machines the opportunity to learn from their experiences and develop themselves without doing any coding.
Machine Learning is a branch of Artificial Intelligence. Machine Learning is the study of making machines more human-like in their actions and decisions by allowing them the ability to learn and create their own programmes. This is achieved with minimal human interference, i.e., no explicit programming. The learning process is automated and enhanced based on the experiences of the machines in the process.
In the real world, we are surrounded by individuals who can learn anything from their experiences thanks to their ability to learn, and we have computers or machines that follow our commands. But, like a human, can a machine learn from past experiences or data? So here's where Machine Learning comes in.
Machine learning is a subfield of artificial intelligence that focuses on the development of algorithms that allow a computer to learn on its own from data and previous experiences. Arthur Samuel was the first to coin the term "machine learning" in 1959. In a nutshell, we can characterize it as follows:
“Machine learning enables a machine to automatically learn from data, improve performance from experiences, and predict things without being explicitly programmed.”
Machine learning algorithms create a mathematical model with the help of sample historical data, referred to as training data, that aids in making predictions or judgments without being explicitly programmed. In order to create predictive models, machine learning combines computer science and statistics. Machine learning is the process of creating or employing algorithms that learn from past data. The more information we supply, the better our performance will be.
If a system can enhance its performance by gaining new data, it has the potential to learn.
Steps
There are Seven Steps of Machine Learning
- Gathering Data
- Preparing that data
- Choosing a model
- Training
- Evaluation
- Hyperparameter Tuning
- Prediction
Features of ML
● Data is used by machine learning to find distinct patterns in a dataset.
● It can learn from previous data and improve on its own.
● It is a technology that is based on data.
● Data mining and machine learning are very similar in that they both deal with large amounts of data.
Key takeaway:
Machine Learning is an Application of Artificial Intelligence (AI) that gives machines the opportunity to learn from their experiences and develop themselves without doing any coding.
On the research-side of things, machine learning can be seen through the prism of theoretical and mathematical simulation of how this method works.
Learning is a process, whereas knowledge is the result, consequence, output, or effect of learning through experience, study, education, or training data.
"The process of obtaining new understanding, knowledge, behaviors, abilities, beliefs, attitudes, and preferences is known as learning."
Learning is a system/method/algorithm through which you acquire information from your parents, a peer, a street, a school or college or an institution, a book, or the internet. So learning is the process of acquiring skill or knowledge by trial and error in life or through study, observation, instruction, and other means.
Facts, information, data, truths, principles, beliefs, and harmful behaviors are all learned.
What we learn and learn to know is what we know.
Scientific activities such as theoretical, experimental, computational, or data analytic activities drive human and machine knowledge and learning. Science is the sum total of all known facts about reality, people, and technology. And only at the transdisciplinary level, where all barriers between and within knowledge areas and technical domains are transgressed by holistic, systematic, and transdisciplinary learning and knowledge, as shown above, could real-world learning and knowledge be achieved.
Once again, human knowledge is highly specialized and fragmented into small disciplines and fields, impeding our ability to address global change issues like as climate change, pandemics, and other planetary hazards and threats.
There is only one type of causal learning. However, in behavioristic psychology, learning is classified as either passive or active, non-associative, associative, or active (the process by which a person or animal or machine learns an association between two stimuli or events). Informal and formal education, e-learning, meaningful or rote learning, interactive or accidental or episodic learning, enculturation, and socialization are all factors to consider.
Learning, according to the ML/DL philosophy, is a change in behavior brought about by interacting with the environment in order to establish new patterns of reactions to stimuli/inputs, whether internal or external, conscious or subconscious, voluntary or involuntary.
All that a humanity, society, mind, or machine understands has been learned through experience or education. Intuition and experience, information and comprehension, values, attitudes and views, awareness and familiarity are all part of knowledge. Human minds, unlike robots, are capable of one-short learning, transfer learning, and relearning.
Learned behaviors could be demonstrated by humans, animals, plants, and machines.
When the program does not learn from advice, it can learn by generalizing from its own experiences.
● Learning by parameter adjustment
● Learning with macro-operators
● Learning by chunking
● The unity problem
Learning by parameter adjustment
● The learning system in this example uses an evaluation technique that combines data from various sources into a single static summary.
● For example, demand and production capacity might be combined into a single score to indicate the likelihood of increased output.
● However, defining how much weight each component should be given a priori is difficult.
● By guessing the correct settings and then letting the computer modify them based on its experience, the correct weight can be discovered.
● Features that appear to be strong indicators of overall performance will have their weights increased, while those that do not will have their weights reduced.
● This type of learning strategy is useful when there is a shortage of knowledge.
● In game programming, for example, factors such as piece advantage and mobility are combined into a single score to assess whether a particular board position is desired. This single score is nothing more than data that the algorithm has gathered through calculation.
● This is accomplished via programs' static evaluation functions, which combine several elements into a single score. This function's polynomial form is as follows:
● The t words represent the values of the traits that contribute to the evaluation. The c terms are the coefficients or weights that are allocated to each of these values.
● When it comes to creating programs, deciding on the right value to give each weight might be tricky. As a result, the most important rule in parameter adjustment is to:
● Begin by making an educated guess at the appropriate weight values.
● Adjust the program's weight based on your previous experience.
● Traits that appear to be good forecasters will have their weights increased, while features that look to be bad predictions will have their weights reduced.
● The following are significant factors that affect performance:
● When should the value of a coefficient be increased and when should it be decreased?
● The coefficients of good predictors should be increased, while the coefficients of undesirable predictors should be reduced.
● The difficulty of properly assigning blame to each of the phases that led to a single outcome is referred to as the credit assignment system.
● How much should the price change?
● Hill climbing is a type of learning technique.
● This strategy is particularly beneficial in situations when new knowledge is scarce or in programs where it is used in conjunction with more knowledge-intensive strategies.
Learning with Macro-Operators
● Macro-operators are collections of actions that can be handled together.
● The learning component saves the computed plan as a macro-operator once a problem is solved.
● The preconditions are the initial conditions of the just-solved problem, while the postconditions are the problem's outcomes.
● The problem solver makes good use of prior experience-based knowledge.
● By generalizing macro-operations, the issue solver can even tackle other challenges. To achieve generality, all constants in the macro-operators are substituted with variables.
● Despite the fact that the macro operator's multiple operators cause countless undesirable local changes, it can have a minimal global influence.
● Macro operators can help us develop domain-specific knowledge.
Learning by Chunking
● It's similar to chunking to learn with macro-operators. Problem-solving systems that use production systems are most likely to use it.
● A series of rules in the form of if-then statements makes up a production system. That is, what actions should be made in a specific situation. Bring an umbrella if it's raining, for example.
● Problem solvers employ rules to solve difficulties. Some of these rules may be more valuable than others, and the results are grouped together.
● Chunking can be used to gain a general understanding of search control.
● Chunks learned at the start of the problem-solving process can be employed at a later stage. The chunk is saved by the system to be used in future challenges.
The utility problem
● When knowledge is acquired in the hopes of improving a system's performance, it actually causes harm. In learning systems, this is referred to as the utility problem.
● The problem can be encountered in a wide range of AI systems, but it's particularly common in speedup learning. Speedup learning approaches are aimed to teach people control principles in order to assist them enhance their problem-solving skills. When these systems are given complete freedom to learn, they usually display the unwelcome attribute of actually slowing down.
● Although each control rule has a positive utility (improve performance), when combined, they have a negative utility (reduce performance) (degrade performance).
● The serial nature of modern hardware is one of the sources of the utility problem. The more control rules a system acquires, the longer it takes for the system to test them on each cycle.
● This type of utility analysis can be used to a wide range of learning challenges.
● Each control rule in the PRODIGY program has a utility measure. This metric considers the rule's average savings, the frequency with which it is used, and the cost of matching it.
● A proposed regulation is discarded or forgotten if it provides a negative utility.
Induction learning
Learning through Induction We have a collection of xi, f (xi) for 1in in supervised learning, and our goal is to determine 'f' using an adaptive approach. It's a machine learning method for inferring rules from facts or data. In logic, conditional or antecedent reasoning is the process of reasoning from the specific to the general. Theoretical studies in machine learning mostly concern supervised learning, a sort of inductive learning. An algorithm is provided with samples that are labeled in a useful way in supervised learning.
Inductive learning techniques, such as artificial neural networks, allow the real robot to learn simply from data that has already been collected. Another way is to let the bot learn everything in his environment by inducing facts from it. Inductive learning is the term for this type of learning. Finally, you may train the bot to evolve across numerous generations and improve its performance.
f(x) is the target function
An example is a pair [x, f(x)]
Some practical examples of induction are:
● Credit risk assessment.
● The x is the property of the customer.
● The f(x) is credit approved or not.
● Disease diagnosis.
● The x are the properties of the patient.
● The f(x) is the disease they suffer from.
● Face recognition.
● The x are bitmaps of peoples faces.
● The f(x) is to assign a name to the face.
● Automatic steering.
● The x are bitmap images from a camera in front of the car.
● The f(x) is the degree the steering wheel should be turned.
Two perspectives on inductive learning:
● Learning is the removal of uncertainty. Data removes some of the uncertainty. We are removing more uncertainty by selecting a class of hypotheses.
● Learning is guessing a good and small hypothesis class. It necessitates speculation. Because we don't know the answer, we'll have to rely on trial and error. You don't need to learn if you're confident in your domain knowledge. But we're not making educated guesses.
In the fields of statistics and machine learning, probabilistic models—in which unobserved variables are seen as stochastic and dependencies between variables are recorded in joint probability distributions—are commonly utilized.
Many desirable properties of probabilistic models include the ability to reason about the uncertainties inherent in most data, the ability to build complex models from simple parts, a natural safeguard against overfitting, and the ability to infer fully coherent inferences over complex structures from data.
Probabilistic Models in Machine Learning is the application of statistical coding to data analysis. It was one of the first machine learning methods. To this day, it's still widely used. The Naive Bayes algorithm is one of the most well-known algorithms in this group.
Probabilistic modeling provides a framework for adopting the concept of learning. The probabilistic framework specifies how to express and deploy model reservations. In scientific data analysis, predictions play a significant role. Machine learning, automation, cognitive computing, and artificial intelligence all rely heavily on them.
The use of probabilistic models to define the world is portrayed as a common idiom. These were explained using random variables, such as building pieces that were thought to be connected by probabilistic linkages.
In machine learning, there are probabilistic and non-probabilistic models. Basic knowledge of probability concepts, such as random variables and probability distributions, would be beneficial in gaining a thorough understanding of probabilistic models.
Intelligent systems must be able to derive representations from noisy or ambiguous data. Bayes' theorem, in particular, is useful in probability theory as a systematic framework for mixing prior knowledge and empirical evidence.
Key takeaway
Probabilistic Models in Machine Learning is the application of statistical coding to data analysis. It was one of the first machine learning methods. To this day, it's still widely used.
Expert systems are computer programs that are designed to tackle complicated issues in a certain domain at a level of human intellect and expertise.
An expert system is a piece of software that can deal with difficult problems and make choices in the same way as a human expert can. It achieves it by using reasoning and inference techniques to retrieve knowledge from its knowledge base based on the user's requests.
The performance of an expert system is based on the knowledge stored in its knowledge base by the expert. The better the system functions, the more knowledge is saved in the KB. When typing in the Google search box, one of the most common examples of an ES is a suggestion of spelling problems.
An expert system is a computer simulation of a human expert. It can alternatively be described as a computer software that imitates the judgment and conduct of a human or an organization with substantial knowledge and experience in a certain field.
In such a system, a knowledge base containing accumulated experience and a set of rules for applying the knowledge base to each specific case presented to the program are usually provided. Expert systems also leverage human knowledge to solve problems that would normally necessitate human intelligence. These expert systems use computers to transmit expertise knowledge as data or rules.
The expert system is a type of AI, and the first ES was created in 1970, making it the first successful artificial intelligence approach. As an expert, it solves the most difficult problems by extracting knowledge from its knowledge base. Like a human expert, the system assists in decision making for complex problems by employing both facts and heuristics. It is so named because it comprises expert knowledge of a certain subject and is capable of solving any complex problem in that domain. These systems are tailored to a particular field, such as medicine or science.
An expert system's performance is determined on the knowledge stored in its knowledge base by the expert. The more knowledge that is stored in the KB, the better the system performs. When typing in the Google search box, one of the most common examples of an ES is a suggestion of spelling problems.
The following is a block diagram that depicts how an expert system works:
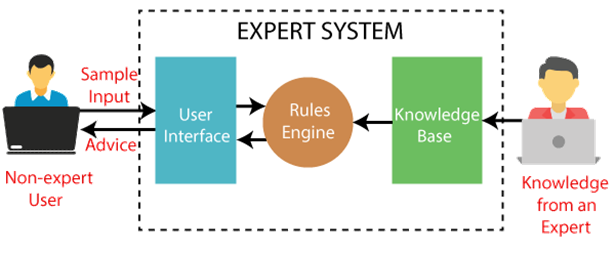
Fig 1: Expert system
Characteristics of Expert System
● High Efficiency and Accuracy - The expert system provides high efficiency and accuracy when addressing any type of complex problem in a specified domain.
● Understandable - It replies in a way that the user can easily comprehend. It can accept human-language input and produce human-language output.
● Reliable - It is really dependable in terms of producing an efficient and accurate result.
● Highly responsive - ES returns the answer to any sophisticated query in a matter of seconds.
Key takeaway
Expert systems are computer programs that are designed to tackle complicated issues in a certain domain at a level of human intellect and expertise.
An expert system is a piece of software that can deal with difficult problems and make choices in the same way as a human expert can.
Inference is used in First-Order Logic to generate new facts or sentences from current ones. It's crucial to understand some basic FOL terms before diving into the FOL inference rule.
Substitution:
Substitution is a fundamental approach for modifying phrases and formulations. All first-order logic inference systems contain it. The substitution becomes more difficult when there are quantifiers in FOL. We refer to the replacement of a constant "a" for the variable "x" when we write F[a/x].
Equality:
Atomic sentences are generated in First-Order Logic not only through the employment of predicate and words, but also through the application of equality. We can do this by using equality symbols, which indicate that the two terms relate to the same thing.
FOL inference rules for quantifier:
Because inference rules in first-order logic are comparable to those in propositional logic, below are some basic inference rules in FOL:
● Universal Generalization
● Universal Instantiation
● Existential Instantiation
● Existential introduction
Universal Generalization:
- Universal generalization is a valid inference rule that states that if premise P(c) is true for any arbitrary element c in the universe of discourse, we can arrive at the conclusion x P. (x).
- It can be represented as:

- If we want to prove that every element has a similar property, we can apply this rule.
- x must not be used as a free variable in this rule.
Example: Let's represent, P(c): "A byte contains 8 bits", so for ∀ x P(x) "All bytes contain 8 bits.", it will also be true.
Universal Instantiation:
- Universal instantiation, often known as universal elimination or UI, is a valid inference rule. It can be used numerous times to add more sentences.
- The new knowledge base is logically equivalent to the previous one.
- We can infer any phrase by replacing a ground word for the variable, according to the UI.
- According to the UI rule, any phrase P(c) can be inferred by substituting a ground term c (a constant inside domain x) for any object in the universe of discourse in x P(x).
- It can be represented as:

Example: IF "Every person like ice-cream"=> ∀x P(x) so we can infer that
"John likes ice-cream" => P(c)
Existential Instantiation:
- Existential Elimination, also known as Existential Instantiation, is a valid first-order logic inference rule.
- It can only be used once to substitute for the existential sentence.
- Despite the fact that the new KB is not conceptually identical to the previous KB, it will suffice if the old KB was.
- This rule states that one can infer P(c) from the formula given in the form of ∃x P(x) for a new constant symbol c.
- The only constraint with this rule is that c must be a new word for which P(c) is true.
- It can be represented as:

Existential introduction
- An existential generalization, also known as an existential introduction, is a valid inference rule in first-order logic.
- If some element c in the world of discourse has the characteristic P, we can infer that something else in the universe has the attribute P, according to this rule.
- It can be represented as:

Example: Let's say that,
"Pritisha got good marks in English."
"Therefore, someone got good marks in English."
Propositional vs. First-Order Inference
Previously, inference in first order logic was checked via propositionalization, which is the act of turning the Knowledge Base included in first order logic into propositional logic and then utilizing any of the propositional logic inference mechanisms to check inference.
Inference rules for quantifiers:
To get sentences without quantifiers, several inference techniques can be applied to sentences containing quantifiers. We'll be able to make the conversion if we follow these requirements.
Universal Instantiation (UI):
The rule states that by substituting a ground word (a term without variables) for the variable, we can deduce any sentence. Let SUBST () stand for the outcome of the substitution on the sentence a. The rule is then written.
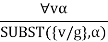
For any v and g ground term combinations. For example, in the knowledge base, there is a statement that states that all greedy rulers are Evil.
x King(x)A Greedy(x) Evil(x)
For the variable x, with the substitutions like {x/John}, {x/Richard} the following sentences can be inferred
King(John) A Greedy(John) Evil(John)
King(Richard) A Greedy(Richard) Evil(Richard)
As a result, the set of all potential instantiations can be used to substitute a globally quantified phrase.
Existential Instantiation (EI):
The existential statement states that there is some object that fulfills a requirement, and that the instantiation process is simply giving that object a name that does not already belong to another object. A Skolem constant is the moniker given to this new name. Existential Instantiation is a subset of a broader process known as "skolemization."
If the statement a, variable v, and constant symbol k do not occur anywhere else in the knowledge base,
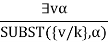
For example, from the sentence
3x Crown(x)A OnHead(x, John)
So we can infer the sentence
Crown(C1)A OnHead(C1, John)
As long as C1 does not appear elsewhere in the knowledge base. Thus, an existentially quantified sentence can be replaced by one instantiation
Elimination of Universal and Existential quantifiers should give new knowledge base which can be shown to be inferentially equivalent to old in the sense that it is satisfiable exactly when the original knowledge base is satisfiable.
Key takeaway
In First-Order Logic, inference is used to derive new facts or sentences from existing ones.
In First-Order Logic, atomic sentences are formed not only via the use of predicate and words, but also via the application of equality.
There are some Inference rules that can be applied to sentences with quantifiers to obtain sentences without quantifiers.
Knowledge acquisition is the process of gathering or collecting information from a variety of sources. It refers to the process of adding new information to a knowledge base while simultaneously refining or upgrading current information. Acquisition is the process of increasing a system's capabilities or improving its performance at a certain activity.
As a result, it is the creation and refinement of knowledge toward a certain goal. Acquired knowledge contains facts, rules, concepts, procedures, heuristics, formulas, correlations, statistics, and any other important information. Experts in the field, text books, technical papers, database reports, periodicals, and the environment are all possible sources of this knowledge.
Knowledge acquisition is a continuous process that takes place throughout a person's life. Knowledge acquisition is exemplified via machine learning. It could be a method of self-study or refining aided by computer programs. The newly acquired knowledge should be meaningfully linked to previously acquired knowledge.
The data should be accurate, non-redundant, consistent, and thorough. Knowledge acquisition helps with tasks like knowledge entry and knowledge base upkeep. The knowledge acquisition process builds dynamic data structures in order to refine current information.
The knowledge engineer's role is equally important in the development of knowledge refinements. Knowledge engineers are professionals who elicit knowledge from specialists. They write and edit code, operate several interactive tools, and create a knowledge base, among other things, to combine knowledge from multiple sources.
Fig 2: Knowledge Engineer’s Roles in Interactive Knowledge Acquisition
Key takeaway
- The gathering or collection of knowledge from multiple sources is known as knowledge acquisition.
- Knowledge acquisition is an ongoing process that spans a person's entire life.
- Machine learning is an example of knowledge acquisition.
A knowledge-based system (KBS) is a computer programme that collects and applies information from various sources. Artificial intelligence aids in the solution of problems, particularly difficult challenges, with a KBS. These systems are largely used to assist humans in making decisions, learning, and doing other tasks.
A knowledge-based system is a popular artificial intelligence application. These systems are capable of making decisions based on the data and information in their databases. They can also understand the context of the data being processed.
An interface engine plus a knowledge base make up a knowledge-based system. The interface engine serves as a search engine, while the knowledge base serves as a knowledge repository. Learning is an important part of any knowledge-based system, and learning simulation helps to enhance it over time. Expert systems, intelligent tutoring systems, hypertext manipulation systems, CASE-based systems, and databases with an intelligent user interface are all examples of knowledge-based systems.
The following are examples of knowledge-based systems:
● Medical diagnosis systems - There are systems that can assist with disease diagnosis, such as Mycin, one of the earliest KBS. Such algorithms can identify likely diagnoses (and include a confidence level around the diagnosis) as well as make therapy suggestions by inputting data or answering a series of questions.
● Eligibility analysis systems - It is possible to determine whether a person is eligible for a particular service by answering guided questions. When determining eligibility, a system would ask questions until it received an answer that was processed as disqualifying, and then transmit that information to the individual, all without the requirement for a third-party person's involvement.
● Blackboard systems - A blackboard system, in contrast to other KBS, is highly reliant on updates from human specialists. A varied collection of people will collaborate to discover the best answer to an issue. Each individual will update the blackboard with a partial solution as they work on the problem until it is eventually solved.
● Classification systems - KBS can also be used to examine various pieces of information in order to determine their classification status. For example, by studying mass spectra and chemical components, information might be inputted to determine distinct chemical compounds.
Key takeaway
A knowledge-based system (KBS) is a computer programme that collects and applies information from various sources. Artificial intelligence aids in the solution of problems, particularly difficult challenges, with a KBS.
Natural Language Processing (NLP) is an artificial intelligence (AI) way of communicating with intelligent computers using natural language such as English.
Natural language processing is essential when you want an intelligent system, such as a robot, to follow your commands, when you want to hear a decision from a dialogue-based clinical expert system, and so on.
Computational linguistics—rule-based human language modeling—is combined with statistical, machine learning, and deep learning models in NLP. These technologies, when used together, allow computers to process human language in the form of text or speech data and 'understand' its full meaning, including the speaker's or writer's intent and sentiment.
NLP is used to power computer programmes that translate text from one language to another, respond to spoken commands, and quickly summarise vast amounts of material—even in real time. Voice-activated GPS systems, digital assistants, speech-to-text dictation software, customer care chatbots, and other consumer conveniences are all examples of NLP in action. However, NLP is increasingly being used in corporate solutions to help businesses streamline operations, boost employee productivity, and simplify mission-critical business processes.
An NLP system's input and output can be anything -
● Speech
● Written Text
Components of NLP
As stated previously, NLP has two components. −
Natural Language Understanding (NLU)
The following tasks are required for comprehension:
● Translating natural language input into meaningful representations.
● Examining many features of the language.
Natural Language Generation (NLG)
It's the process of turning some internal information into meaningful words and sentences in natural language.
It involves −
● Text planning − It comprises obtaining appropriate content from the knowledge base as part of the text planning process.
● Sentence planning − Sentence planning entails selecting necessary words, generating meaningful phrases, and determining the tone of the sentence.
● Text Realization − It is the mapping of a sentence plan into a sentence structure that is known as text realization.
The NLU is more difficult than the NLG.
NLP Terminology
● Phonology - It is the systematic organization of sounds.
● Morphology - It is the study of how words are constructed from simple meaningful units.
● Morpheme − In a language, a morphe is a basic unit of meaning.
● Syntax - It is the process of putting words together to form a sentence. It also entails figuring out what structural role words have in sentences and phrases.
● Semantics - It is the study of the meaning of words and how to put them together to form meaningful phrases and sentences.
● Pragmatics - It is the study of how to use and understand sentences in various settings, as well as how this affects the sentence's meaning.
● Discourse - It is concerned with how the prior sentence influences the interpretation of the following sentence.
● World Knowledge − The term "world knowledge" refers to general knowledge about the world.
NLP Tasks
Because human language is riddled with ambiguities, writing software that accurately interprets the intended meaning of text or voice input is extremely challenging. Homonyms, homophones, sarcasm, idioms, metaphors, grammar and usage exceptions, sentence structure variations—these are just a few of the irregularities in human language that take humans years to learn, but that programmers must teach natural language-driven applications to recognise and understand accurately from the start if those applications are to be useful.
Several NLP activities help the machine understand what it's absorbing by breaking down human text and speech input in ways that the computer can understand. The following are some of these responsibilities:
● Speech recognition, The task of consistently turning voice data into text data is known as speech recognition, or speech-to-text. Any programme that follows voice commands or responds to spoken questions requires speech recognition. The way individuals speak—quickly, slurring words together, with varied emphasis and intonation, in diverse dialects, and frequently using improper grammar—makes speech recognition particularly difficult.
● Part of speech tagging, The method of determining the part of speech of a particular word or piece of text based on its use and context is known as part of speech tagging, or grammatical tagging. 'Make' is a verb in 'I can make a paper aircraft,' and a noun in 'What make of car do you own?' according to part of speech.
● Natural language generation is the job of converting structured data into human language; it is frequently referred to as the polar opposite of voice recognition or speech-to-text.
● Word sense disambiguation is the process of determining the meaning of a word with several meanings using a semantic analysis procedure to discover which word makes the most sense in the current context. The meaning of the verb 'make' in 'make the grade' (achieve) vs.'make a bet', for example, can be distinguished via word sense disambiguation (place).
● NEM (named entity recognition) is a technique for recognising words or sentences as useful entities. 'Kentucky' is a region, and 'Fred' is a man's name, according to NEM.
References:
- E.Rich and K. Knight, Artificial Intelligence, Tata McGraw Hill, 2008.
- Artificial intelligence and soft computing for beginners by Anandita Das Bhattachargee, Shroff Publishers
- Artificial Intelligence – A Practical Approach: Patterson , Tata McGraw Hill, 3rd Edition