Unit - 3
Organization structures of Business analytics
Q1) What is Team Management? Write its importance in business analytics.5
A1) Are you planning to start a business, or are you ready for a managerial profile and confused about how to manage individuals with different skills, trends, lifestyles, and belief systems? Second, team management strategies and their proper implementation help motivate all team members to achieve common or organizational goals.
This post introduces the world of team management, how to incorporate the importance, type, and effective team management strategy of team management, and channel individual teams to get the most out of their assigned tasks. Helps you understand. Accuracy and effectiveness in a results-oriented way.
The parable of two sons, his father, and a bunch of sticks taught us that we are united and strong very early in our lives.
A bunch of rods cannot be easily broken, but a single rod can be easily broken. Similarly, working as a team creates more workforce than taking over goals with one hand.
Today, many organizations focus on employee harmony by paying attention to the quality of good leadership to perform the desired work as needed.
If someone understands the importance of teamwork as part of project management, you can improve the overall performance of your company. There are many important steps to achieving functional team management. Continue reading every corner of team management and leadership.
Team management is the idea that groups of people work together to achieve a common goal. The top leaders will lead this team.
Leaders need to manage the needs and satisfaction of their employees. This inevitably improves work performance and results in profitable results. Team management is the collective effort of colleagues to reach the desired organizational skills needed to accomplish a team's mission.
Team managers assign projects or day-to-day tasks, prioritize opinions as well, and discuss issues in open forums. It's also important to ensure that employees don't feel left behind and avoid potential conflicts while working.
Importance of team management
Team management has several advantages, including:
1. Appropriate team building
Assigning the right work to an employee's educational background and personality traits is essential for the entire team and for maximum efficiency. This is why previous education is important for employees as well.
2. Significant increase in productivity
Teamwork and clear communication between employees and leaders improve productivity and organizational objectivity. Having a clear vision of your responsibilities helps your staff focus more on their tasks.
3. Opportunity to explore
Teamwork facilitates learning and offers exploration of new ideas and perspectives. In addition, cooperation suggests the exchange of skills, which leads to different outcomes for further success in different areas of life.
4. Consumer satisfaction
By increasing productivity and exploring a unique perspective, you can complete your project in a timely manner and maximize your satisfaction. A healthy workplace environment also creates a sense of accomplishment for employees.
5. Motivation
Employees need motivation from time to time. This can be achieved by delegating exciting tasks and providing additional incentives for considerable effort.
6. Feedback
Gathering workers' 360-degree feedback and opinions gives them the opportunity to improve their results for consumer satisfaction. It also stands out from the crowd and helps to create innovative ideas for future projects of the organization.
Q2) Explain the types of team models. Write the tips and strategies for team management. 8
A2) Team model type
1. Cross-functional team
In such teams, employees with different work backgrounds and responsibilities come together to achieve common goals. This team includes people with different interests, knowledge and skills to complete the task.
2. Self-management team
Voluntary or self-managed teams work without leaders and organize their work to better understand their responsibilities and the skills to make the right decisions.
3. Special purpose team
Special purpose teams or temporary teams are set up to achieve short-term goals super-efficiently.
4. Task force team
Such teams are organized to work exclusively for a short period of time for a special or important purpose. This team is run by a task force and strict deadlines.
5. Virtual team or cyber team
Virtual or cyber teams are separated by distance and combined by a computer or other electronic device. They work on internet related projects and work together to reach their goals for better results.
How to manage a team?
Some useful team management strategies and tips
You have many basic skills needed for good team management. Successful team management consists of factors. This is an important skill that managers of all teams need to make their teams work well.
Leaders need to know how to delegate, direct, control, and maximize the results provided by their team. Let's take a look at some useful strategies that can help you manage your team effectively.
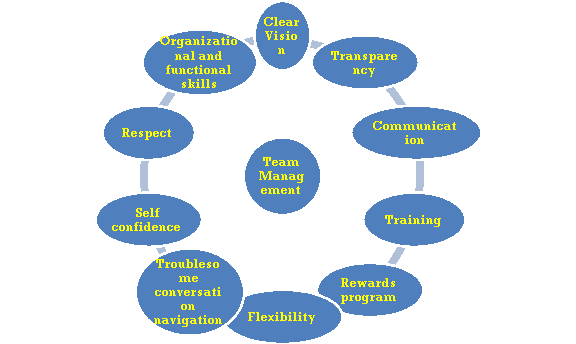
1. Communication
Communication between leaders and employees is essential for a better understanding of work and work.
2. Transparency
A transparent work strategy helps build employee trust in leaders.
3. Clear vision
A logically and strategically active mind for better execution is essential as one of the qualities of leadership.
4. Organizational and functional skills
The ability to accurately organize tasks and distribute them among staff is as important as certain practical skills that must be disseminated during the task force. Also known as delegation.
Proper delegation of authority ensures effective team management. Used when work is assigned between team members. It also helps maintain the trust of employees working under the manager. Efficient delegation shows employees that managers have confidence and confidence in their abilities and work skills.
5. Respect
It is important to have a polite environment in your office or workplace. It is essential to listen to the issues of seniors and colleagues in order to manage them without interruption.
6. Self-confidence
It is beneficial to build confidence in employees who maintain heartfelt behavior. We also encourage you to build relationships based on trust.
7. Troublesome conversation navigation
In the process of work, do not feel that no one has been left behind or heard. When discussing difficult issues, you need to create peace.
8. Flexibility
Team members should be given the opportunity to use their knowledge about solving tasks. In addition, it should be given flexibility in the workplace. In short, you need to be able to maintain a balance between healthy work and life. It also helps you be more productive and productive. Increasing business output helps maintain a balance of effective team management.
9. Rewards program
Team management also includes awards for high-performing employees. It shows them that their efforts are not wasted and are drawing attention. These efforts should be recognized, among other things, through promotions, rewards and prices. These are important parts of effective team management as they also help increase employee self-confidence and satisfaction.
10. Training
You can update each employee's skills through regular exercise and development programs. Then it helps to build a strong team that can work on new issues and use cases. It also guarantees team learning and personal growth.
You can significantly improve your team's performance by honing your employees' skill sets through learning methods, new features, tools, and training sessions. In addition, it helps ensure proper team management.
Q3) Raman wants to make its team in business. What features does he need for its team management. Also give few examples of team management. 5
A3) Team characteristics that team management strategies need to channel
Successful team management has several specific features. Workplace and team success begins with the ability of team managers to build healthy and happy teams. It also depends on the interaction of team members.
Together, the team is strong and can accomplish many things. However, splitting prevents the team from achieving their goals. Some of the characteristics of a good team are:
1. Collaboration environment
Strong collaboration is needed between team members. Appropriate commitment and good leadership from each member of the team always paves the way for creating a collaborative team. The result is a productive work environment that leads to the growth of everyone.
2. Efforts for unified work
Effective team management consists primarily of full commitment from each team member. This is the only way to easily and efficiently achieve your organization's goals and goals.
3. Respectful outlook for different opinions
Not only do we need to respect all the members of the team, but we also need mutual trust. It is an important factor in working together towards the goals of the company. Different opinions should never be ignored or ignored. They should always be evaluated and considered to check feasibility. They should also be recorded so that they can be revisited in the event of a future situation that requires it exactly.
4. Principal leadership
Fair and strong leadership defines the team. Team managers need to be competent leaders with the experience they need to lead their teams to success.
5. Active participation
Each team member must be actively involved in the tasks that the team is working on. They need to actively attend meetings and work to support the growth of the team. This reflects the level of commitment of team members and their understanding of the project or task.
6. Awareness of effort
The efforts of individual team members should be acknowledged. It helps show them that their work has not been overlooked. It also effectively builds their trust in organizations, teams, and projects.
7. Realistic deadline
You need to give your team an appropriate deadline for your project. It should not be too tight or too wide. This avoids stress when the deadline is approaching and loosening when the deadline expires. Appropriate external support and assistance should be provided to the team as needed.
Team management example
Business department team management
Teamwork is required in every area of life. The fields of politics, business, sports and entertainment are supported by teamwork. This team management works towards a specific purpose and achieves union victory.
Here are some examples of team management:
1) Business department team management
Take, for example, the marketing manager of a company. The marketing manager's goal of selling a branded product or service in a particular region or region.
The marketing leader needs to manage several teams under her or him. These teams include a branding team that is also responsible for design and packaging, a product team that guarantees product quality, a marketing team that is responsible for communication, and a sales team that manages product sales.
Therefore, overall, marketing managers need to coordinate the efforts of all teams to ensure sales success. It is only possible with practical and efficient team management.
2) Team management in the sports department
Whether it's hockey, cricket, soccer or basketball, you need effective management of your team.
The team coach or team manager ensures that all players have a strong bond and a good understanding of them. The coach also ensures that the team is always in good shape and always has the motivation to win the game.
Team managers can also take care of team doctors, player consultants, public relations (PR) managers, club boards, and more. All of these factors come together to help your team work better, win the game and make a profit.
Q4) You probably had a bad manager or were a new manager. So you know that you can make big mistakes. Throw some light on the problems faced by them. 5
A4) The first is team building or "teaming".
- Employees need to know what their stakes are, what the game is, and how it is played. This is often difficult for new mid-career managers who have never fully understood themselves. Remember that your company has a clear vision and can support it by communicating it well and often, either as a new manager or with a new manager who reports to you. The CEO should be able to tell a great story about what the company has become what the company represents, where it wants to go, and what the company honestly values. HR should help new employees understand why this company exists and its purpose. Administrators need to enhance this message as well as during on boarding.
Does everyone in your organization understand your company's business goals, unique sales positions, brand commitments, operational environment, and market reality?
2. Employees need to understand how to make the most contribution to their vision. Not all employees need to agree to the vision, but they need to be able to tailor their work to the goals of the institution. Performance assessments should be conducted with a view to contributing to these goals. As a manager, you may need to lower your vision from 30,000 feet to a more targeted level. Let your staff know how their work fits into the big picture of your organization and your consumers, clients, or other audience.
3. Employees need a reason to care about their contributions. Salary is not a reason to worry. People need to belong and feel that they are important. Is employee contribution important? Do you care about his contribution? Do you understand how he wants to be rewarded or what makes him feel productive? Are you engaged in your work as a manager? How good are your communication skills in terms of inspiration and gratitude?
4. Managers need to create a positive environment that fosters the traits they want to show to their employees. You may need to experiment a bit here. Some teams have different needs and values. For example, primarily DiSC C-style teams feel rewarded by challenges, but not necessarily personal perceptions. S-style teams value structures that support work-life balance.
5. Employees don't want to feel ready for failure. Do you know what feels like a failure to your employees? Do they have the resources they need to make a full contribution? Are you guessing them second or are you in the way of them? Is it possible that an employee is playing with the old rules because the rules of the game change frequently? How do you, as a manager, deal with failures? Do employees know what your reaction to their failure will be? Will you punish or help them learn?
6. Employees see bad behavior, poor performance overlooked, and no problems. Nothing is more intriguing than seeing a team member fail while others are striving for excellence. Employees "What are they going to do? Will you fire me?" No one has witnessed a rebuke, not to mention the dismissal? Do you have a clear understanding of what constitutes good behavior and good performance? Are you a manager who models both?
7. Employees may be ignored or not evaluated. Do you understand what kind of attention it is beneficial to each employee or team? Do you understand what each employee needs to be grateful, or do managers act on their own tastes?
8. Both managers and employees need continuing education. Without the opportunity for continuous learning, skill gaps can grow rapidly. It's not always possible to hire new skills, and it can be wasteful not to invest in people who are already there. It is important to adopt new technologies such as just-in-time learning. Mentoring and coaching are valuable throughout everyone's career and can help reduce turnover.
Q5) What is team management? Write some challenges in designing an information security policy. 5
A5) Are you planning to start a business, or are you ready for a managerial profile and confused about how to manage individuals with different skills, trends, lifestyles, and belief systems? Second, team management strategies and their proper implementation help motivate all team members to achieve common or organizational goals.
This post introduces the world of team management, how to incorporate the importance, type, and effective team management strategy of team management, and channel individual teams to get the most out of their assigned tasks. Helps you understand. Accuracy and effectiveness in a results-oriented way.
The parable of two sons, his father, and a bunch of sticks taught us that we are united and strong very early in our lives.
A bunch of rods cannot be easily broken, but a single rod can be easily broken. Similarly, working as a team creates more workforce than taking over goals with one hand.
Today, many organizations focus on employee harmony by paying attention to the quality of good leadership to perform the desired work as needed.
If someone understands the importance of teamwork as part of project management, you can improve the overall performance of your company. There are many important steps to achieving functional team management. Continue reading every corner of team management and leadership.
Team management is the idea that groups of people work together to achieve a common goal. The top leaders will lead this team.
Leaders need to manage the needs and satisfaction of their employees. This inevitably improves work performance and results in profitable results. Team management is the collective effort of colleagues to reach the desired organizational skills needed to accomplish a team's mission.
Team managers assign projects or day-to-day tasks, prioritize opinions as well, and discuss issues in open forums. It's also important to ensure that employees don't feel left behind and avoid potential conflicts while working.
Information security policies aim to keep your organization's data safe. However, designing an effective information security policy is not easy. The rapid evolution of technology poses new threats every day, and most policies need to be implemented across multi-user organizations.
Policy design faces many challenges that need to be overcome. These challenges arise not only from external factors, but also from internal discrepancies. Find out about some of the challenges in designing an information security policy.
- Non-compliance
Designing an information security policy that is enforceable but has command compliance is a major challenge. If an employee decides not to comply with the policy, the employee can pose a significant threat to the security of the organization. Common reasons for employee non-compliance include the impact of policies on productivity, forgetting, and uncontrolled behaviour. Whatever the reason for the non-compliance, the design and implementation of your organization's policies should discontinue this behaviour.
b. Employee distrust
If the policy is too strong or too protected, it can spread distrust to employees. This can also happen if the employee is not part of the decision-making process. Non-privacy oversight can increase discomfort and negatively impact policy design. Please spend a considerable amount of time assessing this issue before designing your policy.
c. Lack of consciousness
Many organizations do not have training sessions aimed at raising awareness about new policies under design. This can be a major setback to policy design. Lack of awareness means that employees are not trained and willing to follow your policies. Awareness should not be limited to policy design. Employees need to understand the risks to their organization when they use the network illegally. This challenge to policy design is urgent as employees struggle to keep up with changes in the organization.
d. False sense of security
Fostering false security can be more harmful than the security breach itself. If the data is regulated by federal law, the case of data breach may be decided in court. This happens when the policy design lacks robustness. That is, it breaks the security façade. The security policy may be inadequate or improperly enforced. Therefore, designing a truly protective policy is a daily challenge for organizations.
e. Lack of security system updates
Every minute, your organization's data security system faces emerging cyber threats. Designing policies that can respond to rapidly evolving security threats is a crucial challenge. The policy design team must have the foresight to include imminent risks to data security in the policy. In addition, policy design should consider the scope of future policy updates and extensions.
Q6) What is outsourcing? What are the reasons of outsourcing? 5
A6) Outsourcing is the passing of individual tasks, sub-areas, or business processes to a third party, thereby receiving results from outside the company. The services that your company was responsible for will now be provided by a professional service provider. These tasks are often secondary to the business. That is, the tasks that a company needs to perform in order to focus on its core activities.
The term "outsourcing" refers to a strategy in which a company's tasks and structure are given to an external contractor. These can be individual tasks, specific areas, or entire business processes.
Outsourcing typically provides one or more tasks or processes to external partners. However, under certain circumstances, some tasks are performed internally (in-house outsourcing). For example, if you give a task to another area of the company, or a department that specializes in it, this is commonly known as internal outsourcing. In contrast, the task given entirely to an external company is known as external outsourcing. External companies may be region-based or foreign contractors. The focus is on potential cost savings.
What is the reason for outsourcing?
In addition to the expected cost savings of outsourcing, there are other reasons to hand over a particular task.
- Increasing shortages: Enterprises can focus on their core competencies and work more efficiently.
- Optimal Scalability: Outsourcing improves workforce availability. As a result, maximum output can be achieved and production guaranteed, even in the event of seasonal or non-operating capacity fluctuations.
- Respond quickly: You can pass these tasks to a professional third-party company to improve your response to changes.
- Quality Improvement: Outsourcing often results in quality improvement. For example, you can improve the quality of your products by manufacturing good factories and workshops.
- Cost Savings: External companies have a high degree of expertise in services. They offer discounted rates because they can do much more cost-effective work.
- Lack of Know-how: While companies often require new processes and operations, employees often lack the necessary know-how and implementation skills. Outsourcing is an alternative to hiring skilled workers for this purpose.
Q7) State the various forms of outsourcing. Give example. 5
A7) Various forms of outsourcing
There are many forms of outsourcing.
- Business Process Outsourcing: Business process outsourcing outsources the entire process of a company. This example: An external service provider can create salaries for employees.
- Knowledge Process Outsourcing: This type of outsourcing outsources complex tasks to third-party companies. This example may be a search engine optimized text preparation for your website. Each company usually trains highly specialized professionals.
- Out tasks: In this case, only individually defined tasks are handed over to another company. This is often due to time-consuming management processes such as email archiving and data backup. However, the company is responsible for this as the entire business area remains independently managed.
- Selective Outsourcing: Selective outsourcing is a combination of business process outsourcing and out tasking. The individual sub-areas are outsourced and are broader than the individual tasks, but they are still not a complete process.
Outsourcing strategies are used, for example, in customer service, accounting, tax consulting, IT, and marketing departments.
Examples of possible outsourcing strategies:
- Customer Service: A company transfers customer service work to a specialized company. Call centers often take over a particular call capacity for a fixed price.
- Marketing: Companies outsource support for social media channels to external service providers (such as agencies).
- Product Manufacturing: For many fashion companies, manufacturing garments in the United States is too expensive. Therefore, they often choose to produce in Asia. After production is complete, the garment will be shipped to the United States.
These are just a few examples of outsourcing. In fact, today's enterprises can outsource every step of their business, whether manufacturing or servicing, to an outside contractor. Thanks to the networked world, it no longer matters whether foreign companies are in the neighbouring village or on the other side of the world.
Q8) Write the advantages and disadvantages of outsourcing. 8
A8) There are many reasons why organizations choose outsourcing. If anything, outsourcing generally helps businesses increase their productivity and boost their bottom line.
- Outsourcing as an efficient business strategy
To better understand how this practice can help your organization, here's a list of its benefits.
b. Save money
One of the main reasons many companies outsource is the ability to reduce costs. If you want to hire employees in your company, you need to provide training, resources, and workspaces. Outsourcing is less expensive than if you had to hire a full-time staff member. It also saves time in the hiring process and on boarding.
c. Veteran talent
Outsourcing providers have a pool of industry professionals with different experiences because they need to work with clients from different industries. Offshore outsourcing in particular gives you the opportunity to collaborate with global talent at a lower cost.
d. Access to the latest technology
In addition to highly specialized staff, outsourcing vendors are investing in the type of technology they use because they are part of their core business. It also serves as a competitive advantage among other third-party service providers.
On your part as a client, you need to take advantage of better technology and world-class equipment to optimize your day-to-day operational capabilities. Therefore, this improves profitability.
e. Focus on core business functions
Organizations tend to overlook core features as they grow their business. Outsourcing some of your operational tasks frees you and your staff more time to focus on the more important parts of your business.
f. Crisis management
Outsourcing companies provide talent with specific areas of expertise, reducing the risk of poor employee performance. In addition, you can continue your day-to-day work, especially when employee turnover peaks.
Disadvantages of outsourcing
Outsourcing brings many benefits to the market industry, but there are still companies sceptical about doing so. Keep in mind that outsourcing also has its downsides. As a business owner, it is imperative to recognize the following possible shortcomings before making a decision:
- Out of control
Performing some of the business tasks also means providing these capabilities to outsourcing providers. However, that does not necessarily mean that you have complete control over the subcontracting tasks. Keep in mind that third-party providers have their own methods, whatever they think is appropriate, and must compromise to give them that freedom.
b. Benefits of outsourcing
c. Security risk
When subcontracting with personal data such as customer information, there is always the risk of data privacy issues. Nevertheless, we are now living in an era of data protection. Outsourcing providers ensure the security and security of your client's information. Be sure to include a security clause in your contract or have another non-disclosure agreement signed.
d. Hidden cost
Although outsourcing can save you money, outsourcing providers usually include hidden costs in long-term contracts. That's why you need to discuss costs in advance when negotiating with the vendor of your choice. It is also your responsibility to carefully read and understand their terms before signing their contract, otherwise you may incur unexpected costs.
e. Time zone shift
This is from the perspective of off shoring to countries with different time zones. The biggest challenges here are communication and working hours. When working with offshore staff, you need to coordinate your meetings with a viable time that everyone can communicate effectively.
Fortunately, there are various communication tools available today. These tools not only keep track of offshore tasks and project progress, but also make it easier for you and remote staff to work together.
For some companies, different time zones can also be an advantage. One example of this is that if you are off shoring your contact center, you will have the advantage of being able to serve for 24 hours or even longer.
Q9) What is data quality? What are the features of data quality? Why it is important? 8
A9) Data quality is the ability of data to serve its intended purpose based on seven different characteristics. (If you are already familiar with data quality, move on to its characteristics.)
Before examining these characteristics, let's better understand the concept of data quality.
Definition of data quality
A simple online search will give you countless definitions. After some thought, here's how to define data quality and high-quality data:
Data quality is the answer to the question "What about my data?" Data is of high quality when it is useful for business and decision making.
By the way ... Of all the sources online, the definitions from Thomas C. Redman, "Data Doc", and the author of this Data Driven have been found to be the most relevant.
Data is generally considered to be of high quality if it is "suitable for its intended use in operations, decision making, and planning."
What are the characteristics of data quality?
There are seven factors that play a major role in determining data quality.
- Accuracy: Is the data accurate, accurate, error-free? Without accuracy, the data can be misleading and useless.
- Availability: Is the right data available to the right people in your organization? In order for a data person to do his job, the data must be available and accessible.
- Integrity: Is the data incomplete? Are you missing some information? Incomplete data leads to information gaps that make it difficult to use.
- Particle size: What level of detail can the data provide? Accurate and effective decision making requires proper particle size of the data.
- Relevance: Do you know if you really need the information you collect? What is the purpose of the saved data? Unrelated information wastes time, effort and money.
- Reliability: Does the data contain ambiguous, ambiguous, or inconsistent information? In all such cases, the information you have is unreliable and you cannot trust your data.
- Timeliness: Is the data outdated or outdated? Data collected at the right time is an important indicator of data quality. Relying on untimely data can be misleading and lead to inaccurate decisions.
Why is data quality so important?
Poor, inaccurate, incomplete, and unreliable data can result in significant business damage.
Recall that we spent two weeks creating a sales report showing the wins and losses of an opportunity.
On the first day, everything was Hanky Dolly ... Birds were singing and the sun was shining on you and your Excel.
But by day 5, was the weather cloudy and could be a data error?
On the 13th day, I noticed that even the data was unreliable. There was no way to know because I couldn't see the source or the changes that occurred before the data arrived.
After all, the data provided as a separate Excel file never provides the complete context needed to understand the quality of the data.
Result? The goal-achieving process was not adjusted and the numbers did not improve. At least that wasn't the case within the originally planned time frame.
Q10) How can I ensure data quality and maintain high quality data? 5
A10) But first of all, before we can fix the problem, we need to know why it exists in the first place. What is the cause?
- Start from source
When you come up with something, does your doctor simply treat the symptoms, or does she scrutinize you to find the root cause of your illness?
Well, if she's a good doctor, she'll go with the latter, she'll probably ask you to perform some tests to get to the root of the problem.
The same applies to ensuring data quality. Whenever you notice that the quality of your data is poor, you need to spend time finding the following:
How was the data brought into your organization's data repository?
What was your purpose?
Who was the creator / owner of the data?
Who can access it? Why?
Who made any changes / revisions to the above data?
Where was the data used? (Fixing an issue will notify everyone who used it for reporting or decision making)
This may sound like going down a deep, dark rabbit hole, but it's not.
b. Use data quality tools
Once you understand the reason behind the bad data problem, the next step is to fix it. You can do it manually, but it sounds tedious, time consuming, and complicated.
The good news is that there are plenty of data quality tools available that can help you.
In short, data quality tools help you implement DQM within your organization.
These tools play a very important role, so you need to make sure that they completely resolve all issues, from finding issues to fixing them and monitoring policies to prevent them from recurring.
Let's take a look at some of these functions and see how.
c. Data cleaning
Data cleansing (also known as cleansing) is the process of removing erroneous or duplicate entries while fixing suspicious or missing data. Data quality tools can help you detect and correct such entries.
d. Data standardization
Another function key to ensure data quality is data standardization. This helps ensure data consistency. The content and format of each data type is the same.
e. Data profiling
Yet another feature is data profiling, which provides information about the data (metadata + business context). A data catalog with tags, descriptions, READMEs, and business glossaries makes this feature easy.
And they are just a few features. The data quality tool must be an end-to-end product that handles all these features and all the features needed to ensure the quality of the data.
f. Follow data quality best practices
Data quality tools can help you solve bad data problems, but they are not enough. Without proper processes and quality checks, there is no doubt that more data quality issues will soon occur.
But before you frown, the good news is!
We've put together six best practices for data quality that completely solve your problem. It also serves as a brief summary and summary of all that has been described so far. So go here without any more effort:
- Educate everyone in your organization about data quality. Everyone has a role to play when it comes to improving data quality. Get approval from management.
- Make data quality part of data governance, define quality assurance (QA) metrics, and perform regular QA audits.
- Appoint roles such as data owners, data stewards, and data managers within your organization to establish the right processes to ensure high data quality.
- As mentioned above, investigate quality issues in the source.
- Establish a single source of truth (SSOT) for all data.
- Automate your workflow. In particular, the data entry and ETL / ELT workflows are responsible for ingesting, transforming, and organizing data, making it even more usable.
Q11) What is Managing change? What are the steps for an effective change management process? 8
A11) Managing change means managing people's fears. Change is natural and good, but people's reactions to change can be unpredictable and irrational. If done correctly, it can be managed.
Employee changes should be defined in as much detail as possible. We will provide updates as soon as the front end progresses and becomes clear. If your desk needs to be moved, tell your employees what's going on. "We need to attract more workers. Sales are up 40% and we can't meet that demand with a lot of overtime. We need to sort out a bit to make room for them. Yes. ”You can also ask your employees how to relocate the space. You don't have to accept their suggestions, but that's the beginning of understanding.
The definition is bidirectional. In addition to defining the problem, you need to let employees define the reasons behind their resistance.
Eight key steps for an effective change management process
Your organization is constantly undergoing change. Whether due to new technology implementations, process updates, compliance initiatives, reorganizations, or improved customer service, change is constantly needed for growth and profitability. A consistent change management process helps minimize the impact on your organization and staff.
Below are eight key steps to a successful change initiative.
1. Identify what will be improved
Most changes occur to improve a process, product, or outcome, so it's important to focus and clarify your goals. This includes identifying the resources and individuals who drive the process and lead the effort. Most change systems allow knowing what to improve creates a solid foundation for clarity, ease, and successful implementation.
2. Present a solid business case to stakeholders
There are several layers of stakeholders, including senior executives who direct and fund efforts, advocates of the process, and those who are directly responsible for enacting new normals. Everything has different expectations and experiences, and there must be a high level of "agreement" from the entire spectrum. The process of on boarding the various components varies from change framework to framework, but all provide plans that require time, patience, and communication.
3. Change plan
This is a "roadmap" that identifies the starting point, the route to follow, and the destination. Also, integrate the resources, scope or purpose, and costs you want to leverage into your plan. An important element of planning is to provide a multi-step process rather than a sudden unplanned "sweep" change. This includes out lining the project in clear steps with measurable goals, incentives, measurements, and analysis. For example, a well-planned and controlled change management process for IT services can significantly reduce the business impact of IT infrastructure changes. There are also universal precautions to practice patience throughout this process and avoid shortcuts.
4. Provide resources and use data for evaluation
Resource identification and financing are important factors as part of the planning process. These include infrastructure, equipment, and software systems. Also, consider the tools you need to re-educate, retrain, and rethink your priorities and practices. Many models identify data collection and analysis as an underutilized element. Clear progress reporting clarity enables improved communication, proper and timely distribution of incentives, and measurement of success and milestones.
5. Communication
This is a "golden thread" that runs the entire change management practice. Appropriate communication is required to identify, plan, onboard, and execute the appropriate change management plan. There are psychological and sociological realities inherent in group culture. Those who are already involved have established skill sets, knowledge, and experience. But they also have pecking orders, territories, and corporate practices that need to be addressed. Providing a clear and open communication line throughout the process is an important factor in all change modality. These methods advocate transparency and a two-way communication structure that dissipates frustration, praises what is working, and provides a means of seamlessly changing what is not working.
6. Monitor and control resistance, dependencies, and budgeting dangers
Resistance is a regular a part of alternate control, however it is able to threaten the achievement of your project. Most resistance arises due to worry of the unknown. It additionally arises due to the fact there's significant danger related to the alternate. Risks that have an effect on dependencies, go back on funding dangers, and dangers associated with finances allocation to new ones. Predicting and making ready for resistance via way of means of arming management with gear for handling management enables with a clean alternate lifecycle.
7. Celebrate achievement
Recognizing the final results of milestones is an crucial a part of any project. When handling modifications at some stage in the lifestyles cycle, it's miles crucial to be aware about the achievement of the groups and people involved. This enables undertake each the alternate control procedure and the modifications themselves.
8. Review, revision, non-stop development
Changing is tough or even painful; however it is also an ongoing procedure. Even alternate control techniques are commonly coordinated at some stage in the project. As with communication, this desires to be woven into each step to become aware of and put off obstacles. And, as with the want for sources and data, this procedure is as true because the technique to size and analysis.
Q12) What is descriptive analysis? How does descriptive evaluation work? How it is used? 8
A12) Simply put, descriptive analysis is the process of interpreting historical data to understand what happened. This process collects information with the goal of defining specific events and revealing available patterns and trends.
Descriptive analytics is the most common and fundamental form of analytics that companies use. Every part of the business can use descriptive analytics to keep tabs on operational performance and monitor trends. Examples of descriptive analytics include KPIs such as year-on-year percentage sales growth, revenue per customer and the average time customers take to pay bills. The products of descriptive analytics appear in financial statements, other reports, dashboards and presentations.
Most companies accumulate vast amounts of data, but it’s often impossible to understand what the data means without performing some analysis. For example, examining thousands of individual sales transactions for the latest quarter doesn’t tell you the average amount customers spent or whether total sales were higher or lower than in previous periods. Descriptive analytics is the first step in making sense of that raw data. It often uses basic mathematical operations to produce summary statistics — such as average revenue per customer — to get a better handle on the current state of affairs with your business. Once companies identify trends, they can use other types of analysis to delve deeper into the causes and consequences.
How does descriptive evaluation work?
To examine facts, agencies should first gather uncooked facts from plenty of reassets, mixture it, and rework it right into a not unusual place layout for evaluation. Now you're geared up to research your facts. Many agencies use facts intelligence, or a fixed of strategies and equipment used to gather and examine facts, to attract conclusions and movement plans primarily based totally on their findings. You also can use spreadsheet formulation to use simple descriptive evaluation to aggregated facts to generate KPIs and different facts for inclusion to your reviews.
The included ERP suite makes descriptive evaluation tons less difficult due to the fact you could save all of your organization's enterprise facts in a unmarried database. The essential suite additionally consists of integrated analytic equipment to help in storytelling the facts. This is the act of the usage of visualizations to create an outline of statistics and proportion the which means in the back of the facts in a compelling way. Business intelligence skills constructed into ERP can use real-time facts constructed into dashboards, charts, and reviews to offer not unusual place KPIs.
How is descriptive evaluation used?
Companies use descriptive evaluation in lots of components in their enterprise to evaluate how nicely their enterprise is appearing and the way nicely they're doing to attain their enterprise desires. Business leaders and monetary experts music not unusual place monetary signs generated via way of means of descriptive evaluation, which include quarterly boom in sales and expenses. Marketing groups use descriptive evaluation to music marketing campaign overall performance via way of means of tracking metrics which include conversion quotes and the range of social media followers. Manufacturing businesses reveal signs which include manufacturing line throughput and downtime.
The metrics generated via way of means of the descriptive evaluation are utilized in plenty of ways, including:
- Report: The key monetary signs contained in a organization's monetary statements are generated via way of means of descriptive evaluation. Other widespread reviews additionally use descriptive evaluation to focus on elements of overall performance.
- Visualization: Displaying metrics in graphs and different picture representations permits you to effectively speak their effect to greater users.
- Dashboards: Executives, managers, and different personnel can use dashboards to music development and manipulate their every day workloads. The dashboard suggests a choice of KPIs and different vital statistics tailor-made to every individual's needs. Information may be represented as charts or different visualizations in order that humans can soak up it greater quickly.
Q13) Write the steps for descriptive evaluations. Give examples. 5
A13) Five steps of descriptive evaluation
The software of descriptive evaluation normally starts off evolved with defining the metrics to generate and ends with offering them withinside the favoured layout. Here are the stairs to comply with to generate your personal descriptive evaluation:
- State Business Indicators: The first step is to pick out the signs you need to generate. These have to mirror the important thing enterprise desires of every organization or organization as a whole. For example, a boom-minded organization makes a speciality of measuring quarterly sales boom, and an organization's debts receivable organization tracks unpaid days and different metrics that mirror the time it takes to gather cash from customers. You can also additionally need to.
- Identify the facts you want: Find the facts you want to generate the metrics you want. In a few agencies, the facts can be unfold throughout a couple of packages and files. Companies the usage of ERP structures can also additionally have already got maximum or all the facts they want withinside the device's database. Some metrics may require facts from outside reassets which include enterprise benchmark databases, e-trade websites, and social media platforms.
- Extracting and Preparing Data: If the facts is from a couple of reassets, extracting, combining, and making ready the facts for evaluation is a time-eating however vital step to make sure accuracy. .. This step can also additionally encompass facts cleaning to cast off inconsistencies and mistakes withinside the facts from diverse reassets, and reworking the facts right into a layout appropriate for analytical equipment. Advanced types of facts evaluation use a manner known as facts modelling to assist prepare, structure, and arrange company statistics. Data modelling is a framework inside a statistics device for outlining and formatting facts.
- Analyzing facts: Companies can practice descriptive analytics the use of quite a few tools, from spreadsheets to commercial enterprise intelligence (BI) software. Descriptive evaluation regularly includes making use of fundamental mathematical operations to 1 or greater variables. For example, a income supervisor may also need to tune common sales in step with sale or month-to-month sales from new customers. Executives and economic experts may also need to display economic signs which includes gross margin (gross margin).
- Presenting facts: Presenting facts in a compelling visible format, which includes pie charts, bar charts, and line charts, is regularly less complicated for stakeholders to understand. However, a few people, along with economic professionals, may also need to look the statistics offered as numbers or tables.
Example of descriptive evaluation
Examples of descriptive evaluation exist in each component of the commercial enterprise, from finance to manufacturing and income, along with:
Business reviews on sales and expenses, coins flow, bills receivable and bills payable, stock and manufacturing.
Financial signs and different commercial enterprise KPIs are examples of descriptive evaluation. These consist of signs that examine the fitness and cost of your commercial enterprise, which includes price-profits ratio, modern-day ratio, and go back on invested capital.
Social Media Engagement: Descriptive evaluation produces metrics that assist decide the sales of social media initiatives, which include follower growth, engagement rates, and sales from a specific social media platform.
Survey: Descriptive evaluation produces a précis of inner and outside survey results, which includes the internet promoter score.
Q14) What is Predictive analytics? State its types. 5
A14) Predictive analytics uses mathematical modelling tools to generate predictions about unknown facts, characteristics, or events. "It's about taking data that is known to exist and building a mathematical model from that data to help predict someone [or something] that isn't yet in that dataset." Goulding explains.
The role of analysts in predictive analytics is to collect and organize data, identify the type of mathematical model that applies to the case at hand, and draw the necessary conclusions from the results. They are also often tasked with communicating those conclusions effectively and attractively to stakeholders.
Types of prediction models
The data analyst needs to decide which mathematical model to use in a particular situation, but it does not actually process the data. Statisticians and programmers develop computer programs that perform these processes. Each of these processes works with a different mathematical model.
"The tools we use for predictive analytics have been improved and are much more sophisticated," Goulding explained, and these advanced models "make it possible to process large amounts of data in ways never before possible." It became. "
Advances in these tools have also led to the use of predictive analytics to identify previously unmanageable "unknowns", concisely identifying which model best matches the type of unknown in each scenario. I needed an analyst who could do it overall.
The following describes four common predictive models and the types of questions they can use to answer best.
1. Linear regression
According to Goulding, linear regression is one of the most famous and historic modelling tools. This model considers all known data points on the graph and creates a straight line that passes through the center of those data points. This line represents the minimum possible distance between all points on the graph. Mathematical modelling tools for linear regression can make predictions about non-existent data based on the relationship between this line and existing data points.
2. Text mining
Linear regression uses only numerical data, but mathematical models can also be used to make predictions about non-numerical factors. Text mining is a perfect example.
“Text mining is part of predictive analytics in the sense that analysis is finding information that we didn't know before,” says Goulding. In this scenario, the tool retrieves data points in the form of text-based words or phrases and searches a huge database for those specific points.
3. Optimal quote
Optimal estimation is a modelling technique used to make predictions based on observed factors. This model has been used in analytics for over 50 years and has laid the foundation for many other forecasting tools in use today. According to Goulding, past applications of this method include the determination of "how to optimally recalibrate equipment on the shop floor ... [and] how to estimate where to go when a bullet is shot", and the defence industry. Includes other aspects.
4. Clustering model
The clustering model focuses on finding different groups of similar qualities or elements in the data. Many mathematical modelling tools fall into this category, including:
K-Means
Hierarchical clustering
Two stages
Density-based scan clustering
Gaussian clustering model
Kohonen
5. Neural network
Neural networks are complex algorithms inspired by the structure of the human brain. It processes past and present data, identifying complex relationships within the data and predicting the future, similar to how the human brain finds trends and patterns.
A typical neural network consists of artificial neurons called units, which are arranged in various layers. Neural networks use input units to learn and process data. The output unit, on the other hand, is on the other side, outlining how the neural network responds to the input unit. There is a hidden layer between the two. This is a layer of math functions that produces a particular output.
Q15) Why Predictive analytics is important? Give examples. 8
A15) Following are the reasons that proofs that Predictive analytics is important:
- Rise of big data
Predictive analytics is often discussed in the context of big data. For example, engineering data comes from sensors, equipment, and connected systems around the world. A company's business system data may include transaction data, sales results, customer complaints, and marketing information. Increasingly, companies are making data-driven decisions based on this valuable pile of information.
- Intensifying competition
As competition intensifies, companies want to gain an edge in delivering products and services to crowded markets. Data-driven forecasting models help businesses solve long-standing problems in new ways.
For example, equipment manufacturers may find it difficult to innovate with hardware alone. Product developers can add predictive capabilities to their existing solutions to add value to their customers. Predictive analytics can be used for equipment maintenance or predictive maintenance to predict equipment failures, predict energy demand, and reduce operating costs. For example, sensors that measure the vibration of auto parts can signal the need for maintenance before a vehicle breaks down on the road.
Enterprises also use predictive analytics to create more accurate forecasts, such as forecasts for power grid power demand. These forecasts allow for more effective resource planning (for example, scheduling of different power plants).
b. State-of-the-art technology for big data and machine learning
To derive value from big data, companies use tools such as Hadoop and Spark to apply algorithms to large datasets. The data source may consist of transactional databases, equipment log files, images, video, audio, sensors, or other types of data. Innovation often comes from combining data from multiple sources.
We need tools to use all this data to extract insights and trends. Machine learning techniques are used to find patterns in data and build models that predict future outcomes. A variety of machine learning algorithms are available, including linear and non-linear regression, neural networks, support vector machines, decision trees, and other algorithms.
Predictive analytics example
Predictive analytics supports teams in a variety of industries, including finance, healthcare, pharmaceuticals, automotive, aerospace, and manufacturing.
Automobiles – Breaking new ground with self-driving cars
Companies developing driving assistance technologies and new self-driving cars use predictive analytics to analyze sensor data from connected vehicles and build driving assistance algorithms.
Aerospace-Monitoring the condition of aircraft engines
To improve aircraft uptime and reduce maintenance costs, engine manufacturers have created real-time analytics applications that predict oil, fuel, lift-off, mechanical condition, and control subsystem performance.
Energy Production-Electricity Price and Demand Forecast
Sophisticated forecasting apps use models to monitor plant availability, past trends, seasonality, and weather.
Financial Services-Development of Credit Risk Model
Financial institutions use machine learning techniques and quantitative tools to predict credit risk.
Industrial Automation and Machinery-Prediction of Machine Failure
Producers of plastics and thin films save € 50,000 each month with health monitoring and predictive maintenance applications that reduce downtime and minimize waste.
Medical Devices – Discover Asthma and COPD Using Pattern Detection Algorithms
The asthma management device records and analyzes the patient's breath sounds and provides immediate feedback via a smart phone app to help the patient manage asthma and COPD.
Q16) What is Predictive modelling? Write its types. 5
A16) Predictive modelling is the process of analyzing current and known information to predict future results. Predictive analytics uses predictive modelling algorithms to capture possible future results.
At the peak of data science, predictive modelling has emerged as a convenient data mining technique that allows organizations and businesses to extract forecast results based on currently known data.
The predictive modelling process records data, applies statistical models or algorithms, and predicts future outcomes. In this way, successful predictive analytics validates various models and algorithms.
With the help of artificial intelligence techniques and machine learning algorithms, predictive modelling has become part of data mining over time. Not only did it help gain insight into future potential, but it also influenced the decision-making process in a more accurate and enhanced way. Predictive modelling has greatly improved the role of technology in business and the role of AI in business.
Predictive modelling is just the best machine learning tool for organizations that want to get the predicted results for their current progress. That said, let's learn more about predictive modelling.
Even if this concept has been practiced for more than half a century, it has just recently gained worthy importance from day one. In the early days, we were investigating the effectiveness of this data science technology, but nowadays industries and organizations have successfully implemented it.
In addition, it linked historical data with future assumptions, facilitated decision-making, and strengthened the capabilities of the institution.
Predictive analytics method
Predictive modelling is defined as a predictive analytics tool for leveraging historical data to extract future results, but it can also be thought of as a mathematical procedure used to calculate future potential.
Predictive modelling, also known as predictive analytics, is a variety of predictive models. Tell me more about the same thing.
- Classification model
Of all the predictive modelling techniques in machine learning, the classification model is one of the most widely used techniques. In classification predictive modelling, inputs are categorized into specific categories, treated as labels, and their classes are predicted.
In predictive modelling, common data points are inserted into the software to classify inputs and predict classes of outputs.
2. Predictive model
One of the most common and accurate forecast models, the forecast model is used to predict / predict metric values based on historical data. The predictive model uses historical data to calculate numerical data points and assign values to them based on the historical data.
Like data analysis, predictive analytics algorithms also take into account the nature of historical data to help predictive modelling tools predict future results.
3. Outlier model
Outlier models revolve around detecting outliers in datasets or outliers. Simply put, outlier models are one type of predictive model that helps detect anomalies in a dataset and predict relevant information for a particular dataset.
Especially in the financial arena, outlier models are useful for predictive modelling to detect whether a transaction is fraudulent or secure.
“For example, when identifying fraudulent transactions, the model can evaluate not only the amount, but also the location, time, purchase history, and nature of the purchase.” Predictive analytics algorithm
Q17) State the Predictive Modelling tool? Write Predictive Modelling applications. 8
A17) Predictive modelling tool
- Oracle Data Science
Oracle Data Science is a leading predictive analytics tool that incorporates state-of-the-art machine learning algorithms for performing predictive modelling on different datasets using AI.
Some of the main features of this tool are to provide a high quality platform for building modern algorithms, to provide better results and to provide reliable results. Oracle Data Science uses built-in machine learning algorithms to provide other services such as enhanced business analytics, comprehensive decision making, and improved accuracy of results.
Apart from predictive analytics in the business, the platform also provides other artificial intelligence technology tools such as storage, networking and cloud computing.
2. H2O
H2O, the leading platform for data analysis, is a predictive modelling platform that supports a variety of sectors such as healthcare, finance, marketing and telecommunications.
H2O aims to democratize artificial intelligence and enable it to be used efficiently by people around the world by implementing predictive modelling algorithms on world-renowned clients.
As the use of machine learning tools and algorithms increases in almost every sector, the platform incorporates the ability to model and customize tools according to your needs.
3. Q Research
An advanced data analysis tool, Research is a popular platform for predictive modelling because it can leverage patterns established by historical data records to capture future results and possibilities.
Apart from predictive modelling, the platform also provides big data analytics services that enhance your organization's day-to-day operations and operations.
Simply put, Q Research is a comprehensive guide to market research for anyone who is willing to implement AI-driven methods for business analytics.
Predictive modelling application
Now that you've already learned about predictive modelling in the previous segment, you'll now learn about different areas, including this data analysis technique, for better decision-making processes and operational activities. Now let's discover a real-world predictive modelling application.
- Sale
Sales are one of the most important aspects of a business to keep it going. Based on the types of sales the company has achieved in the past, predictive analytics techniques and tools can very well establish the future of the company in terms of sales and profits.
In addition, you can detect anomalies where your sales department is late. This will improve your company's performance in specific areas or demographic circles.
b. Marketing
Another application of predictive analytics is marketing. Because marketing is the act of promoting a particular service or product to a group of targeted customers, you need to anticipate the customer's reaction and predict the customer's requirements based on the data collected from the customer's feedback.
Here, historical data is accumulated and analyzed accordingly, giving way to future results and predicting the type of future service customers want.
c. Social media
Social media is an unstructured, heterogeneous, vast data hub.
Social media, a platform that millions of people use to interact with the Internet on a daily basis, requires predictive modelling to anticipate customer feedback and determine the type of response on a product or platform. ..
However, the importance of social media and it is one of the widely used applications of predictive modelling that helps different platforms detect customer activity and calculate future results accordingly.
d. Risk assessment
The main use of predictive modelling is risk assessment. Risk assessments are common in financial institutions and fraud detection cases, and you may want to assess the type of risk.
Based on data analysis of historical records, predictive analytics tools help individuals, businesses, or organizations perform risk assessments to determine the depth of risk or benefit they see in the future.
In addition, risk assessment and predictive modelling can indicate the types of risk an action can pose to a company. Nevertheless, predictive modelling is one of the most efficient methods of risk assessment in an organization.
e. Quality improvement
The last of all predictive analytics applications is quality improvement. This is somehow related to the use of predictive modelling in the field of marketing.
It is based on how the product or service receives response or feedback from customers over time. Quality improvement involves reviewing past feedback, improvisation, and recommendations that may lead to quality improvement for your institution or company.
In addition, quality improvements also include adapting improvised versions of the same service / product and assessing the type of response obtained. Perhaps predictive modelling can be very useful in the area of quality improvement.
Q18) What is data mining? Explain its types. 8
A18) Data mining is one of the most useful techniques to help entrepreneurs, researchers, and individuals extract valuable information from vast datasets. Data mining is also known as Knowledge Discovery (KDD) in the database. The knowledge discovery process includes data cleaning, data integration, data selection, data transformation, data mining, pattern evaluation, and knowledge presentation.
The Data Mining Tutorial includes all data mining topics such as applications, data mining and machine learning, data mining tools, social media data mining, data mining techniques, data mining clustering, and data mining challenges.
The process of extracting information and identifying patterns, trends, and useful data that enable businesses to make data-driven decisions from large datasets is called data mining.
In short, data mining is useful for exploring hidden patterns of information from different perspectives and collecting and collecting in specific areas such as data warehousing, efficient analytics, and data mining algorithms to assist in decision making. It can be said that it is a process of classifying into data. Decision-making and other data requirements to ultimately reduce costs and generate revenue.
Data mining is the act of automatically searching large stores of information for trends and patterns that go beyond simple analytical procedures. Data mining utilizes complex mathematical algorithms on data segments to assess the probability of future events. Data mining is also known as data discovery (KDD).
Data mining is the process that organizations use to extract specific data from large databases and solve business problems. It mainly transforms raw data into useful information.
Data mining is similar to data science, where people perform purposefully on a particular dataset in a particular situation. This process includes various types of services such as text mining, web mining, audio and video mining, image data mining, and social media mining. This is done through simple or very specific software. Outsourcing data mining allows you to do all your work faster with lower operational costs. Professional companies can also use new technologies to collect data that cannot be found manually. There is a lot of information available on different platforms, but little knowledge is accessible. The biggest challenge is to analyze the data to extract important information that can be used to solve problems and develop the company. There are many powerful tools and techniques available to mine data and find better insights from it.
Types of data mining
Data mining can be performed on the following types of data:
- Relational database:
A relational database is a collection of datasets that are formally organized by tables, records, and columns, and you can access your data in different ways without knowing the database tables. Tables convey and share information, facilitating data retrieval, reporting, and organization.
b. Data warehouse:
Data warehousing is a technology that collects data from different sources within an organization to provide meaningful business insights. Huge amounts of data come from multiple locations, such as marketing and finance. The extracted data is used for analytical purposes and is useful for corporate organization decision making. Data warehouses are designed for data analysis, not transaction processing.
c. Data repository:
A data repository usually points to a destination for data storage. However, many IT professionals use this term more explicitly to refer to a particular type of setup within an IT structure. For example, a group of databases in which an organization holds different types of information.
d. Object relational database:
The combination of an object-oriented database model and a relational database model is called an object-relational model. Supports classes, objects, inheritance, and more.
One of the main objectives of the object-relational data model is to bridge the gap between relational databases and object-oriented model techniques often used in many programming languages such as C ++, Java, and C #. Is.
e. Transaction database:
A transactional database is a database management system (DBMS) that can undo a database transaction if it is not executed properly. This has been a unique feature for a very long time, but today most relational database systems support transactional database activity.
Q19) What is data mining? State the advantages and disadvantages of it. 8
A19) Data mining is one of the most useful techniques to help entrepreneurs, researchers, and individuals extract valuable information from vast datasets. Data mining is also known as Knowledge Discovery (KDD) in the database. The knowledge discovery process includes data cleaning, data integration, data selection, data transformation, data mining, pattern evaluation, and knowledge presentation.
The Data Mining Tutorial includes all data mining topics such as applications, data mining and machine learning, data mining tools, social media data mining, data mining techniques, data mining clustering, and data mining challenges.
The process of extracting information and identifying patterns, trends, and useful data that enable businesses to make data-driven decisions from large datasets is called data mining.
In short, data mining is useful for exploring hidden patterns of information from different perspectives and collecting and collecting in specific areas such as data warehousing, efficient analytics, and data mining algorithms to assist in decision making. It can be said that it is a process of classifying into data. Decision-making and other data requirements to ultimately reduce costs and generate revenue.
Data mining is the act of automatically searching large stores of information for trends and patterns that go beyond simple analytical procedures. Data mining utilizes complex mathematical algorithms on data segments to assess the probability of future events. Data mining is also known as data discovery (KDD).
Data mining is the process that organizations use to extract specific data from large databases and solve business problems. It mainly transforms raw data into useful information.
Data mining is similar to data science, where people perform purposefully on a particular dataset in a particular situation. This process includes various types of services such as text mining, web mining, audio and video mining, image data mining, and social media mining. This is done through simple or very specific software. Outsourcing data mining allows you to do all your work faster with lower operational costs. Professional companies can also use new technologies to collect data that cannot be found manually. There is a lot of information available on different platforms, but little knowledge is accessible. The biggest challenge is to analyze the data to extract important information that can be used to solve problems and develop the company. There are many powerful tools and techniques available to mine data and find better insights from it.
Benefits of information mining
Data mining generation permits companies to gather know-how-primarily based totally information.
Data mining permits companies to make favourable operational and manufacturing changes.
Compared to different statistical information applications, information mining is fee effective.
Data mining helps an agency's decision-making procedure.
Facilitates automated detection of hidden styles and prediction of developments and behaviours.
This may be induced now no longer most effective on current platforms, however additionally on new systems.
These are a quick procedure that permits new customers to quick and without difficulty examine tremendous quantities of information.
Disadvantages of information mining
An agency can also additionally promote beneficial client information to different companies for money. According to the report, American Express offered its clients' credit score card purchases to different companies.
Much information mining evaluation software program is tough to perform and calls for earlier schooling to paintings.
Different information mining gadgets paintings otherwise due to the one-of-a-kind algorithms used of their designs. Therefore, deciding on the proper information mining device may be a frightening task.
Data mining strategies are erroneous and might have critical results beneath sure conditions.
Q20) Where can you use data mining? Write the challenges in data mining. 8
A20) Data mining is mostly utilized by companies with robust customer demands, including retail, telecommunications, finance, and advertising organizations, to price, customer preferences, product positioning, and sales, client satisfaction, and company profits. Determine the effect. Data mining permits shops to apply point-of-sale statistics of client purchases to expand merchandise and promotions that assist companies appeal to clients.
These are the subsequent regions wherein information mining is broadly used:
- Data mining in fitness care:
Data mining in healthcare has super ability to enhance the healthcare machine. Use information and analytics to become aware of great practices that offer higher insights, decorate fitness care services, and decrease costs. Analysts use information mining techniques including device studying, multidimensional databases, information visualization, gentle computing, and statistics. Data mining may be used to are expecting sufferers in every category. The system guarantees that the affected person gets extensive care on the proper location and on the proper time. Data mining additionally permits healthcare organizations to understand fraud and abuse.
b. Data mining in marketplace basket evaluation:
Market basket evaluation is a hypothesis-primarily based totally modelling method. When you purchase a product from one group, you're much more likely to shop for a product from some other group. This method can also additionally assist shops recognize the client's shopping for behavior. This information can assist shops recognize client necessities and alternate save layouts accordingly. It may be completed the usage of one-of-a-kind analytical comparisons of effects among one-of-a-kind shops and among clients in one-of-a-kind demographic groups.
c. Data mining in education:
Educational information mining is a rising subject associated with the improvement of technology for exploring know-how from information generated from instructional environments. EDM's dreams are diagnosed as figuring out students' destiny studying behavior, analyzing the effect of tutorial support, and selling studying science. Organizations also can use information mining to make correct selections and are expecting scholar outcomes. As a result, establishments can recognition on what they educate and the way they educate.
d. Data mining in production engineering:
Knowledge is the great asset owned via way of means of a manufacturer. Data mining gear assist you locate styles in complicated production processes. Data mining may be utilized in machine-degree designs to seize relationships among product architectures, product portfolios, and client information needs. It also can be used to be expecting product improvement time, costs, and expectations, amongst different tasks.
e. Data mining in CRM (Customer Relationship Management):
Customer dating management (CRM) is all approximately obtaining and maintaining clients, strengthening client loyalty, and imposing client-orientated strategies. Companies want to gather and examine information which will construct true relationships with their clients. Data mining generation permits you to apply the gathered information for evaluation.
f. Data mining in fraud detection:
Fraud can fee billions of dollars. Traditional techniques of fraud detection are a chunk slower and greater sophisticated. Data mining offers significant styles and transforms information into information. The best fraud detection machine has to guard the information of all customers. The monitored approach includes a set of pattern statistics, which might be labelled as rogue or non-illegal. This information is used to construct a version and create a method for figuring out whether or not a file is incorrect.
g. Data mining in lie detectors:
Arresting a criminal is not a big deal, but getting the truth out of him is a daunting task. Law enforcement agencies may use data mining technology to investigate crimes, monitor communications of suspected terrorists, and more. This technique also includes text mining, looking for meaningful patterns of data that are usually unstructured text. The information gathered from previous surveys is compared to build a model of lie detection.
h. Data Mining Financial Banking:
The digitization of banking systems is expected to generate huge amounts of data for each new transaction. Data mining technology is a banking and financial technology that identifies business information and market cost trends, casualties, and correlations that are not immediately visible to managers and executives because of too much or generated data. Help bankers solve business-related problems Displaying on screen by professionals is too fast. Administrators can find these data to better target, acquire, retain, segment, and retain profitable customers.
Data mining is very powerful, but it faces many challenges during execution. Various challenges can be related to performance, data, methods, methods, and so on. The data mining process takes effect when an issue or problem is correctly identified and resolved appropriately.
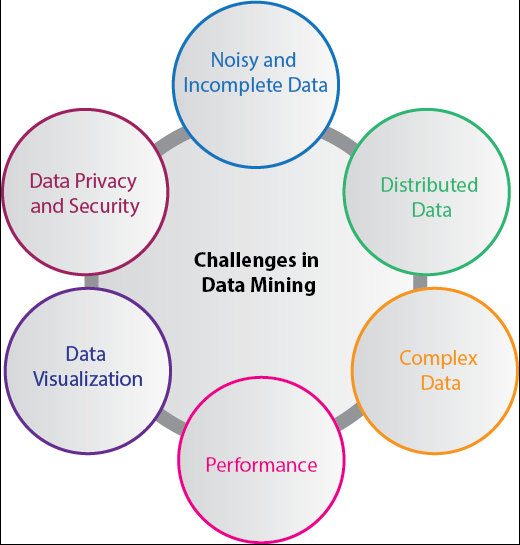
Incomplete and noisy data:
The process of extracting useful data from large amounts of data is data mining. Real-world data is heterogeneous, incomplete, and noisy. Large amounts of data are usually inaccurate or unreliable. These issues can be caused by data instruments or human error. Suppose a retail chain collects phone numbers for customers who spend more than $ 500 and an accountant enters that information into the system. If you enter the wrong number when entering the phone number, the data may be inaccurate. Even some customers are willing to disclose their phone numbers, resulting in incomplete data. Data can change due to human or system errors. All of these results (noisy and incomplete data) make data mining difficult.
Data distribution:
Real-world data is typically stored on different platforms in a distributed computing environment. It can be in a database, on an individual system, or on the Internet. In practice, creating all the data in a centralized data repository is a daunting task, primarily due to organizational and technical concerns. For example, offices in different regions may have servers for storing data. It is not practical to store all the data from all the offices on a central server. Therefore, data mining requires the development of tools and algorithms that enable the mining of distributed data.
Complex data:
Real-world data is heterogeneous and can be multimedia data, including audio and video, images, complex data, spatial data, time series, and more. Managing these different types of data and extracting useful information can be a daunting task. In most cases, new technologies, new tools, and methodologies need to be improved to obtain specific information.
Performance:
The performance of data mining systems depends primarily on the efficiency of the algorithms and techniques used. If the designed algorithms and methods do not meet the standards, the efficiency of the data mining process will be adversely affected.
Data privacy and security:
Data mining usually poses serious problems in terms of data security, governance and privacy. For example, analyzing the details of a product purchased by a retailer reveals data about purchasing habits and preferences without the customer's permission.
Data visualization:
Data visualization is a very important process in data mining. This is because it is the primary way to display the output in a way that looks good to the user. The extracted data must convey the exact meaning of what you are trying to represent. However, it is often difficult to represent information to end users in an accurate and easy way. Input and output information is complex, very efficient, and you need to implement and succeed in a successful data visualization process.
Q21) What are the data mining techniques? 5
A21) Depending at the unique techniques and technology from the intersection of device getting to know, database management, and statistics, statistics mining experts have a profession in higher knowledge a way to system sizable quantities of statistics and draw conclusions. But what's the technique they use to gain it?
Recent statistics mining initiatives have evolved and used quite a few key statistics mining strategies including association, class, clustering, prediction, sequential styles, and regression.
1. Classification:
This approach is used to gain critical and applicable records approximately statistics and metadata. This statistics mining approach enables you classify your statistics into unique classes.
Data mining technology may be labelled with the aid of using numerous standards as follows:
Classification of statistics mining frameworks in keeping with the kind of mined statistics source:
This class relies upon at the kind of statistics being processed. For example, multimedia, spatial statistics, textual content statistics, time collection statistics, the World Wide Web, and so on.
Classification of statistics mining frameworks with the aid of using associated database:
This class primarily based totally at the applicable statistics model. For example. Object-orientated databases, transactional databases, relational databases, etc.
Classification of statistics mining frameworks with the aid of using kind of expertise determined:
This class relies upon at the kind of expertise determined or the statistics mining talents. For example, a few frameworks, including identification, class, clustering, and characterization, have a tendency to be broader frameworks that offer numerous statistics mining talents together.
Classification of statistics mining frameworks in keeping with the statistics mining era used:
This class is primarily based totally at the statistics evaluation processes used, including neural networks, device getting to know, genetic algorithms, visualization, statistics, statistics warehousing or database orientation.
Classification also can don't forget the extent of consumer interplay related to statistics mining procedures, including query-pushed systems, self sustaining systems, and interactive discovery systems.
2. Clustering:
Clustering is the department of records into businesses of linked objects. Writing statistics in numerous clusters particularly loses positive drawback details, however upgrades are achieved. Model the statistics for every cluster. Data modelling locations clustering from a historic attitude rooted in statistics, mathematics, and numerical evaluation. From a device getting to know attitude, clusters relate to hidden styles, cluster seek is unsupervised getting to know, and next frameworks constitute the idea of statistics. From a sensible factor of view, clustering performs a first-rate function in statistics mining packages. Examples encompass clinical statistics retrieval, textual content mining, records retrieval, spatial database packages, CRM, internet evaluation, computational biology, and scientific diagnostics.
In different words, clustering evaluation is a statistics mining approach for figuring out comparable statistics. This approach enables you apprehend the variations and similarities among your statistics. Clustering may be very just like class, however you want to organization chunks of statistics primarily based totally on similarity.
3. Regression:
Regression evaluation is a statistics mining system used to perceive and examine relationships among variables because of the presence of different elements. Used to outline the chance of a selected variable. Regression, particularly withinside the shape of making plans and modelling. For example, it is able to be used to be expecting unique expenses relying on different elements including availability, purchaser demand, and competition. It normally indicates the precise dating among or extra variables in a selected dataset.
4. Association guidelines:
This statistics mining approach enables you locate hyperlinks among or extra gadgets. Find the hidden sample withinside the dataset.
Association guidelines are if-then statements that assist the chance of interplay among statistics gadgets in massive datasets in unique kinds of databases. Association rule mining has numerous packages and is usually used to assist correlate income of statistics or scientific datasets.
Lift:
This size method measures the accuracy of self assurance withinside the frequency with which object B is bought.
(Reliability) / (Item B) / (Whole dataset)
Support:
This size method measures how regularly more than one gadgets are bought and compares it to the complete dataset.
(Item A + Item B) / (entire dataset)
Confidence:
This size technique measures how regularly you purchase object B while you purchase object A.
(Item A + Item B) / (Item A)
5. External detection:
This form of information mining method entails staring at information gadgets in a dataset and does now no longer healthy the predicted sample or behavior. This method may be utilized in a number of domains, together with intrusion, detection, and rogue detection. This is likewise referred to as outlier evaluation or outlier mining. Outliers are information factors that deviate substantially from the relaxation of the dataset. Most of the real datasets have outliers. Outlier detection performs an crucial position withinside the discipline of information mining. Outlier detection is beneficial in a number of areas, together with community interruption identification, credit score or debit card fraud detection, and wi-fi sensor community information outlier detection.
6. Sequential sample:
Sequential styles are information mining strategies focusing on comparing sequential information and detecting sequential styles. It includes locating exciting sub sequences from a hard and fast of sequences, and series stakes may be measured through diverse standards which include length, frequency of occurrence, and so on.
In different words, this information mining method enables you find out or apprehend comparable styles of transactional information over a length of time.
7. Forecast:
Forecasting used an aggregate of different information mining strategies which include trends, clustering, and classification. Analyze beyond activities or times in the precise order to be expecting destiny activities.
Q22) What is Prescriptive analytics? What are its benefits? 5
A22) Prescription analytics is the process of analyzing data and providing immediate recommendations on how to optimize business practices for multiple forecast results. In essence, prescription analysis takes "what we know" (data), comprehensively understands that data to predict what might happen, and moves forward based on informed simulations. We suggest the best steps for.
Prescription analysis is the third and final layer of modern computerized data processing. These three layers are:
Descriptive analysis: Descriptive analysis acts as the first catalyst to make data analysis clear and concise. That's what we know (current user data, real-time data, previous engagement data, big data).
Predictive analytics: Predictive analytics applies mathematical models to current data to inform (predict) future behavior. That is "what can happen".
Prescription analysis: Prescription analysis uses similar modelling structures to predict results and uses a combination of machine learning, business rules, artificial intelligence, and algorithms to simulate different approaches to these many results. Next, we suggest the best action to optimize your business practices. That is "what should happen".
Descriptive analysis is a natural advance from descriptive and predictive analysis procedures. We're taking it one step further to remove guesswork from data analysis. Data scientists and marketers can also save time trying to understand the meaning of the data and the dots they can connect to provide their audience with a highly personalized and relevant user experience
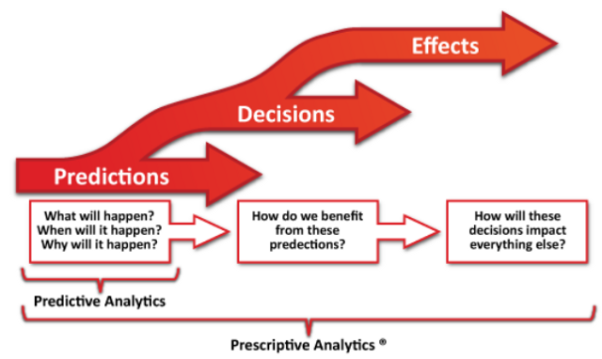
Benefits of prescription analysis
For senior management, we always keep in mind to further optimize the efficiency and success of our organization's operations. Prescription analysis is the smartest and most efficient tool available to provide a foothold for your organization's business intelligence. Prescription analysis enables organizations to:
Easily map the path to success. Prescription analysis models are designed to bring together data and operations to create a roadmap that shows what to do and how to do it right from the start. Artificial intelligence takes the reins of business intelligence and applies simulated actions to scenarios to create the steps necessary to avoid failure and achieve success.
Provides information for real-time and long-term business operations. Decision makers can view both real-time and forecast data at the same time to make decisions that support sustainable growth and success. This streamlines decision making by providing specific recommendations.
Reduce the time you think and the time you run. Immediate turnarounds for data analysis and outcome prediction allow teams to spend less time finding problems and more time designing the perfect solution. Artificial intelligence is a better way than a team of data engineers to curate and process data in a fraction of the time.
Reduces human error and prejudice. Through more sophisticated algorithms and machine learning processes, predictive analytics provides descriptive analytics, predictive analytics, and even more comprehensive and accurate forms of data aggregation and analysis than individuals.