Unit 1
Introduction to Statistics
The word arithmetic is by all accounts got from the German word - 'measurement', the Italian word - 'statistica' or the Latin word - 'status' which all signifies 'state' in the political feeling of the word. This is by all accounts the case since measurements were utilized broadly at the outset to gather data from the state (Government) with the goal that authorities could utilize the information for better arranging later on.
• Mathematics is information science.
• This incorporates gathering, dissecting and deciphering data.
• According to Bowley "Arithmetic is the study of checking".
• According to Loosvelt "Arithmetic is the study of collection and arrangement of mathematical realities as the reason for the significance of importance and correlation. "According to Croxton and Cowden, "the study of computational and quantitative science."
There are two sorts of measurements:
• Descriptive measurements utilize graphical and mathematical strategies to sum up and show information contained in an informational collection.
• Inferential measurements use test information to settle on choices or anticipate huge quantities of information.
Statistical data is the basic maturity of mathematics. Data may be related to the activity of our interest, surprise, or problematic situation in the study. They are available as a result of the measurement, counting and / or viewing process. Statistical data, therefore, refers to those aspects of the problem that can be measured, measured, calculated, or divided.
Any object that is the subject of an object, or a function that generates data through this process is called variability. In other words, the variance is what indicates the degree of variability when measuring successive measurements.
In statistics, data is divided into two broad categories: measurement data and quality data. This category depends on the type of symbols being measured.
Measurement data are those that can be measured on specific units of measurement. This reference to symbols with successive scales reveals unparalleled perceptions. Depending on the type of variation observed measurement, quantitative data can be categorized as continuous and varied data.
Obviously, a variable can be a continuous or a different variable.
(i)Continuous data represents the numerical values of continuous variables. Continuous variance is what can take any value between any two points in the line segment, thus representing the interval of values. The values are very accurate and close to each other, yet different. All factors such as weight, length, height, rigidity, velocity, temperature, rigidity, etc., speak to a persistent variable. Consequently, the information recorded in these other comparable highlights is called consistent information. It very well may be realized that nonstop adaptability takes the best unit of estimation. It is astounding as in it empowers estimations at a significant level of exactness.
(ii) Different information are values that are considered for various factors. The main distinction is that the outcomes are estimated in fixed numbers. Such information really checks the information. This depends on the estimation cycle, for example, the quantity of things with or without a particular factor. The quantity of clients who visit the retail chain day by day, inbound flights, and deficient things at a bargain, are on the whole instances of exceptional information.
Quality information alludes to the quality markers of a subject or item. A component is a characteristic quality wherein its observation is characterized and set apart as indicated by the presence or nonattendance of a specific trait in various numbers. This data is likewise ordered as selection information and rank.
(I) Nomination subtleties are the consequence of the order of at least two classes of things or units containing an example or populace as indicated by certain quality elements. The isolation of understudies by sex, (for example, 6 guys and females), by gifted specialists, (for example, talented, low-gifted, and uninformed), and by scholarly staff, (for example, secondary school, undergrad, and graduate), all outcome in plan information. Given any reason for order, it is consistently conceivable to relegate everything to a particular class and make an outline of each class thing. The figuring information got is called selection information.
(ii) Position information, then again, is the aftereffect of the designation of principles to decide request regarding numbers 1,2,3, ..., n. Positions can be relegated by the degree of execution in the test. An opposition, rivalry, meeting, or show. Applicants who show up for the meeting, for instance, might be designated positions in full numbers going from I ton, contingent upon their exhibition in the meeting. Positions offered consequently can be seen as consistent estimations of variety including capacity as a quality factor.
(i) There are sure marvels or ideas where measurements can't be utilized. This is on the grounds that these marvels or ideas are not manageable to estimation. For instance, excellence, knowledge, fortitude can't be evaluated. Insights is not welcome in all such situations where measurement is unimaginable.
(ii) Statistics uncover the normal conduct, the ordinary or the overall pattern. A use of the 'normal' idea whenever applied to an individual or a specific circumstance may prompt an off-base end and now and again might be sad. For instance, one might be confused when informed that the normal profundity of a stream from one bank to the next is four feet, when there might be a few focuses in the middle of where its profundity is unmistakably multiple feet. On this arrangement, one may enter those focuses having more prominent profundity, which might be perilous.
(iii) Since measurements are gathered for a specific reason, such information may not be important or helpful in different circumstances or cases. For instance, optional information (i.e., information initially gathered by another person) may not be valuable for the other individual.
(iv) Statistics are not 100% exact as is Mathematics or Accountancy. The individuals who use measurements should know about this restriction.
(iv) In factual reviews, examining is commonly utilized as it isn't actually conceivable to cover all the units or components including the universe. The outcomes may not be fitting taking everything into account. Besides, various reviews dependent on similar size of test yet unique example units may yield various outcomes.
(v) At times, affiliation or connection between at least two factors is concentrated in measurements, yet such a relationship doesn't demonstrate circumstances and logical results' relationship. It essentially shows the likeness or difference in the development of the two factors. In such cases, the client needs to decipher the outcomes cautiously, calling attention to the kind of relationship got.
(vi) A significant impediment of measurements is that it doesn't uncover all relating to a specific wonder. There is some foundation data that insights don’t cover. Additionally, there are some different viewpoints identified with the issue available, which are likewise not covered. The client of Statistics must be very much educated and ought to decipher Statistics remembering all different perspectives having pertinence on the given issue.
1. It simplifies data:
2. It facilitates comparison:
3. It helps in ascertaining the relation between variables:
4. Statistics helps in estimation:
5. Statistics helps in Decision making:
6. Statistics helps in making decisions where an uncertainty is involved:
Data may be qualitative or quantitative. Once you know the difference between them, you can know how to use them.
• Qualitative Data: They speak to certain qualities or characteristics. They portray depictions that might be noticed yet can't be processed or determined. For instance, information on characteristics, for example, insight, trustworthiness, astuteness, tidiness, and imagination gathered utilizing the understudies of your group an example would be delegated subjective. They are more exploratory than decisive in nature.
• Quantitative Data: These can be estimated and not just noticed. They can be mathematically spoken to and computations can be performed on them. For instance, information on the quantity of understudies playing various games from your group gives a gauge of the number of the all out understudies play which sport. This data is mathematical and can be delegated quantitative.
Discrete Data: These are information that can take just certain particular qualities as opposed to a scope of qualities. For instance, information on the blood gathering of a specific populace or on their sexual orientations is named as discrete information. A standard method to speak to this is by utilizing bar graphs.
Constant Data: These are information that can take esteems between a specific reach with the most elevated and least qualities. The distinction between the most elevated and least worth is known as the scope of information. For instance, the time of people can take esteems even in decimals or so is the situation of the tallness and loads of the understudies of your school. These are named consistent information. Consistent information can be classified in what is known as recurrence dissemination. They can be graphically spoken to utilizing histograms.
Let’s start with the easiest one to understand. Nominal scales are used for labelling variables, without any quantitative value. “Nominal” scales could simply be called “labels.” Here are some examples, below. Notice that all of these scales are mutually exclusive (no overlap) and none of them have any numerical significance. A good way to remember all of this is that “nominal” sounds a lot like “name” and nominal scales are kind of like “names” or labels.
Examples of Nominal Scales
What is your Gender? M – Male F – Female | What is your hair color? 1 – Brown 2 – Black 3 – Blonde 4 – Gray 5 – Other | What do you live? 1 – North of the equator 2 – South of the equator 3 – Neither: In the international space station
|
With ordinal scales, it is the request for the qualities is what's significant and critical, yet the contrasts between every one aren’t generally known. Investigate the model beneath. For each situation, we realize that a #4 is superior to a #3 or #2, however we don't have the foggiest idea and can't evaluate how much better it is. For instance, is the contrast among "alright" and "Troubled" equivalent to the distinction between "Exceptionally Happy" and "Upbeat?" We can't state. Ordinal scales are regularly estimating of non-numeric ideas like fulfilment, bliss, distress, and so on "Ordinal" is anything but difficult to recollect in light of the fact that it seems like "request" and that is the way to recall with "ordinal scales"– the request matters, however that is all you truly get from these.
Scales area unit numeric scales within which we all know not solely the order, however conjointly the precise variations between the values. The classic example of an interval scale is urologist temperature as a result of the distinction between every worth is that the same. As an example, the distinction between sixty and fifty degrees may be a measurable ten degrees, as is that the distinction between eighty and seventy degrees. Time is Another ideal of an interval scale within which the increments area unit notable, consistent, and measurable.
A census may be thanks to notice and record data concerning each member of a population. It’s a term largely connected with national data though the term will visit well-defined, smaller populations. As an example, you may take a census of dog house owners, or Boy Scouts, or individuals aged sixty-five and on top of.
A census is that the opposite of sampling:
A census includes each member of the population within the results whereas sampling solely includes a little proportion of the population. Rather than asking all dog house owners that whole their dog prefers; you'd raise a sample of them. Therefore, censuses tend to be additional correct than sampling. That said, a organized survey victimization sampling will paint a reasonably correct image of the whole population.
Sample choice
Survey sampling is choosing members from a target population to be in a very sample for a sample survey. Sometimes the survey is a few varieties of form Sample choice for survey samples make up 2 main types:
• Probability-based samples that choose members supported a notable chance. This uses random choice ways like straightforward sampling or systematic sampling.
• Non-probability samples, wherever the chance of selecting a member can't be calculated. Instead, non-random choice ways use the researcher’s judgment, proximity of subjects, or another non-random issue.
1.10.1 Statistical distribution
In this section, we glance at ways that to prepare information so as to create it user friendly. We start by presenting 2 information sets, from which, due to however the info is bestowed, it's tough to get pregnant data. We are going to gift ways that to prepare and gift the info, from that pregnant outline data are often derived at a look.
Relative frequency:
The frequency of a class is that the frequency of that class (the range of observations that make up the category) divided by the overall range of observations:
Here only a few of the standard graphic forms of representing the data are being discussed as listed below:
1. A Histogram
2. A Bar Diagram or Bar Graph
3. A Frequency Polygon
4. A Cumulative Frequency Curve or Ogive
1.10.2 Pie chart
One approach to blessing our subjective data diagrammatically is utilizing an outline. The pie is painted by a circle (Spanning 360). The elements of the pie cut speaking to each class is corresponding to the recurrence of the class. The point that the cut makes at the centre is furthermore corresponding to the recurrence of the classification; really, the plot for a given class is given by:
Classification point at the centre = frequency class × 3600.
The diagram should consistently cling to the domain rule. That is the extent of the domain of the pie committed to any class is that the equivalent on the grounds that the proportion of the info that lies therein class. This principle is often desecrated to change perception and subtly promote a specific purpose of read.
In a chart, the varied observations or parts area unit painted by the sectors of a circle and therefore the whole circle represents the ad of the values of all parts.
Central angle for a component =
How to make a pie chart or graph?
Construction of making a pie chart or graph can be derived from the given data:
Steps of pie graphs Construction:
1. Calculate the central angle for each component, given by
Central angle for a component =
2. Draw a circle of convenient radius.
3. Within this circle, draw a horizontal radius.
4. Starting with the horizontal radius, draw radii making central angles corresponding to the values of the respective components, till all the components are exhausted. These radii divide the whole circle into various sectors.
5. Shade each sector with different design.
This will be the required pie chart for the given data.
Pie chart examples on how to do a pie chart:
1. Mr. Bin's with a yearly salary of ₹ 10800 plans his budget for a year as given below:
Item | Food | Education | Rent | Savings | Miscellaneous |
Amount (in ₹) | 3150 | 1950 | 2100 | 2400 | 1200 |
Represent the above data by a pie graph.
Solution:
Total amount earned in a year = ₹10800.
Central angle of component =
Calculation of central angles
Item | Amount (in ₹) | Central Angle |
Food | 3150 | (³¹⁵/₁₀₈₀₀×360) ° = 105° |
Education | 1950 | (¹⁹⁵/₁₀₈₀₀×360) ° = 65° |
Rent | 2100 | (²¹/₁₀₈₀₀×360) ° = 70° |
Savings | 2400 | (²⁴/₁₀₈₀₀×360) ° = 80° |
Miscellaneous | 1200 | (¹²/₁₀₈₀₀×360) ° = 40° |
Construction of making pie chart
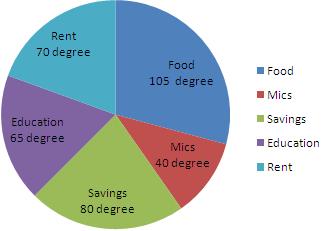
Steps of construction:
1. Draw a circle of any convenient radius.
2. Draw a horizontal radius of this circle.
3. Draw sectors starting from the horizontal radius with central angles of 105-degree, 65-degree, 70-degree, 80 degree and 40 degree respectively.
4. Shade the sectors differently using different colours and label them.
Thus, we obtain the required pie chart, as shown in the given figure.
1.10.3 Bar graph
We can likewise speak to our information graphically on a Bar Chart or Bar Graph. Here the classifications of the subjective variable are spoken to by bars, where the stature of each bar is the classification recurrence, classification relative recurrence, or class rate.
The bases, all things considered, should be equivalent in width. Having equivalent bases guarantees that the reference diagram holds fast to the zone standard, which for this situation implies that the extent of the complete zone of the bars committed to a classification (= zone of the bar over a classification partitioned by the amount of the zones, everything being equal) should be equivalent to the extent of the information in the class.
The following table shows the number of visitors to a park for the months January to March.
Month | January | February | March |
Number of visitors | 150 | 300 | 250 |
a) Construct a vertical and a horizontal bar chart for the table.
b) What is the percentage of increase of visitors to the park in March compared to January?
c) What percentage of visitors came in February compared with total number of visitors over the three months?
Solution:
a) If we choose a scale of 1:50 for the frequency then the vertical bar chart and horizontal bar chart will be as shown.
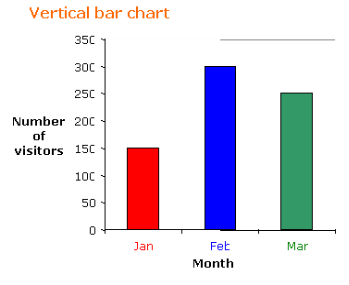
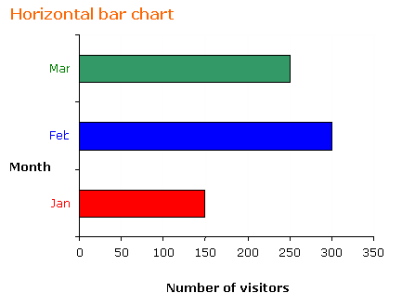
b) Increase in March compared to January is
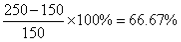
c) Percentage of visitors in February compared to the total number of visitors is

1.10.4 Histograms
A histogram is a bar chart in which each bar represents a category and its height represents either the frequency, relative frequency (proportion) or percentage in thatcategory.
If a variable can only take on a finite number of values (or the values can be listed in an infinite sequence) the variable is said to bediscrete.
- The histogram for a frequency distribution is given below.
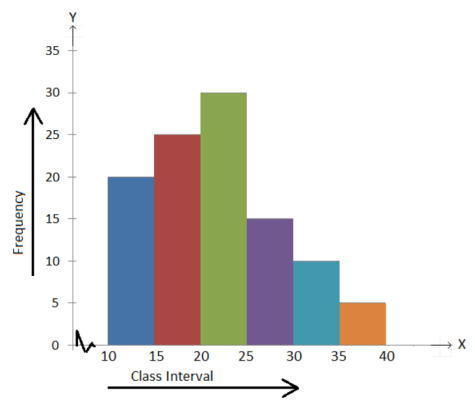
Answer the following.
(i) What is the frequency of the class interval 15 – 20?
(ii) What is the class intervals having the greatest frequencies?
(iii) What is the cumulative frequency of the class interval 25 – 30?
(iv) Construct a short frequency table of the distribution.
(v) Construct a cumulative frequency table of the distribution.
Solution:
(i) 25
(ii) 20 – 25
(iii) 90
(iv)
Class Interval 10 - 15 15 - 20 20 - 25 25 - 30 30 - 35 35 - 40 | Frequency 20 25 30 15 10 5 |
(v)
Class Interval 10 - 15 15 - 20 20 - 25 25 - 30 30 - 35 35 - 40 | Frequency 20 25 30 15 10 5 | Cumulative Frequency 20 45 75 90 100 105 |
1.10.5 Frequency Distribution
Frequency polygons are a graphical device for understanding the shapes of distributions. They serve the same purpose as histograms, but are especially helpful for comparing sets of data. Frequency polygons are also a good choice for displaying cumulative frequency distributions.
Frequency Distribution of Psychology Test Scores.
Lower Limit | Upper Limit | Count | Cumulative Count |
29.5 | 39.5 | 0 | 0 |
39.5 | 49.5 | 3 | 3 |
49.5 | 59.5 | 10 | 13 |
59.5 | 69.5 | 53 | 66 |
69.5 | 79.5 | 107 | 173 |
79.5 | 89.5 | 147 | 320 |
89.5 | 99.5 | 130 | 450 |
99.5 | 109.5 | 78 | 528 |
109.5 | 119.5 | 59 | 587 |
119.5 | 129.5 | 36 | 623 |
129.5 | 139.5 | 11 | 634 |
139.5 | 149.5 | 6 | 640 |
149.5 | 159.5 | 1 | 641 |
159.5 | 169.5 | 1 | 642 |
169.5 | 179.5 | 0 | 642 |
The primary name on the X-pivot is 35. This speaks to a span stretching out from 29.5 to 39.5. Since the most reduced grade is 46, this span has a recurrence of 0. The point named 45 speaks to the stretch from 39.5 to 49.5. There are three scores in this span. There are 147 scores in the span that encompasses 85.
You can undoubtedly observe the state of the appropriation from Figure. The greater part of the scores are somewhere in the range of 65 and 115. Plainly the conveyance isn't symmetric since acceptable scores (to one side) trail off more continuously than
Figure: Frequency polygon for the psychology test scores.
1.10.6 Cumulative frequency or OGIVES
A cumulative frequency polygon for the same test scores is shown in Figure. The graph is the same as before except that the Y value for each point is the number of students in the corresponding class interval plus all numbers in lower intervals. For example, there are no scores in the interval labelled "35," three in the interval "45," and 10 in the interval "55." Therefore, the Y value corresponding to "55" is 13. Since 642 students took the test, the cumulative frequency for the last interval is 642.
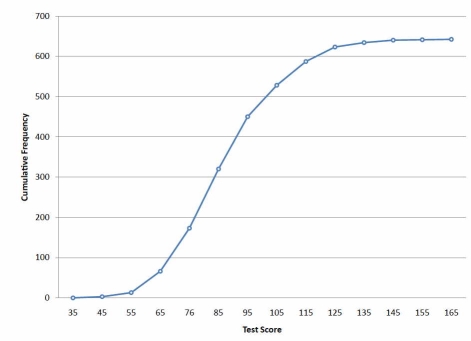
Figure: Cumulative frequency polygon for the psychology test scores.
Frequency polygons are useful for comparing distributions. This is achieved by overlaying the frequency polygons drawn for different data sets. Figure 3 provides an example. The data come from a task in which the goal is to move a computer cursor to a target on the screen as fast as possible. On 20 of the trials, the target was a small rectangle; on the other 20, the target was a large rectangle. Time to reach the target was recorded on each trial. The two distributions (one for each target) are plotted together in Figure . The figure shows that, although there is some overlap in times, it generally took longer to move the cursor to the small target than to the large one.
1.2.1 Central tendency
A measure of central tendency is a single value that attempts to describe a set of data by identifying the central position within that set of data. As such, measures of central tendency are sometimes called measures of central location.
Terms for statistics
- Population: Any well-defined set of objects about which a statistical inquiry is being made is the population. The information required from each individual member of the population is called its characteristic.
- Variate and attribute: if the characteristic that is being studied is numerical then it is called variate. The qualitative characteristic under study is called attribute
- Discrete variable: A variate which takes only integer values, like 1, 2, 3, …. 10,100, even 0, -2,-5 etc. will come under a discrete variate
- Continuous variate: A variate which is capable of taking all the values is called continuous values.
- Data in statistics: when the characteristic under study of a population is collected, it is called data.
- Frequency distribution: if instead of 10 students, we have 100 students then frequency is better choice to handle this data, frequency will give number of times the relevant variate
- Cumulative Frequencies: for each value x of a variate, we can speak of the total number of observations less than or equal to x, within its range.
- Arithmetic mean (A.M): It is mean or the average value.
- How Arithmetic Mean is Calculated
The calculation of arithmetic mean can be studied under two broad categories:
1. Arithmetic Mean for Ungrouped Data.
2. Arithmetic Mean for Grouped Data.
- Arithmetic Mean for Series of Ungrouped Data
Direct Method
Arithmetic mean by direct method is the sum of all observations in a series divided by the total number of observations.
Assumed Mean Method
If the number of observations in the data is more and/or figures are large, it is difficult to compute arithmetic mean by direct method. The computation can be made easier by using assumed mean method.
Let,
A = assumed mean
X = individual observations
N = total numbers of observations
d = deviation of assumed mean from individual observation,
i.e. d = X – A Then sum of all deviations is taken as Σd=Σ (X-A)
Then find Then add A and Σd N to get X
Therefore, = A +
You should remember that any value, whether existing in the data or not, can be taken as assumed mean. However, in order to simplify the calculation, centrally located value in the data can be selected as assumed mean.
Q1. In a college having 70% girls, the average of students opting for C.A is 25%. If the average of boys opting for C.A is 15%, find the average of girls opting for C.A course.
Solution: X =
Let x1 = average of boys opting for C.A
=15%
N1= number of boys in the college with 70% girls
= (100- 70) % = 30%
X2= average of girls opting for C.A to be found out
N2 = number of girls in the college
= 70%
X = combined average
= 25%
Substituting in the formula
X =
25 =
X2 = 29.2857
The average of girls opting for C.A is 29.2857
Q2Calculate Arithmetic Mean from the data showing marks of students in aclass in an economics test: 40, 50, 551 78, 58
Solution: ![]()
![]() |
Q3. | The following data shows the weekly Income of 10 families. Family ![]() Weekly Income (in Rs) ![]()
Solution | ||||||||||||||||||||||||||||||||||||||||||||||||
| Compute mean family income. | ||||||||||||||||||||||||||||||||||||||||||||||||
| Computation of Arithmetic Mean by Assumed Mean Method | ||||||||||||||||||||||||||||||||||||||||||||||||
|
| ||||||||||||||||||||||||||||||||||||||||||||||||
| Arithmetic Mean using assumed mean method
![]() ![]() Thus, the average weekly income of a family be each method is Rs.1,116. You can check this by sung the direct method. |
1.2.2 Weighted arithmetic mean
The math mean, meant x, of a bunch of n numbers x1, x2, … , xn is characterized as the amount of the numbers isolated by n:
The math mean (generally inseparable from normal) speaks to a point about which the numbers balance. For instance, if unit masses are set on a line at focuses with organizes x1, x2, … , xn, at that point the number juggling mean is the arrange of the focal point of gravity of the framework.
Q1: A student obtained the marks 40, 50, 60, 80, and 45 in math, statistics, physics, chemistry and biology respectively. Assuming weights 5, 2, 4, 3, and 1 respectively for the above-mentioned subjects, find the weighted arithmetic mean per subject.
Solution: | Mark Obtained | Weight | Wxwx |
Math | 4040 | 55 | 200200 |
Statistics | 5050 | 22 | 100100 |
Physics | 6060 | 44 | 240240 |
Chemistry | 8080 | 33 | 240240 |
Biology | 4545 | 11 | 4545 |
Total |
| ∑w=15∑w=15 | ∑wx=825∑wx=825 |
Now we will find the weighted arithmetic mean as:
Xw=∑w x ∑w=82515=55X
w=∑w x ∑w=82515=55 marks/subject.
Q2. Suppose that a marketing firm conducts a survey of 1,000 households to determine the average number of TVs each household owns. The data show a large number of households with two or three TVs and a smaller number with one or four. Every household in the sample has at least one TV and no household has more than four.
Solution:
Here’s the sample data for the survey:
Number of TVs per Household | Number of Households |
1 | 73 |
2 | 378 |
3 | 459 |
4 | 90 |
As many of the values in this data set are repeated multiple times, you can easily compute the sample mean as a weighted mean. Follow these steps to calculate the weighted arithmetic mean:
Step 1: Assign a weight to each value in the dataset:
x1=1,w1=73
x2=2,w2=378
x3=3,w3=459
x4=4,w4=90
Step 2: Compute the numerator of the weighted mean formula.
Multiply each sample by its weight and then add the products together:
==w1x1+w2x2+w3x3+w4x4
= (1)(73)+(2)(378)+(3)(459)+(4)(90)
=2566
Step 3: Now, compute the denominator of the weighted mean formula by adding the weights together.
==w1+w2+w3+w4
= 73 + 378 + 459 + 90
=1000
Step 4: Divide the numerator by the denominator
=/ wi
=2566/1000
=2.566
The mean number of TVs per household in this sample is 2.566.
Q3 | Plots in a housing colony come in only three sizes: 100 sq. Metre, 200 sq. Metres and 300sq. Metre and the number of plots is respectively 200, 50 and 10. Solution: | |||||||||||||||||||||||||
| Computation of Arithmetic Mean by Direct Method | |||||||||||||||||||||||||
|
| |||||||||||||||||||||||||
| Arithmetic mean using direct method.
![]() |
Q4 | Calculate average marks of the following students using (a) Direct method (b) Step deviation method. | ||||||||||||||||||||||||||||||||||||||||||||||||||||||||
| Direct Method Marks ![]()
No. Of Students ![]() | ||||||||||||||||||||||||||||||||||||||||||||||||||||||||
| Computation of Average Marks for Exclusive Class Interval by Direct Method | ||||||||||||||||||||||||||||||||||||||||||||||||||||||||
|
| ||||||||||||||||||||||||||||||||||||||||||||||||||||||||
| Steps: | ||||||||||||||||||||||||||||||||||||||||||||||||||||||||
| 1. | Obtain mid values for each class denoted by m. | |||||||||||||||||||||||||||||||||||||||||||||||||||||||
| 2. | Obtain ![]() | |||||||||||||||||||||||||||||||||||||||||||||||||||||||
| ![]() | ||||||||||||||||||||||||||||||||||||||||||||||||||||||||
| Step deviation method | ||||||||||||||||||||||||||||||||||||||||||||||||||||||||
| 1 | Obtain ![]() | |||||||||||||||||||||||||||||||||||||||||||||||||||||||
| 2. | Take A=35. (any arbitrary figure). C= common factor. | |||||||||||||||||||||||||||||||||||||||||||||||||||||||
| ![]() ![]() | ||||||||||||||||||||||||||||||||||||||||||||||||||||||||
1.2.3 MODE
In statistics, finding mode of grouped data is given with a formula. It is the number that occurs most frequently in given data. For example, for the data 1,2,3,4,5 the mode is 3, because it occurs for maximum times.
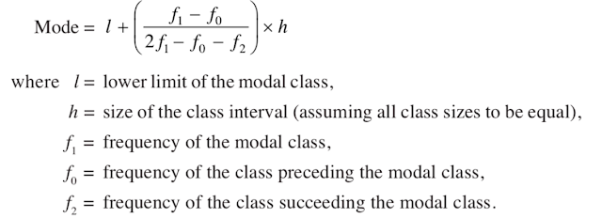
Q1. A survey was conducted in a housing complex, to find out numbers of person in various age groups, who are using the ATM cards of banks. The result of the survey is as shown below.
Age group | Number of persons |
Below 20 | 10 |
Below 40 | 25 |
Below 60 | 50 |
Below 80 | 70 |
Find the mode of above distribution
Solution:
Class interval | Frequencies |
0-20 | 10 |
20-40 | 25-10=15 |
40-60 | 50-25=25 |
60-80 | 70-50=20 |
The maximum frequency 25 is for the class 40-60
Class 40-60 is modal class.
Hence, l1=40
L2= 60
F1=25
F0= 15
F2= 20
Mode = 53.3333
Q 2 | Calculate the value of modal worker family’s monthly income from the following data: | ||||||||||||||||||||||||||||||||||||||||||||||||||||||
| Less than cumulative frequency distribution Of income per month (in ‘000 Rs) | ||||||||||||||||||||||||||||||||||||||||||||||||||||||
|
| ||||||||||||||||||||||||||||||||||||||||||||||||||||||
| Solution: | ||||||||||||||||||||||||||||||||||||||||||||||||||||||
| This table should be converted into an ordinary frequency Table to determine the modal class. | ||||||||||||||||||||||||||||||||||||||||||||||||||||||
|
| ||||||||||||||||||||||||||||||||||||||||||||||||||||||
| The value of the mode lies in 25–30 class interval. By inspection also, it can be seeming that this is a modal class. Now L =25, D1 (30–18) = 12, D2 = (30-20) =10, h = 5 Using the formula, you can obtain the value of the mode as: | ||||||||||||||||||||||||||||||||||||||||||||||||||||||
| ![]()
![]() | ||||||||||||||||||||||||||||||||||||||||||||||||||||||
| Thus, the modal worker family’s monthly income is Rs 27.273. |
1.2.4 Mode using histogram
The highest peak of the histogram represents the location of the mode of the data set. The mode is the data value that occurs the most often in a data set. For a symmetric histogram, the values of the mean, median, and mode are all the same and are all located at the centre of the distribution.
Draw a histogram for the following information.
Height (feet): | Frequency | Relative Frequency |
0-2 | 0 | 0 |
2-4 | 1 | 1 |
4-5 | 4 | 8 |
5-6 | 8 | 16 |
6-8 | 2 | 2 |
When drawing a histogram, the y-axis is labelled 'relative frequency' or 'frequency density'. You must work out the relative frequency before you can draw a histogram. To do this, first you must choose a standard width of the groups. Some of the heights are grouped into 2s (0-2, 2-4, 6-8) and some into 1s (4-5, 5-6).
Most are 2s, so we shall call the standard width 2. To make the areas match, we must double the values for frequency which have a class division of 1 (since 1 is half of 2). Therefore, the figures in the 4-5 and the 5-6 columns must be doubled. If any of the class divisions were 4 (for example if there was an 8-12 group), these figures would be halved. This is because the area of this 'bar' will be twice the standard width of 2 unless we half the frequency.
Area of bar = frequency x standard width.
The position of the median is
{(n + 1) ÷ 2}th value, where n is the number of values in a set of data.
In order to calculate the median, the data must first be ranked (sorted in ascending order). The median is the number in the middle.
1.3.1 Raw data
In raw data, the median is the point at which exactly half of the data are above and half below. These halves meet at the median position. If the number of observations is odd, the median fits perfectly and the depth of the median position will be a whole number. If the number of observations is even, the depth of the median position will include a decimal. You need to find the midpoint between the numbers on either side of the median position.
Example 1 – Raw data (discrete variables)
Imagine that a top running athlete in a typical 200-metre training session runs in the following times:
26.1, 25.6, 25.7, 25.2 et 25.0 seconds.
How would you calculate his median time?
Solution:First, the values are put in ascending order: 25.0, 25.2, 25.6, 25.7, 26.1. Then, using the following formula, figure out which value is the middle value. Remember that n represents the number of values in the data set.
Median = {(n + 1) ÷ 2}th value
= (5 + 1) ÷ 2
= 3
The third value in the data set will be the median. Since 25.6 is the third value, 25.6 seconds would be the median time.
= 25.6 seconds
1.3.2 Ungrouped frequency distribution
In order to find the median using cumulative frequencies you must calculate the first value with a cumulative frequency greater than or equal to the median. If the median's value is exactly 0.5 more than the cumulative frequency of the previous value, than the median is the midpoint between the two values.
Imagine that your school baseball team scores the following number of home runs in 10 games:
4, 5, 8, 5, 7, 8, 9, 8, 8, 7
If you were to place the total home runs in a frequency table, what would the median be?
First, put the scores in ascending order:
4, 5, 5, 7, 7, 8, 8, 8, 8, 9
Number of home runs | Frequency |
4 | 1 |
5 | 2 |
6 | 0 |
7 | 2 |
8 | 4 |
9 | 1 |
To find the median, again use the same formula:
Median = {(n + 1) ÷ 2}th value
= (10 + 1) ÷ 2
= 11 ÷ 2
= 5.5
= the median is the 5.5th value in the data set
1.3.3 Grouped variables - frequency distribution
Eight (cm) | Frequency (f) | Endpoint (x) | Cumulative frequency | Percentage | Cumulative percentage |
150 to < 155 | 4 | 155 | 4 | 8 | 8 |
155 to < 160 | 7 | 160 | 11 | 14 | 22 |
160 to < 165 | 18 | 165 | 29 | 36 | 58 |
165 to < 170 | 11 | 170 | 40 | 22 | 80 |
170 to < 175 | 6 | 175 | 46 | 12 | 92 |
175 to < 180 | 4 | 180 | 50 | 8 | 100 |
Using the grouped data, you created a cumulative frequency graph to accompany your table. The endpoints of the height intervals, the numbers for cumulative frequency and the numbers for cumulative percentage have been plotted on the graph.
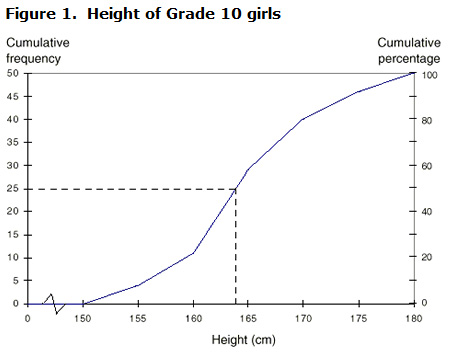
By just looking at the graph, you can try to find the median value. The median is the point where the x-axis (Height) intersects with the midpoint (25) of the y-axis (Cumulative frequency). You will see that the median value is approximately 164 cm. Using mathematical calculations, you can find out that the value is actually 163.9 cm. Here's how:
- According to the information provided in Table 2:
Median = {(n + 1) ÷ 2}th value
= (50 + 1) ÷ 2
= 51 ÷ 2
= 25.5
By adding up the frequencies, we find that the median (25.5) lies in the median group of the 160 to < 165 cm interval. - The cumulative percentage of the preceding interval (A) is 22.
- The percentage needed in order to get 50% of the total cumulative percentage (B) is 28.
B = 50 - A
= 50 - 22
= 28 - The range of the median interval (C) is 5 and the percentage for the median interval (D) is 36.
- The number of values to count down within the interval in order to get to 50% of the total data set is 3.9.
E = (B ÷ D) x C
= (28 ÷ 36) x 5
= 3.9 - Since the lower value of the median interval is 160, when you add the value of E to that you get a median of 163.9 cm.
Median = lower value of median interval + (B ÷ D) x C
= 160 + (28 ÷ 36) x 5
= 160 + 3.9
= 163.9 cm
Consider the following sets of data that represent the number of points scored by 3 players in 11 lacrosse games.
Eileen: 1, 1, 1, 2, 2, 2, 2, 2, 3, 3, 3
Mean = 22 ÷ 11 = 2
Median = 2
Jeremy: 1, 1, 1, 2, 2, 2, 2, 2, 3, 3, 4
Mean = 23 ÷ 11 = 2.1
Median = 2
Randy: 1, 1, 1, 2, 2, 2, 2, 2, 3, 3, 14
Mean = 33 ÷ 11 = 3
Median = 2
The three sets of data above are identical except for the last observation values (3, 4 and 14).
The median does not alter because it is only dependent on the middle observation's value. The mean does change, however, because it is dependent on the average value of all observations. So, in the above example, as the last value of the last observation increases, so too does the mean.