UNIT-1
Component of the Decision Making Process
- Introduction:
- Decision making is a daily activity for any human being. There is no exception to that. When it comes to business organizations, decision making is a habit and a process as well.
- Effective and successful decisions make a profit to the company and unsuccessful ones make losses. Therefore, the corporate decision-making process is the most critical in any organization.
- In the decision-making process, we choose one course of action from a few possible alternatives. In the process of decision making, we may use many tools, techniques, and perceptions.
- Besides, we may make our own private decisions or may prefer a collective decision.
- Usually, decision making is hard. The majority of corporate decisions involve some level of dissatisfaction or conflict with another party.
- Let's have a look at the decision-making process in detail.
- Steps of Decision Making Process:
Following are the important steps of the decision-making process. Each step may be supported by different tools and techniques.
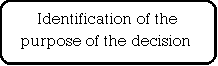

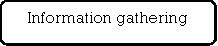

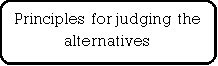

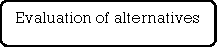

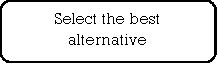

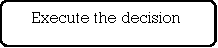

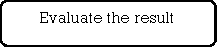
Step 1:- Identification of the purpose of the decision:
In this step, the problem is thoroughly analyzed. There are a couple of questions one should ask when it comes to identifying the purpose of the decision.
- What exactly is the problem?
- Why the problem should be solved?
- Who are the affected parties of the problem?
- Does the problem have a deadline or a specific time-line?
Step 2:- Information gathering:
A problem of an organization will have many stakeholders. Besides, there can be dozens of factors involved and affected by the problem.
In the process of solving the problem, you will have to gather as much information related to the factors and stakeholders involved in the problem. For the process of information gathering, tools such as 'Check Sheets' can be effectively used.
Step 3:- Principles for judging the alternatives:
In this step, the baseline criteria for judging the alternatives should be set up. When it comes to defining the criteria, organizational goals as well as the corporate culture should be taken into consideration.
As an example, profit is one of the main concerns in every decision-making process. Companies usually do not make decisions that reduce profits, unless it is an exceptional case. Likewise, baseline principles should be identified related to the problem at hand.
Step 4:- Brainstorm and analyze the different choices:
For this step, brainstorming to list down all the ideas is the best option. Before the idea generation step, it is vital to understand the causes of the problem and prioritization of causes.
For this, you can make use of Cause-and-Effect diagrams and the Pareto Chart tool. The cause-and-Effect diagram helps you to identify all possible causes of the problem and the Pareto chart helps you to prioritize and identify the causes with the highest effect.
Then, you can move on to generating all possible solutions (alternatives) for the problem at hand.
Step 5:- Evaluation of alternatives:
Use your judgment principles and decision-making criteria to evaluate each alternative. In this step, experience and effectiveness of the judgment principles come into play. You need to compare each alternative for its positives and negatives.
Step 6:- Select the best alternative:
Once you go through from Step 1 to Step 5, this step is easy. Besides, the selection of the best alternative is an informed decision since you have already followed a methodology to derive and select the best alternative.
Step 7:- Execute the decision:
Convert your decision into a plan or a sequence of activities. Execute your plan by yourself or with the help of subordinates.
Step 8: -Evaluate the results:
Evaluate the outcome of your decision. See whether there is anything you should learn and then correct in future decision making. This is one of the best practices that will improve your decision-making skills.
- Conclusion:-
When it comes to making decisions, one should always weigh the positive and negative business consequences and should favour the positive outcomes.
This avoids the possible losses to the organization and keeps the company running with sustained growth. Sometimes, avoiding decision-making seems easier; especially, when you get into a lot of confrontation after making the tough decision.
But, making the decisions and accepting its consequences is the only way to stay in control of your corporate life and time.
1.1.1. Definition:-
- Business intelligence (BI) leverages software and services to transform data into actionable insights that inform an organization’s strategic and tactical business decisions. BI tools access and analyze data sets and present analytical findings in reports, summaries, dashboards, graphs, charts, and maps to provide users with detailed intelligence about the state of the business.
- The term business intelligence often also refers to a range of tools that provide quick, easy-to-digest access to insights about an organization's current state, based on available data.
1.1.2. Business Intelligence Examples:-
- Reporting is a central facet of business intelligence and the dashboard is perhaps the archetypical BI tool. Dashboards are hosted software applications that automatically pull together available data into charts and graphs that give a sense of the immediate state of the company.
- Although business intelligence does not tell business users what to do or what will happen if they take a certain course, neither is BI solely about generating reports. Rather, BI offers a way for people to examine data to understand trends and derive insights by streamlining the effort needed to search for, merge, and query the data necessary to make sound business decisions.
- For example, a company that wants to better manage its supply chain needs BI capabilities to determine where delays are happening and where variabilities exist within the shipping process, says Chris Hagans, vice president of operations for WCI Consulting, a consultancy focused on BI. That company could also use its BI capabilities to discover which products are most commonly delayed or which modes of transportation are most often involved in delays.
- The potential use cases for BI extend beyond the typical business performance metrics of improved sales and reduced costs, says Cindi Howson, research vice president at Gartner, an IT research and advisory firm. She points to the Columbus, Ohio, school system and its success using BI tools to examine numerous data points — from attendance rates to student performance — to improve student learning and high school graduation rates.
- BI vendors Tableau and G2 also offer concrete examples of how organizations might put business intelligence tools to use:
- A co-op organization could use BI to keep track of member acquisition and retention.BI tools could automatically generate sales and delivery reports from CRM data.
- A sales team could use BI to create a dashboard showing where each rep's prospects are on the sales pipeline.
1.1.3. Business Intelligence vs. Business Analytics:
- One thing you will have noticed from those examples is that they provide insights into the current state of the business or organization: where are sales prospects in the pipeline today? How many members have we lost or gained this month? This gets to the key distinction between business intelligence and another, a related term, business analytics.
- Business intelligence is descriptive, telling you what's happening now and what happened in the past to get us to that state. Business analytics, on the other hand, is an umbrella term for data analysis techniques that are predictive — that is, they can tell you what's going to happen in the future — and prescriptive — that is, they can tell you what you should be doing to create better outcomes. (Business analytics are usually thought of as that subset of the larger category of data analytics that's specifically focused on business.)
- The distinction between the descriptive powers of BI and the predictive or descriptive powers of business analytics goes a bit beyond just the timeframe we're talking about. It also gets to the heart of the question of who business intelligence is for. As the Stitch data blog explains, BI aims to deliver straightforward snapshots of the current state of affairs to business managers. While the predictions and advice derived from business analytics require data science professionals to analyze and interpret, one of the goals of BI is that it should be easy for relatively non-technical end-users to understand, and even to dive into the data and create new reports.
- For more, see “Business intelligence vs. Business analytics: Where BI fits in your data strategy.”
1.1.4. Business Intelligence Strategy:
- In the past, IT professionals had been the primary users of BI applications. However, BI tools have evolved to be more intuitive and user-friendly, enabling a large number of users across a variety of organizational domains to tap the tools.
- Gartner’s Howson differentiates two types of BI. The first is traditional or classic BI, where IT professionals use in-house transactional data to generate reports. The second is modern BI, where business users interact with agile, intuitive systems to analyze data more quickly.
- Howson explains that organizations generally opt for classic BI for certain types of reporting, such as regulatory or financial reports, where accuracy is paramount and the questions and data sets used are standard and predictable. Organizations typically use modern BI tools when business users need insight into quickly changing dynamics, such as marketing events, in which being fast is valued over getting the data 100 percent right.
- But while solid business intelligence is essential to making strategic business decisions, many organizations struggle to implement effective BI strategies, thanks to poor data practices, tactical mistakes, and more.
- For more, see “7 keys to a successful business intelligence strategy” and “9 ways you’re failing at business intelligence.”
1.1.5. Self-service Business Intelligence:
- The drive to make it possible for just about anyone to get useful information out of business intelligence tools has given rise to self-service business intelligence, a category of BI tools aimed at abstracting away the need for IT intervention in generating reports. Self-service BI tools enable organizations to make the company's internal data reports more readily available to managers and other non-technical staff.
- Among the keys to self-service BI, success is business intelligence dashboards and UIs that include pull-down menus and intuitive drill-down points that allow users to find and transform data in easy-to-understand ways. A certain amount of training will no doubt be required, but if the advantages of the tools are obvious enough, employees will be eager to get on board. (If you're shopping for a self-service BI solution, CIO.com’s Martin Heller walks you through the decision-making process and compares his top five choices.)
- Keep in mind, though, that there are pitfalls to self-service BI as well. By steering your business users into becoming ad hoc data engineers, you can end up with a chaotic mix of metrics that vary across departments, run into data security problems, and even run up big licensing or SaaS bills if there's no centralized control over the tool rollout. So even if you are committing to self-service business intelligence within your organization, you can't just buy an off-the-shelf product, point your staff to the UI, and hope for the best.
1.1.6. Business Intelligence Software and Systems:
A variety of different types of tools fall under the business intelligence umbrella.
The software selection service Select Hub breaks down some of the most important categories and features:
1) Dashboards
2) Visualizations
3) Reporting
4) Data mining
5) ETL (extract-transfer-load —tools that import data from one data store into another)
6) OLAP (online analytical processing)
Of these tools, Select Hub says the dashboards and visualization are by far the most popular; they offer quick and easy-to-digest data summaries that are at the heart of BI's value proposition.
There are tons of vendors and offerings in the BI space, and wading through them can get overwhelming. Some of the major players include:
1. Tableau, a self-service analytics platform provides data visualization and can integrate with a range of data sources, including Microsoft Azure SQL Data Warehouse and Excel
2. Splunk, a “guided analytics platform” capable of providing enterprise-grade business intelligence and data analytics
3. Alteryx, which blends analytics from a range of sources to simplify workflows as well as provide a wealth of BI insights
4. Qlik, which is grounded in data visualization, BI, and analytics, providing an extensive, scalable BI platform
5. Domo, a cloud-based platform that offers business intelligence tools tailored to various industries (such as financial services, health care, manufacturing, and education) and roles (including CEOs, sales, BI professionals, and IT workers)
6. Dundas BI, which is mostly used for creating dashboards and scorecards, but can also do standard and ad-hoc reporting
7. Google Data Studio, a supercharged version of the familiar Google Analytics offering
8. Einstein Analytics, Salesforce.com’s attempt to improve BI with AI
9. Birst, a cloud-based service in which multiple instances of the BI software share a common data backend.
10. For a deeper look at today’s most popular business intelligence systems, see "Top 12 BI tools of 2019" and "Top 10 BI data visualization tools."
1.1.7. Business Intelligence Analyst:
- Any company that's serious about BI will need to have business intelligence analysts on staff. CIO.com has an in-depth article on what that job entails; in general, they aim to use all the features of BI tools to get the data that companies need, the most important being discovering areas of revenue loss and identifying where improvements can be made to save the company money or increase profits.
- Even if your company relies on self-service BI tools on a day-to-day basis, business intelligence analysts have an important role to play, as they are necessary for managing and maintaining those tools and their vendors. They also set up and standardize the reports that managers are going to be generating to make sure that results are consistent and meaningful across your organization. And to avoid garbage in/garbage out problems, business intelligence analysts need to make sure the data going into the system is correct and consistent, which often involves getting it out of other data stores and cleaning it up.
- Business intelligence analyst jobs often require only a bachelor's degree, at least at the entry-level, though to advance up the ranks an MBA may be helpful or even required. As of October 2019, the median business intelligence salary is around $67,500, though depending on your employer that could range from $49,000 to $94,000.
1.1.8. The Future of Business Intelligence:
- Moving ahead, Howson says Gartner sees a third wave of disruption on the horizon, something the research firm calls “augmented analytics,” where machine learning is baked into the software and will guide users on their queries into the data.
- “It will be BI and analytics, and it will be smart,” she says.
- The combinations included in these software platforms will make each function more powerful individually and more valuable to the businesspeople using them, Gorman says.
- “Someone will look at reports from, for example, last year’s sales — that’s BI — but they’ll also get predictions about next year’s sales — that’s business analytics — and then add to that a what-if capability: What would happen if we did X instead of Y,” Gorman says, explaining that software makers are moving to develop applications that will provide those functions within a single application rather than delivering them via multiple platforms as is now the case.
- “Now the system delivers higher-value recommendations. It makes the decision-maker more efficient, more powerful, and more accurate,” he adds.
- And although BI will remain valuable in and of itself, Howson says organizations can’t compete if they’re not moving beyond only BI and adopting advanced analytics as well.
- Gartner’s Magic Quadrant report predicts that by 2020 organizations offering “users access to a curated catalog of internal and external data will realize twice the business value from analytics investments than those that do not.”
- Howson adds: “There is a need for reporting, but reporting alone is not enough. If you’re only doing reporting you’re behind already. Unless your reporting is smart and agile, you’re behind. You’re a laggard.”
Key Takeaways:
- Business intelligence (BI) leverages software and services to transform data into actionable insights that inform an organization’s strategic and tactical business decisions
- BI tools have evolved to be more intuitive and user-friendly, enabling a large number of users across a variety of organizational domains to tap the tools.
- Business intelligence is descriptive, telling you what's happening now and what happened in the past to get us to that state. Business analytics, on the other hand, is an umbrella term for data analysis techniques that are predictive — that is, they can tell you what's going to happen in the future — and prescriptive — that is, they can tell you what you should be doing to create better outcomes.
- Among the keys to self-service BI, success is business intelligence dashboards and UIs that include pull-down menus and intuitive drill-down points that allow users to find and transform data in easy-to-understand ways. A certain amount of training will no doubt be required, but if the advantages of the tools are obvious enough, employees will be eager to get on board.
1.2.1. Decision Support System (DSS):
- A decision support system (DSS) is an information system that aids a business in decision-making activities that require judgment, determination, and a sequence of actions.
- The information system assists the mid-and high-level management of an organization by analyzing huge volumes of unstructured data and accumulating information that can help to solve problems and help in decision-making.
- A DSS is either human-powered, automated or a combination of both.
1.2.2. Purpose of a Decision Support System:
- A decision support system produces detailed information reports by gathering and analyzing data.
- Hence, a DSS is different from a normal operations application, whose goal is to collect data and not analyze it.
- In an organization, a DSS is used by the planning departments
– Such as the operations department
– Which collects data and creates a report that can be used by managers for decision-making.
- Mainly, a DSS is used in sales projection, for inventory and operations-related data, and to present information to customers in an easy-to-understand manner.
- Theoretically, a DSS can be employed in various knowledge domains from an organization to forest management and the medical field. One of the main applications of a DSS in an organization is real-time reporting. It can be very helpful for organizations that take part in just-in-time (JIT) inventory management.
- In a JIT inventory system, the organization requires real-time data of their inventory levels to place orders “just in time” to prevent delays in production and cause a negative domino effect. Therefore, a DSS is more tailored to the individual or organization that is making the decision rather than a traditional system.
1.2.3. Components of a Decision Support System:
The three main components of a DSS framework are:
1. Model Management System
- The model management system S=stores models that managers can use in their decision-making.
- The models are used in decision-making regarding the financial health of the organization and forecasting demand for a good or service.
2. User Interface
- The user interface includes tools that help the end-user of a DSS to navigate through the system.
3. Knowledge Base
- The knowledge base includes information from internal sources (information collected in a transaction process system) and external sources (newspapers and online databases).
1.2.4. Types of Decision Support Systems:
1. Communication-driven:
Allows companies to support tasks that require more than one person to work on the task. It includes integrated tools such as Microsoft SharePoint Workspace and Google Docs.
2. Model-driven:
Allows access to and the management of financial, organizational, and statistical models. Data is collected, and parameters are determined using the information provided by users. The information is created into a decision-making model to analyze situations. An example of a model-driven DSS is Dicodess – an open-source model-driven DSS.
3.Knowledge-driven:
Provides factual and specialized solutions to situations by using stored facts, procedures, rules, or interactive decision-making structures like flowcharts.
4. Document-driven:
Manages unstructured information in different electronic formats.
5. Data-driven:
Helps companies to store and analyze internal and external data.
1.2.5. Advantages of a Decision Support System:
- A decision support system increases the speed and efficiency of decision-making activities. It is possible, as a DSS can collect and analyze real-time data.
- It promotes training within the organization, as specific skills must be developed to implement and run a DSS within an organization.
- It automates monotonous managerial processes, which means more of the manager’s time can be spent on decision-making
- It improves interpersonal communication within the organization.
1.2.6. Disadvantages of a Decision Support System:
- The cost to develop and implement a DSS is a huge capital investment, which makes it less accessible to smaller organizations.
- A company can develop a dependence on a DSS, as it is integrated into daily decision-making processes to improve efficiency and speed. However, managers tend to rely on the system too much, which takes away the subjectivity aspect of decision-making.
- A DSS may lead to information overload because an information system tends to consider all aspects of a problem. It creates a dilemma for end-users, as they are left with multiple choices.
- Implementation of a DSS can cause fear and backlash from lower-level employees. It is because many of them are not comfortable with new technology and are afraid of losing their jobs to technology.
Key Takeaways:
- A decision support system (DSS) is an information system that aids a business in decision-making activities that require judgment, determination, and a sequence of actions.
- A DSS is either human-powered, automated or a combination of both
- A decision support system (DSS) is an information system that aids a business in decision-making activities that require judgment, determination, and a sequence of actions.
- A decision support system increases the speed and efficiency of decision-making activities.
- A data warehouse is a central repository of information that can be analyzed to make more informed decisions.
- Data flows into a data warehouse from transactional systems, relational databases, and other sources, typically on a regular cadence.
- Business analysts, data engineers, data scientists, and decision-makers access the data through business intelligence (BI) tools, SQL clients, and other analytics applications.
- Data and analytics have become indispensable to businesses to stay competitive.
- Business users rely on reports, dashboards, and analytics tools to extract insights from their data, monitor business performance, and support decision making.
- Data warehouses power these reports, dashboards, and analytics tools by storing data efficiently to minimize the input and output (I/O) of data and deliver query results quickly to hundreds and thousands of users concurrently.
1.3.1. Data Warehouse Architected:
- Data warehouse architecture is made up of tiers. The top tier is the front-end client that presents results through reporting, analysis, and data mining tools. The middle tier consists of the analytics engine that is used to access and analyze the data.
- The bottom tier of the architecture is the database server, where data is loaded and stored. Data is stored in two different types of ways:
-Data that is accessed frequently is stored in very fast storage (like SSD drives) and
-Data that is infrequently accessed is stored in a cheap object store, like Amazon S3.
- The data warehouse will automatically make sure that frequently accessed data is moved into the “fast” storage so query speed is optimized.
1.3.2. Data Warehouse Work:
- A data warehouse may contain multiple databases. Within each database, data is organized into tables and columns. Within each column, you can define a description of the data, such as integer, data field, or string.
- Tables can be organized inside of schemas, which you can think of like folders. When data is ingested, it is stored in various tables described by the schema. Query tools use the schema to determine which data tables to access and analyze.
1.3.3. Benefits of Using a Data Warehouse:
- Informed decision making
- Consolidated data from many sources
- Historical data analysis
- Data quality, consistency, and accuracy
- Separation of analytics processing from transactional databases, which improves the performance of both systems
1.3.4. Working of Data Warehouses, Databases, and Data Lakes Work together:
- Typically, businesses use a combination of a database, a data lake, and a data warehouse to store and analyze data.
- Amazon Redshift’s lake house architecture makes such integration easy.
- As the volume and variety of data increases, it’s advantageous to follow one or more common patterns for working with data across your database, Data Lake, and data warehouse.
1.3.5. Data Warehouse vs Data Lake:
Characteristics | Data Warehouse
| Data Lake |
Data | Relational data from transactional systems, operational databases, and line of business applications
| All data, including structured, semi-structured, and unstructured
|
Schema | Often designed before the data warehouse implementation but also can be written at the time of analysis(schema-on-write or schema-on-read)
| Written at the time of analysis (schema-on-read)
|
Price/Performance
| Fastest query results using local storage
| Query results getting faster using low-cost storage and decoupling of computing and storage
|
Data quality
| Highly curated data that serves as the central version of the truth
| Any data that may or may not be curated (i.e. raw data)
|
Users | Business analysts, data scientists, and data developers
| Business analysts (using curated data), data scientists, data developers, data engineers, and data architects
|
Analytics | Batch reporting, BI, and visualizations
| Machine learning, exploratory analytics, data discovery, streaming, operational analytics, big data, and profiling
|
1.3.6. Data Warehouse vs Database:
Characteristics | Data Warehouse
| Transactional Database |
Suitable workloads
| Analytics, reporting, big data
| Transaction processing |
Data source
| Data collected and normalized from many sources | Data captured as-is from a single source, such as a transactional system
|
Data capture
| Bulk write operations typically on a predetermined batch schedule
| Optimized for continuous write operations as new data is available to maximize transaction throughput
|
Data normalization
| De-normalized schemas, such as the Star schema or Snowflake schema
| Highly normalized, static schemas
|
Data storage | Optimized for simplicity of access and high-speed query performance using columnar storage
| Optimized for high throughout write operations to a single row-oriented physical block
|
Data access | Optimized to minimize I/O and maximize data throughput
| High volumes of small read operations
|
Key Takeaways:
- A data warehouse is a central repository of information that can be analyzed to make more informed decisions
- Data warehouse architecture is made up of tiers. The top tier is the front-end client that presents results through reporting, analysis, and data mining tools. The middle tier consists of the analytics engine that is used to access and analyze the data. The bottom tier of the architecture is the database server, where data is loaded and stored.
- Data flows into a data warehouse from transactional systems, relational databases, and other sources, typically on a regular cadence.
- A data warehouse may contain multiple databases. Within each database, data is organized into tables and columns. Within each column, you can define a description of the data, such as integer, data field, or string.
References :
- Business Intelligence – Data Mining and optimization for Decision Making – Curlo Vercellis-Wiley Publications.
- Data Mining: Concepts and Techniques Second Edition- Jaiwei Han and Micheline Kamber-Morgan KaufMan publisher
- Data Mining and Analysis Fundamental Concepts and Algorithms –Mohammed J. Zaki and Wager Meira Jr. Cambridge University Press.